
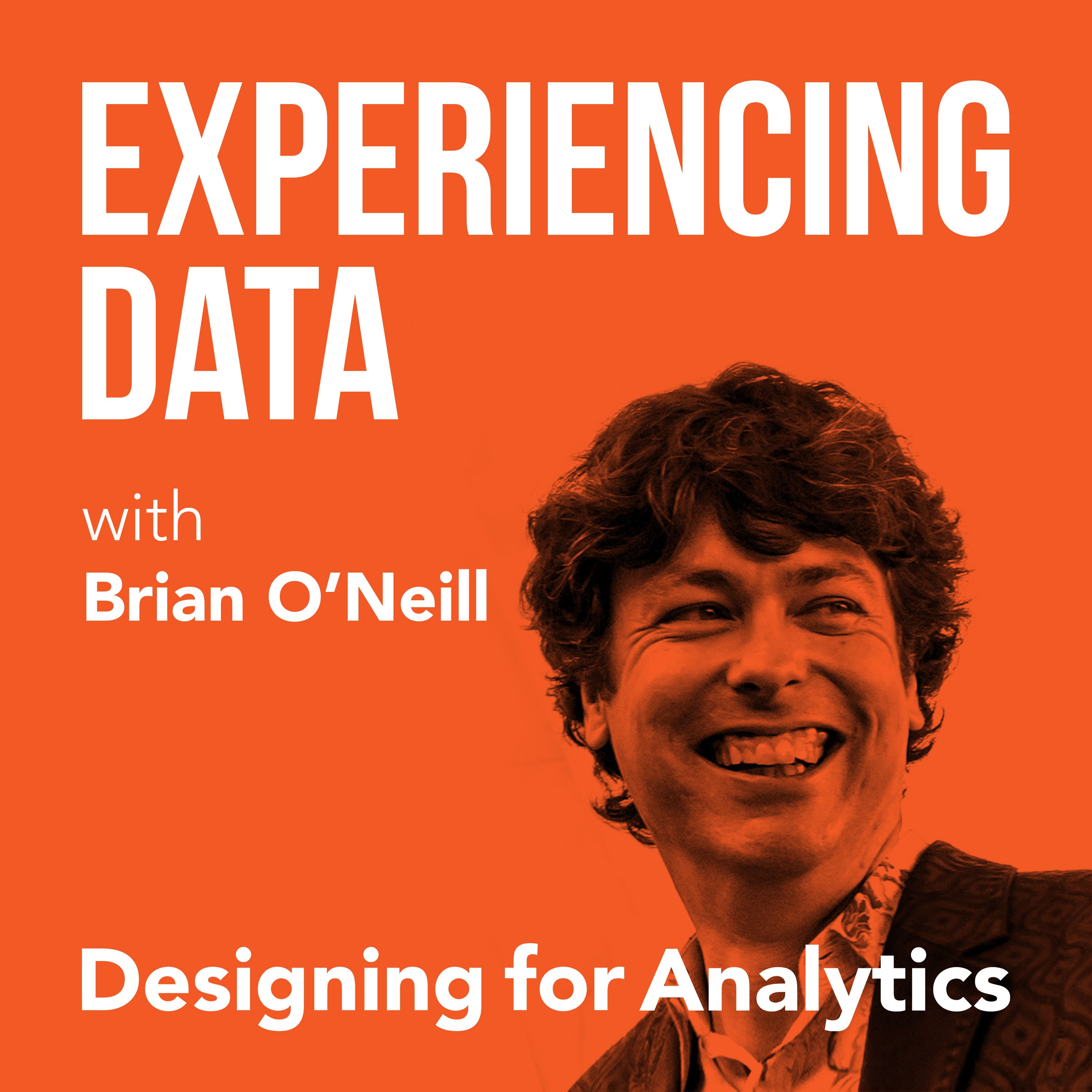
107.9K
Downloads
142
Episodes
If you’re a leader tasked with generating business and org. value through ML/AI and analytics, you’ve probably struggled with low user adoption. Making the tech gets easier, but getting users to use, and buyers to buy, remains difficult—but you’ve heard a ”data product” approach can help. Can it? My name is Brian T. O’Neill, and on Experiencing Data—one of the top 2% of podcasts in the world—I offer you a consulting designer’s perspective on why creating ML and analytics outputs isn’t enough to create business and UX outcomes. How can UX design and product management help you create innovative ML/AI and analytical data products? What exactly are data products—and how can data product management help you increase user adoption of ML/analytics—so that stakeholders can finally see the business value of your data? Every 2 weeks, I answer these questions via solo episodes and interviews with innovative chief data officers, data product management leaders, and top UX professionals. Hashtag: #ExperiencingData. PODCAST HOMEPAGE: Get 1-page summaries, text transcripts, and join my Insights mailing list: https://designingforanalytics.com/ed ABOUT THE HOST, BRIAN T. O’NEILL: https://designingforanalytics.com/bio/
Episodes
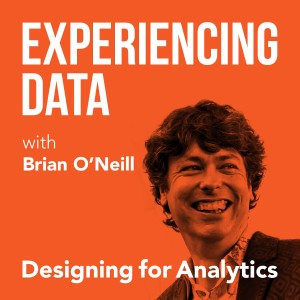
Tuesday Apr 16, 2024
Tuesday Apr 16, 2024
In this week's episode of Experiencing Data, I'm joined by Duncan Milne, a Director, Data Investment & Product Management at the Royal Bank of Canada (RBC). Today, Duncan (who is also a member of the DPLC) gives a preview of his upcoming webinar on April 24, 2024 entitled, “Is that Data Product Worth Building? Estimating Economic Value…Before You Build It!” Duncan shares his experience of implementing a product mindset within RBC's Chief Data Office, and he explains some of the challenges, successes, and insights gained along the way. He emphasizes the critical role of understanding user needs and evaluating the economic impact of data products—before they are built. Duncan was gracious to let us peek inside and see a transformation that is currently in progress and I’m excited to check out his webinar this month!
