
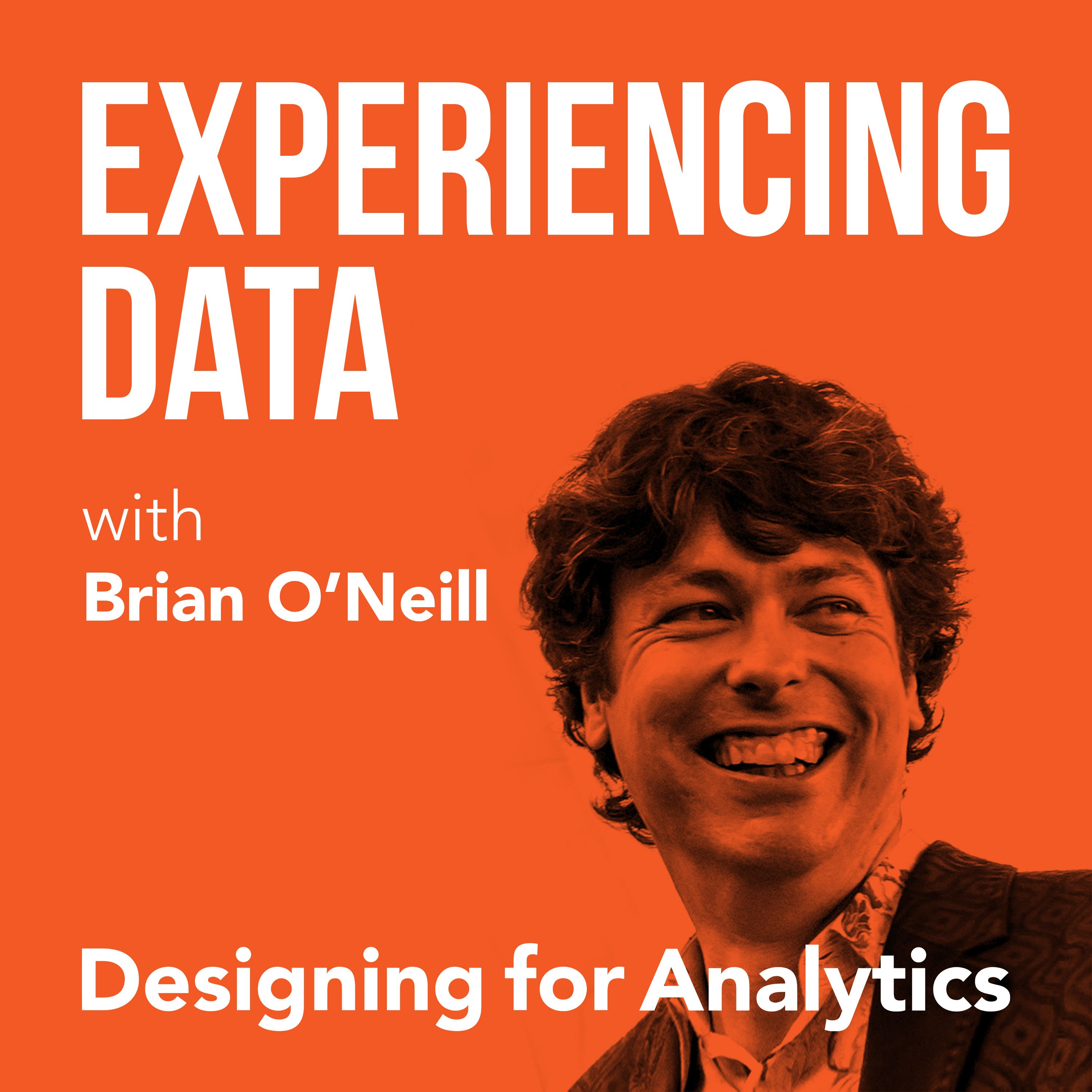
141.5K
Downloads
173
Episodes
Is the value of your enterprise analytics SAAS or AI product not obvious through it’s UI/UX? Got the data and ML models right...but user adoption of your dashboards and UI isn’t what you hoped it would be? While it is easier than ever to create AI and analytics solutions from a technology perspective, do you find as a founder or product leader that getting users to use and buyers to buy seems harder than it should be? If you lead an internal enterprise data team, have you heard that a ”data product” approach can help—but you’re concerned it’s all hype? My name is Brian T. O’Neill, and on Experiencing Data—one of the top 2% of podcasts in the world—I share the stories of leaders who are leveraging product and UX design to make SAAS analytics, AI applications, and internal data products indispensable to their customers. After all, you can’t create business value with data if the humans in the loop can’t or won’t use your solutions. Every 2 weeks, I release interviews with experts and impressive people I’ve met who are doing interesting work at the intersection of enterprise software product management, UX design, AI and analytics—work that you need to hear about and from whom I hope you can borrow strategies. I also occasionally record solo episodes on applying UI/UX design strategies to data products—so you and your team can unlock financial value by making your users’ and customers’ lives better. Hashtag: #ExperiencingData. JOIN MY INSIGHTS LIST FOR 1-PAGE EPISODE SUMMARIES, TRANSCRIPTS, AND FREE UX STRATEGY TIPS https://designingforanalytics.com/ed ABOUT THE HOST, BRIAN T. O’NEILL: https://designingforanalytics.com/bio/
Episodes
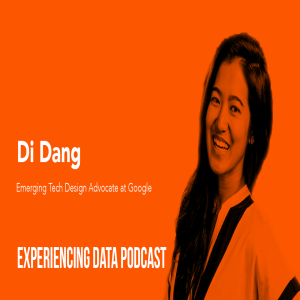
Tuesday Dec 31, 2019
Tuesday Dec 31, 2019
Di Dang is an emerging tech design advocate at Google and helped lead the creation of Google’s People + AI Guidebook. In her role, she works with product design teams, external partners, and end users to support the creation of emerging tech experiences. She also teaches a course on immersive technology at the School of Visual Concepts. Prior to these positions, Di worked as an emerging tech lead and senior UX designer at POP, a UX consultant at Kintsugi Creative Solutions, and a business development manager at AppLift. She earned a bachelor of arts degree in philosophy and religious studies from Stanford University.
Join Brian and Di as they discuss the intersection of design and human-centered AI and:
- Why a data science leader should care about design and integrating designers during a machine-learning project, and the impacts when they do not
- What exactly Di does in her capacity as an emerging tech design advocate at Google and the definition of human-centered AI
- How design helps data science teams save money and time by elucidating the problem space and user needs
- The two key purposes of Google’s People + AI Research (PAIR) team
- What Google’s triptych methodology is and how it helps teams prevent building the wrong solution
- A specific example of how user research and design helped ship a Pixel 2 feature
- How to ensure an AI solution is human-centered when a non-tech company wants to build something but lacks a formal product manager or UX lead/resource
- The original goals behind the creation of Google’s People + AI Guidebook
- The role vocabulary plays in human-centered AI design
Resources and Links
Twitter: @Dqpdang
Quotes from Today’s Episode
“Even within Google, I can’t tell you how many times I have tech leaders, engineers who kind