Highlights/ Skip to:
- I introduce Duncan Milne from RBC (00:00)
- Duncan outlines the Chief Data Office's function at RBC (01:01)
- We discuss data products and how they are used to improve business process (04:05)
- The genesis behind RBC's move towards a product-centric approach in handling data, highlighting initial challenges and strategies for fostering a product mindset (07:26)
- Duncan discusses developing a framework to guide the lifecycle of data products at RBC (09:29)
- Duncan addresses initial resistance and adaptation strategies for engaging teams in a new product-centric methodology (12:04)
- The scaling challenges of applying a product mindset across a large organization like RBC (22:02)
- Insights into the framework for evaluating and prioritizing data product ideas based on their desirability, usability, feasibility, and viability. (26:30)
- Measuring success and value in data product management (30:45)
- Duncan explores process mapping challenges in banking (34:13)
- Duncan shares creating specialized training for data product management at RBC (36:39)
- Duncan offers advice and closing thoughts on data product management (41:38)
Quotes from Today’s Episode
- “We think about data products as anything that solves a problem using data... it's helping someone do something they already do or want to do faster and better using data." - Duncan Milne (04:29)
- “The transition to data product management involves overcoming initial resistance by demonstrating the tangible value of this approach." - Duncan Milne (08:38)
- "You have to want to show up and do this kind of work [adopting a product mindset in data product management]…even if you do a product the right way, it doesn’t always work, right? The thing you make may not be desirable, it may not be as usable as it needs to be. It can be technically right and still fail. It’s not a guarantee, it’s just a better way of working.” - Brian T. O’Neill (15:03)
- “[Product management]... it's like baking versus cooking. Baking is a science... cooking is much more flexible. It’s about... did we produce a benefit for users? Did we produce an economic benefit? ...It’s a multivariate problem... a lot of it is experimentation and figuring out what works." - Brian T. O'Neill (23:03)
- "The easy thing to measure [in product management] is did you follow the process or not? That is not the point of product management at all. It's about delivering benefits to the stakeholders and to the customer." - Brian O'Neill (25:16)
- “Data product is not something that is set in stone... You can leverage learnings from a more traditional product approach, but don’t be afraid to improvise." - Duncan Milne (41:38)
- “Data products are fundamentally different from digital products, so even the traditional approach to product management in that space doesn’t necessarily work within the data products construct.” - Duncan Milne (41:55)
- “There is no textbook for data product management; the field is still being developed…don’t be afraid to create your own answer if what exists out there doesn’t necessarily work within your context.”- Duncan Milne (42:17)
Links
- Duncan’s Linkedin: https://www.linkedin.com/in/duncanwmilne/?originalSubdomain=ca
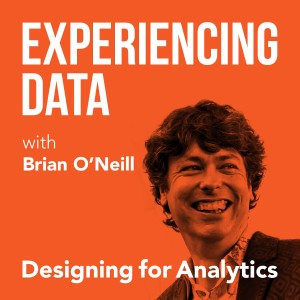
Tuesday Apr 02, 2024
Tuesday Apr 02, 2024
This week on Experiencing Data, I chat with a new kindred spirit! Recently, I connected with Thabata Romanowski—better known as "T from Data Rocks NZ"—to discuss her experience applying UX design principles to modern analytical data products and dashboards. T walks us through her experience working as a data analyst in the mining sector, sharing the journey of how these experiences laid the foundation for her transition to data visualization. Now, she specializes in transforming complex, industry-specific data sets into intuitive, user-friendly visual representations, and addresses the challenges faced by the analytics teams she supports through her design business. T and I tackle common misconceptions about design in the analytics field, discuss how we communicate and educate non-designers on applying UX design principles to their dashboard and application design work, and address the problem with "pretty charts." We also explore some of the core ideas in T's Design Manifesto, including principles like being purposeful, context-sensitive, collaborative, and humanistic—all aimed at increasing user adoption and business value by improving UX.
Highlights/ Skip to:
- I welcome T from Data Rocks NZ onto the show (00:00)
- T's transition from mining to leading an information design and data visualization consultancy. (01:43)
- T discusses the critical role of clear communication in data design solutions. (03:39)
- We address the misconceptions around the role of design in data analytics. (06:54)
- T explains the importance of journey mapping in understanding users' needs. (15:25)
- We discuss the challenges of accurately capturing end-user needs. (19:00)
- T and I discuss the importance of talking directly to end-users when developing data products. (25:56)
- T shares her 'I like, I wish, I wonder' method for eliciting genuine user feedback. (33:03)
- T discusses her Data Design Manifesto for creating purposeful, context-aware, collaborative, and human-centered design principles in data. (36:37)
- We wrap up the conversation and share ways to connect with T. (40:49)
Quotes from Today’s Episode
- "It's not so much that people…don't know what design is, it's more that they understand it differently from what it can actually do..." - T from Data Rocks NZ (06:59)
- "I think [misconception about design in technology] is rooted mainly in the fact that data has been very tied to IT teams, to technology teams, and they’re not always up to what design actually does.” - T from Data Rocks NZ (07:42)
- “If you strip design of function, it becomes art. So, it’s not art… it’s about being functional and being useful in helping people.” - T from Data Rocks NZ (09:06)
- "It’s not that people don’t know, really, that the word design exists, or that design applies to analytics and whatnot; it’s more that they have this misunderstanding that it’s about making things look a certain way, when in fact... It’s about function. It’s about helping people do stuff better." - T from Data Rocks NZ (09:19)
- “Journey Mapping means that you have to talk to people... Data is an inherently human thing. It is something that we create ourselves. So, it’s biased from the start. You can’t fully remove the human from the data" - T from Data Rocks NZ (15:36)
- “The biggest part of your data product success…happens outside of your technology and outside of your actual analysis. It’s defining who your audience is, what the context of this audience is, and to which purpose do they need that product. - T from Data Rocks NZ (19:08)
- “[In UX research], a tight, empowered product team needs regular exposure to end customers; there’s nothing that can replace that." - Brian O'Neill (25:58)
- “You have two sides [end-users and data team] that are frustrated with the same thing. The side who asked wasn’t really sure what to ask. And then the data team gets frustrated because the users don’t know what they want…Nobody really understood what the problem is. There’s a lot of assumptions happening there. And this is one of the hardest things to let go.” - T from Data Rocks NZ (29:38)
- “No piece of data product exists in isolation, so understanding what people do with it… is really important.” - T from Data Rocks NZ (38:51)
Links
- Design Matters Newsletter: https://buttondown.email/datarocksnz
- Website: https://www.datarocks.co.nz/
- LinkedIn: https://www.linkedin.com/company/datarocksnz/
- BlueSky: https://bsky.app/profile/datarocksnz.bsky.social
- Mastodon: https://me.dm/@datarocksnz
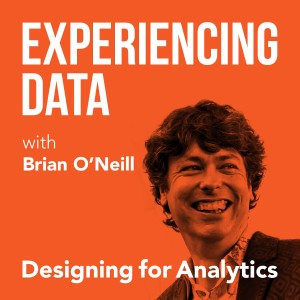
Tuesday Mar 19, 2024
Tuesday Mar 19, 2024
This week on Experiencing Data, something new as promised at the beginning of the year. Today, I’m exploring the world of embedded analytics with Zalak Trivedi from Sigma Computing—and this is also the first approved Promoted Episode on the podcast. In today’s episode, Zalak shares his journey as the product lead for Sigma’s embedded analytics and reporting solution which seeks to accelerate and simplify the deployment of decision support dashboards to their SAAS companies’ customers. Right there, we have the first challenge that Zalak was willing to dig into with me: designing a platform UX when we have multiple stakeholder and user types. In Sigma’s case, this means Sigma’s buyers, the developers that work at these SAAS companies to integrate Sigma into their products, and then the actual customers of these SAAS companies who will be the final end users of the resulting dashboards. also discuss the challenges of creating products that serve both beginners and experts and how AI is being used in the BI industry.
Highlights/ Skip to:
- I introduce Zalak Trivedi from Sigma Computing onto the show (03:15)
- Zalak shares his journey leading the vision for embedded analytics at Sigma and explains what Sigma looks like when implemented into a customer’s SAAS product . (03:54)
- Zalak and I discuss the challenge of integrating Sigma's analytics into various companies' software, since they need to account for a variety of stakeholders. (09:53)
- We explore Sigma's team approach to user experience with product management, design, and technical writing (15:14)
- Zalak reveals how Sigma leverages telemetry to understand and improve user interactions with their products (19:54)
- Zalak outlines why Sigma is a faster and more supportive alternative to building your own analytics (27:21)
- We cover data monetization, specifically looking at how SAAS companies can monetize analytics and insights (32:05)
- Zalak highlights how Sigma is integratingAI into their BI solution (36:15)
- Zalak share his customers' current pain points and interests (40:25)
- We wrap up with final thoughts and ways to connect with Zalak and learn more about Sigma (49:41)
Quotes from Today’s Episode
- "Something I’m really excited about personally that we are working on is [moving] beyond analytics to help customers build entire data applications within Sigma. This is something we are really excited about as a company, and marching towards [achieving] this year." - Zalak Trivedi (04:04)
- “The whole point of an embedded analytics application is that it should look and feel exactly like the application it’s embedded in, and the workflow should be seamless.” - Zalak Trivedi (09:29)
- “We [at Sigma] had to switch the way that we were thinking about personas. It was not just about the analysts or the data teams; it was more about how do we give the right tools to the [SAAS] product managers and developers to embed Sigma into their product.” - Zalak Trivedi (11:30)
- “You can’t not have a design, and you can’t not have a user experience. There’s always an experience with every tool, solution, product that we use, whether it emerged organically as a byproduct, or it was intentionally created through knowledge data... it was intentional” - Brian O’Neill (14:52)
- “If we find that [in] certain user experiences,people are tripping up, and they’re not able to complete an entire workflow, we flag that, and then we work with the product managers, or [with] our customers essentially, and figure out how we can actually simplify these experiences.” - Zalak Trivedi (20:54)
- “We were able to convince many small to medium businesses and startups to sign up with Sigma. The success they experienced after embedding Sigma was tremendous. Many of our customers managed to monetize their existing data within weeks, or at most, a couple of months, with lean development teams of two to three developers and a few business-side personnel, generating seven-figure income streams from that.” - Zalak Trivedi (32:05)
- “At Sigma, our stance is, let’s not just add AI for the sake of adding AI. Let’s really identify [where] in the entire user journey does the intelligence really lie, and where are the different friction points, and let’s enhance those experiences.” - Zalak Trivedi (37:38)
- “Every time [we at Sigma Computing] think about a new feature or functionality, we have to ensure it works for both the first-degree persona and the second-degree persona, and consider how it will be viewed by these different personas, because that is not the primary persona for which the foundation of the product was built." - Zalak Trivedi (48:08)
Links
Sigma Computing: https://sigmacomputing.com
Email: zalak@sigmacomputing.com
LinkedIn: https://www.linkedin.com/in/trivedizalak/
Sigma Computing Embedded: https://sigmacomputing.com/embedded
About Promoted Episodes on Experiencing Data: https://designingforanalytics.com/promoted
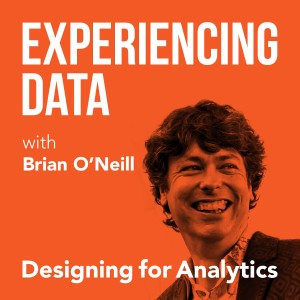
Tuesday Mar 05, 2024
Tuesday Mar 05, 2024
In this episode of Experiencing Data, I speak with Ellen Chisa, Partner at BoldStart Ventures, about what she’s seeing in the venture capital space around AI-driven products and companies—particularly with all the new GenAI capabilities that have emerged in the last year. Ellen and I first met when we were both engaged in travel tech startups in Boston over a decade ago, so it was great to get her current perspective being on the “other side” of products and companies working as a VC. Ellen draws on her experience in product management and design to discuss how AI could democratize software creation and streamline backend coding, design integration, and analytics. We also delve into her work at Dark and the future prospects for developer tools and SaaS platforms. Given Ellen’s background in product management, human-centered design, and now VC, I thought she would have a lot to share—and she did!
Highlights/ Skip to:
- I introduce the show and my guest, Ellen Chisa (00:00)
- Ellen discusses her transition from product and design to venture capital with BoldStart Ventures. (01:15)
- Ellen notes a shift from initial AI prototypes to more refined products, focusing on building and testing with minimal data. (03:22)
- Ellen mentions BoldStart Ventures' focus on early-stage companies providing developer and data tooling for businesses. (07:00)
- Ellen discusses what she learned from her time at Dark and Lola about narrowing target user groups for technology products (11:54)
- Ellen's Insights into the importance of user experience is in product design and the process venture capitalists endure to make sure it meets user needs (15:50)
- Ellen gives us her take on the impact of AI on creating new opportunities for data tools and engineering solutions, (20:00)
- Ellen and I explore the future of user interfaces, and how AI tools could enhance UI/UX for end users. (25:28)
- Closing remarks and the best way to find Ellen on online (32:07)
Quotes from Today’s Episode
- “It's a really interesting time in the venture market because on top of the Gen AI wave, we obviously had the macroeconomic shift. And so we've seen a lot of people are saying the companies that come out now are going to be great companies because they're a little bit more capital-constrained from the beginning, typically, and they'll grow more thoughtfully and really be thinking about how do they build an efficient business.”- Ellen Chisa (03: 22)
- “We have this big technological shift around AI-enabled companies, and I think one of the things I’ve seen is, if you think back to a year ago, we saw a lot of early prototyping, and so there were like a couple of use cases that came up again and again.”-Ellen Chisa (3:42)
- “I don't think I've heard many pitches from founders who consider themselves data scientists first. We definitely get some from ML engineers and people who think about data architecture, for sure..”- Ellen Chisa (05:06)
- “I still prefer GUI interfaces to voice or text usually, but I think that might be an uncanny valley sort of thing where if you think of people who didn’t have technology growing up, they’re more comfortable with the more human interaction, and then you get, like, a chunk of people who are digital natives who prefer it.”- Ellen Chisa (24:51)
- [Citing some excellent Boston-area restaurants!] “The Arc browser just shipped a bunch of new functionality, where instead of opening a bunch of tabs, you can say, “Open the recipe pages for Oleana and Sarma,” and it just opens both of them, and so it’s like multiple search queries at once.” - Ellen Chisa (27:22)
- “The AI wave of technology biases towards people who already have products [in the market] and have existing datasets, and so I think everyone [at tech companies] is getting this big, top-down mandate from their executive team, like, ‘Oh, hey, you have to do something with AI now.’”- Ellen Chisa (28:37)
- “I think it’s hard to really grasp what an LLM is until you do a fair amount of experimentation on your own. The experience of asking ChatGPT a simple search question compared to the experience of trying to train it to do something specific for you are quite different experiences. Even beyond that, there’s a tool called superwhisper that I like that you can take audio content and end up with transcripts, but you can give it prompts to change your transcripts as you’re going. So, you can record something, and it will give you a different output if you say you’re recording an email compared to [if] you’re recording a journal entry compared to [if] you’re recording the transcript for a podcast.”- Ellen Chisa (30:11)
Links
- Boldstart ventures: https://boldstart.vc/
- LinkedIn: https://www.linkedin.com/in/ellenchisa/
- Personal website: https://ellenchisa.com
- Email: ellen@boldstart.vc
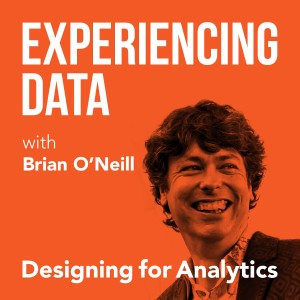
Tuesday Feb 20, 2024
Tuesday Feb 20, 2024
This week, I'm chatting with Karen Meppen, a founding member of the Data Product Leadership Community and a Data Product Architect and Client Services Director at Hakkoda. Today, we're tackling the difficult topic of developing data products in situations where a product-oriented culture and data infrastructures may still be emerging or “at odds” with a human-centered approach. Karen brings extensive experience and a strong belief in how to effectively negotiate the early stages of data maturity. Together we look at the major hurdles that businesses encounter when trying to properly exploit data products, as well as the necessity of leadership support and strategy alignment in these initiatives. Karen's insights offer a roadmap for those seeking to adopt a product and UX-driven methodology when significant tech or cultural hurdles may exist.
Highlights/ Skip to:
- I Introduce Karen Meppen and the challenges of dealing with data products in places where the data and tech aren't quite there yet (00:00)
- Karen shares her thoughts on what it's like working with "immature data" (02:27)
- Karen breaks down what a data product actually is (04:20)
- Karen and I discuss why having executive buy-in is crucial for moving forward with data products (07:48)
- The sometimes fuzzy definition of "data products." (12:09)
- Karen defines “shadow data teams” and explains how they sometimes conflict with tech teams (17:35)
- How Karen identifies the nature of each team to overcome common hurdles of connecting tech teams with business units (18:47)
- How she navigates conversations with tech leaders who think they already understand the requirements of business users (22:48)
- Using design prototypes and design reviews with different teams to make sure everyone is on the same page about UX (24:00)
- Karen shares stories from earlier in her career that led her to embrace human-centered design to ensure data products actually meet user needs (28:29)
- We reflect on our chat about UX, data products, and the “producty” approach to ML and analytics solutions (42:11)
Quotes from Today’s Episode
- "It’s not really fair to get really excited about what we hear about or see on LinkedIn, at conferences, etc. We get excited about the shiny things, and then want to go straight to it when [our] organization [may not be ] ready to do that, for a lot of reasons." - Karen Meppen (03:00)
- "If you do not have support from leadership and this is not something [they are] passionate about, you probably aren’t a great candidate for pursuing data products as a way of working." - Karen Meppen (08:30)
- "Requirements are just friendly lies." - Karen, quoting Brian about how data teams need to interpret stakeholder requests (13:27)
- "The greatest challenge that we have in technology is not technology, it’s the people, and understanding how we’re using the technology to meet our needs." - Karen Meppen (24:04)
- "You can’t automate something that you haven’t defined. For example, if you don’t have clarity on your tagging approach for your PII, or just the nature of all the metadata that you’re capturing for your data assets and what it means or how it’s handled—to make it good, then how could you possibly automate any of this that hasn’t been defined?" - Karen Meppen (38:35)
- "Nothing upsets an end-user more than lifting-and-shifting an existing report with the same problems it had in a new solution that now they’ve never used before." - Karen Meppen (40:13)
- “Early maturity may look different in many ways depending upon the nature of business you’re doing, the structure of your data team, and how it interacts with folks.” (42:46)
Links
- Data Product Leadership Community https://designingforanalytics.com/community/
- Karen Meppen on LinkedIn: https://www.linkedin.com/in/karen--m/
- Hakkōda, Karen's company, for more insights on data products and services:https://hakkoda.io/
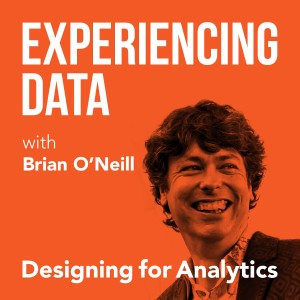
Tuesday Feb 06, 2024
Tuesday Feb 06, 2024
This week I’m chatting with Caroline Zimmerman, Director of Data Products and Strategy at Profusion. Caroline shares her journey through the school of hard knocks that led to her discovery that incorporating more extensive UX research into the data product design process improves outcomes. We explore the complicated nature of discovering and building a better design process, how to engage end users so they actually make time for research, and why understanding how to navigate interdepartmental politics is necessary in the world of data and product design. Caroline reveals the pivotal moment that changed her approach to data product design, as well as her learnings from evolving data products with the users as their needs and business strategies change. Lastly, Caroline and I explore what the future of data product leadership looks like and Caroline shares why there's never been a better time to work in data.
Highlights/ Skip to:
- Intros and Caroline describes how she learned crucial lessons on building data products the hard way (00:36)
- The fundamental moment that helped Caroline to realize that she needed to find a different way to uncover user needs (03:51)
- How working with great UX researchers influenced Caroline’s approach to building data products (08:31)
- Why Caroline feels that exploring the ‘why’ is foundational to designing a data product that gets adopted (10:25)
- Caroline’s experience building a data model for a client and what she learned from that experience when the client’s business model changed (14:34)
- How Caroline addresses the challenge of end users not making time for user research (18:00)
- A high-level overview of the UX research process when Caroline’s team starts working with a new client (22:28)
- The biggest challenges that Caroline faces as a Director of Data Products, and why data products require the ability to navigate company politics and interests (29:58)
- Caroline describes the nuances of working with different stakeholder personas (35:15)
- Why data teams need to embrace a more human-led approach to designing data products and focus less on metrics and the technical aspects (38:10)
- Caroline’s closing thoughts on what she’d like to share with other data leaders and how you can connect with her (40:48)
Quotes from Today’s Episode
- “When I was first starting out, I thought that you could essentially take notes on what someone was asking for, go off and build it to their exact specs, and be successful. And it turns out that you can build something to exact specs and suffer from poor adoption and just not be solving problems because I did it as a wish fulfillment, laundry-list exercise rather than really thinking through user needs.” — Caroline Zimmerman (01:11)
- “People want a thing. They’re paying for a thing, right? And so, just really having that reflex to try to gently come back to that why and spending sufficient time exploring it before going into solution build, even when people are under a lot of deadline pressure and are paying you to deliver a thing [is the most important element of designing a data product].” – Caroline Zimmerman (11:53)
- “A data product evolves because user needs change, business models change, and business priorities change, and we need to evolve with it. It’s not like you got it right once, and then you’re good for life. At all.” – Caroline Zimmerman (17:48)
- “I continue to have lots to learn about stakeholder management and understanding the interplay between what the organization needs to be successful, but also, organizations are made up of people with personal interests, and you need to understand both.” – Caroline Zimmerman (30:18)
- “Data products are built in a political context. And just being aware of that context is important.” – Caroline Zimmerman (32:33)
- “I think that data, maybe more than any other function, is transversal. I think data brings up politics because, especially with larger organizations, there are those departmental and team silos. And the whole thing about data is it cuts through those because it touches all the different teams. It touches all the different processes. And so in order to build great data products, you have to be navigating that political context to understand how to get things done transversely in organizations where most stuff gets done vertically.” – Caroline Zimmerman (34:37)
- “Data leadership positions are data product expertise roles. And I think that often it’s been more technical people that have advanced into those roles. If you follow the LinkedIn-verse in data, it’s very much on every data leader’s mind at the moment: how do you articulate benefits to your CEO and your board and try to do that before it’s too late? So, I’d say that’s really the main thing and that there’s just never been a better time to be a data product person.” – Caroline Zimmerman (37:16)
Links
- Profusion: https://profusion.com/
- Caroline Zimmerman LinkedIn: https://www.linkedin.com/in/caroline-zimmerman-4a531640/
- Nick Zervoudis LinkedIn: https://www.linkedin.com/in/nzervoudis/
- Email: mailto:carolinez@profusion.com
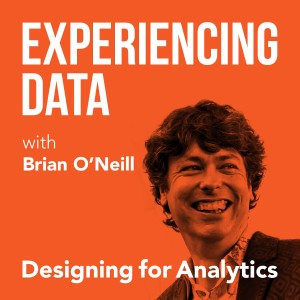
Tuesday Jan 23, 2024
Tuesday Jan 23, 2024
This week, I’m chatting with Steve Portigal, who is the Principal of Portigal Consulting and the Author of Interviewing Users. We discuss the changes that prompted him to release a second version of his book 10 years after its initial release, and dive into the best practices that any team can implement to start unlocking the value of data product UX research. Steve explains that the key to making time for user research is knowing what business value you’re after, not simply having a list of research questions. We then role-play through some in-depth examples of real-life experiences we’ve seen from both end users and leadership when it comes to implementing a user research strategy. Thhroughout our conversation, we come back to the idea that even taking imperfect action towards doing user research can lead to increased data product adoption and business value.
Highlights/ Skip to:
- I introduce Steve Portigal, Principal of Portigal Consulting and Author of Interviewing Users (00:38)
- What changes caused Steve to release a second edition of his book (00:58)
- Steve and I discuss the importance of understanding how to conduct effective user research (03:44)
- Steve explains why it’s crucial to understand that the business challenge and the research questions are two different things (08:16)
- Brian and Steve role-play a common scenario that comes up in user research, and Steve explains an optimal workflow for user research (11:50)
- The importance of provocation in performing user research (21:02)
- How Steve would handle a situation where a member of leadership is preventing research being done with end users (24:23)
- Why a consultative approach is valuable when getting buy-in for conducting user research (35:04)
- Steve shares some of the major benefits of taking imperfect action towards starting user research (36:59)
- The impact and value even easy wins in user research can have (42:54)
- Steve describes the exploratory nature of user research and how to maximize the chance of finding the most valuable insights (46:57)
- Where you can connect with Steve and get a copy of v2 of his book, Interviewing Users (49:35)
Quotes from Today’s Episode
- “If you don’t know what you’re doing, and you don’t know what you should be investing effort-wise, that’s the inexperience in the approach. If you don’t know how to plan, what should we be trying to solve in this research? What are we trying to learn? What are we going to do with it in the organization? Who should we be talking to? How do we find them? What do we ask them? And then a really good one: how do we make sense of that information so that it has impact that we can take away?” — Steve Portigal (07:15)
- “What do people get [from user research]? I think the chance for a team to align around something that comes in from the outside.” – Steve Portigal (41:36)
- On the impact user research can have if teams embrace it: “They had a product that did a thing that no one [understood], and they had to change the product, but also change how they talked about it, change how they built it, and change how they packaged it. And that was a really dramatic turnaround. And it came out of our research, but [mostly] because they really leaned into making use of this stuff.” – Steve Portigal (42:35)
- "If we knew all the questions to ask, we would just write a survey, right? It’s a lower time commitment from the participant to do that. But we’re trying to get at what we don’t know that we don’t know. For some of us, that’s fun!" – Steve Portigal (48:36)
Links
- Interviewing Users (use code DATA20 to get 20% off the list price): https://rosenfeldmedia.com/books/interviewing-users-second-edition/
- Personal website: https://portigal.com
- Publisher website: https://rosenfeldmedia.com
- LinkedIn: https://www.linkedin.com/in/steveportigal/
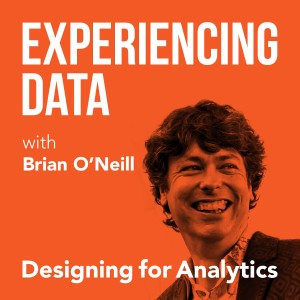
Tuesday Jan 09, 2024
134 - What Sanjeev Mohan Learned Co-Authoring “Data Products for Dummies”
Tuesday Jan 09, 2024
Tuesday Jan 09, 2024
In this episode, I’m chatting with former Gartner analyst Sanjeev Mohan who is the Co-Author of Data Products for Dummies. Throughout our conversation, Sanjeev shares his expertise on the evolution of data products, and what he’s seen as a result of implementing practices that prioritize solving for use cases and business value. Sanjeev also shares a new approach of structuring organizations to best implement ownership and accountability of data product outcomes. Sanjeev and I also explore the common challenges of product adoption and who is responsible for user experience. I purposefully had Sanjeev on the show because I think we have pretty different perspectives from which we see the data product space.
Highlights/ Skip to:
- I introduce Sanjeev Mohan, co-author of Data Products for Dummies (00:39)
- Sanjeev expands more on the concept of writing a “for Dummies” book (00:53)
- Sanjeev shares his definition of a data product, including both a technical and a business definition (01:59)
- Why Sanjeev believes organizational changes and accountability are the keys to preventing the acceleration of shipping data products with little to no tangible value (05:45)
- How Sanjeev recommends getting buy-in for data product ownership from other departments in an organization (11:05)
- Sanjeev and I explore adoption challenges and the topic of user experience (13:23)
- Sanjeev explains what role is responsible for user experience and design (19:03)
- Who should be responsible for defining the metrics that determine business value (28:58)
- Sanjeev shares some case studies of companies who have adopted this approach to data products and their outcomes (30:29)
- Where companies are finding data product managers currently (34:19)
- Sanjeev expands on his perspective regarding the importance of prioritizing business value and use cases (40:52)
- Where listeners can get Data Products for Dummies, and learn more about Sanjeev’s work (44:33)
Quotes from Today’s Episode
- “You may slap a label of data product on existing artifact; it does not make it a data product because there’s no sense of accountability. In a data product, because they are following product management best practices, there must be a data product owner or a data product manager. There’s a single person [responsible for the result]. — Sanjeev Mohan (09:31)
- “I haven’t even mentioned the word data mesh because data mesh and data products, they don’t always have to go hand-in-hand. I can build data products, but I don’t need to go into the—do all of data mesh principles.” – Sanjeev Mohan (26:45)
- “We need to have the right organization, we need to have a set of processes, and then we need a simplified technology which is standardized across different teams. So, this way, we have the benefit of reusing the same technology. Maybe it is Snowflake for storage, DBT for modeling, and so on. And the idea is that different teams should have the ability to bring their own analytical engine.” – Sanjeev Mohan (27:58)
- “Generative AI, right now as we are recording, is still in a prototyping phase. Maybe in 2024, it’ll go heavy-duty production. We are not in prototyping phase for data products for a lot of companies. They’ve already been experimenting for a year or two, and now they’re actually using them in production. So, we’ve crossed that tipping point for data products.” – Sanjeev Mohan (33:15)
- “Low adoption is a problem that’s not just limited to data products. How long have we had data catalogs, but they have low adoption. So, it’s a common problem.” – Sanjeev Mohan (39:10)
- “That emphasis on technology first is a wrong approach. I tell people that I’m sorry to burst your bubble, but there are no technology projects, there are only business projects. Technology is an enabler. You don’t do technology for the sake of technology; you have to serve a business cause, so let’s start with that and keep that front and center.” – Sanjeev Mohan (43:03)
Links
- Data Products for Dummies: https://www.dataops.live/dataproductsfordummies
- “What Exactly is A Data Product” article: https://medium.com/data-mesh-learning/what-exactly-is-a-data-product-7f6935a17912
- It Depends: https://www.youtube.com/@SanjeevMohan
- Chief Data Analytics and Product Officer of Equifax: https://www.youtube.com/watch?v=kFY7WGc-jFM
- SanjMo Consulting: https://www.sanjmo.com/
- dataops.live: https://dataops.live
- dataops.live/dataproductsfordummies: https://dataops.live/dataproductsfordummies
- LinkedIn: https://www.linkedin.com/in/sanjmo/
- Medium articles: https://sanjmo.medium.com
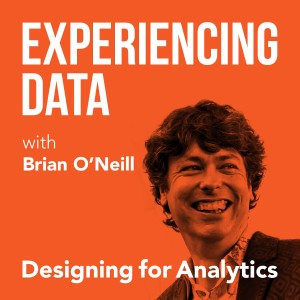
Tuesday Dec 26, 2023
133 - New Experiencing Data Interviews Coming in January 2024
Tuesday Dec 26, 2023
Tuesday Dec 26, 2023
Today I am sharing some highlights for 2023 from the podcast, and also letting you all know I’ll be taking a break from the podcast for the rest of December, but I’ll be back with a new episode on January 9th, 2024. I’ve also got two links to share with you—details inside!
Transcript
Greetings everyone - I’m taking a little break from Experiencing Data over December of 2023, but I’ll be back in January with more interviews and insights on leveraging UX design and product management to create indispensable data products, machine learning apps, and decision support tools.
Experiencing Data turned this year five years old back in November, with over 130 episodes to date! I still can’t believe it’s been going that long and how far we’ve come.
Some highlights for me in 2023 included launching the Data Product Leadership Community, finding out that the show is now in the top 2% of all podcasts worldwide according to ListenNotes, and most of all, hearing from you that the podcast, and my writing, and the guests that I have brought on are having an impact on your work, your careers, and hopefully the lives of your customers, users, and stakeholders as well!
So, for now, I’ve got just two links for you:
If you’re wondering how to either:
- support the show yourself with a really fast review on Apple Podcasts,
- to record a quick audio question for me to answer on the show,
- or if you want to join my free Insights mailing lists where I share my bi-weekly ideas and thoughts and 1-page episode summaries of all the show drops that I put out here on Experiencing Data.
…just head over to designingforanalytics.com/podcast and you’ll get links to all those things there.
And secondly, if you need help increasing customer adoption, delight, the business value, or the usability of your analytics and machine learning applications in 2024, I invite you to set up a free discovery call with me 1 on 1.
You bring the questions, I’ll bring my ears, and by the end of the call, I’ll give you my best advice on how to move forward with your situation – whether it’s working with me or not. To schedule one of those free discovery calls, visit designingforanalytics.com/go
And finally, there will be some news coming out next year with the show, as well as my business, so I hope you’ll hop on the mailing list and stay tuned, that’s probably the best place to do that. And if you celebrate holidays in December and January, I hope they’re safe, enjoyable, and rejuvenating. Until 2024, stay tuned right here - and in the words of the great Arnold Schwarzenegger, I’ll be back.
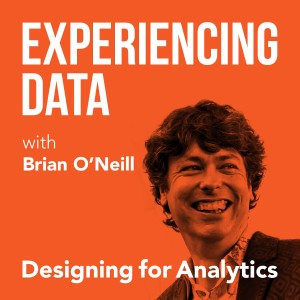
Tuesday Dec 12, 2023
Tuesday Dec 12, 2023
In this conversation with Klara Lindner, Service Designer at diconium data, we explore how behavioral science and UX can be used to increase adoption of data products. Klara describes how she went from having a highly technical career as an electrical engineer and being the founder of a solar startup to her current role in service design for data products. Klara shares powerful insights into the value of user research and human-centered design, including one which stopped me in my tracks during this episode: how the people making data products and evangelizing data-driven decision making aren’t actually following their own advice when it comes to designing their data products. Klara and I also explore some easy user research techniques that data professionals can use, and discuss who should ultimately be responsible for user adoption of data products. Lastly, Klara gives us a peek at her upcoming December 19th, 2023 webinar with the The Data Product Leadership Community (DPLC) where she will be going deeper on two frameworks from psychology and behavioral science that teams can use to increase adoption of data products. Klara is also a founding member of the DPLC and was one of—if not the very first—design/UX professionals to join.
Highlights/ Skip to:
- I introduce Klara, and she explains the role of Service Design to our audience (00:49)
- Klara explains how she realized she’s been doing design work longer than she thought by reflecting on the company she founded, Mobisol (02:09)
- How Klara balances the desire to design great dashboards with the mission of helping end users (06:15)
- Klara describes the psychology behind user research and her upcoming talk on December 19th at The Data Product Leadership Community (08:32)
- What data product teams can do as a starting point to begin implementing user research principles (10:52)
- Klara gives a powerful example of the type of insight and value even basic user research can provide (12:49)
- Klara and I discuss a key revelation when it comes to designing data products for users, which is the irony that even developers use intuition as well as quantitative data when building (16:43)
- What adjustments Klara had to make in her thinking when moving from a highly technical background to doing human-centered design (21:08)
- Klara describes the two frameworks for driving adoption that she’ll be sharing in her talk at the DPLC on December 19th (24:23)
- An example of how understanding and addressing adoption blockers is important for product and design teams (30:44)
- How Klara has seen her teams adopt a new way of thinking about product & service design (32:55)
- Klara gives her take on the Jobs to be Done framework, which she will also be sharing in her talk at the DPLC on December 19th (35:26)
- Klara’s advice to teams that are looking to build products around generative AI (39:28)
- Where listeners can connect with Klara to learn more (41:37)
Links
- diconium data: http://www.diconium.com/
- LinkedIn: https://www.linkedin.com/in/klaralindner/
- Personal Website: https://magic-investigations.com/
- Hear Klara speak on Dec 19, 2023 at 10am ET here: https://designingforanalytics.com/community/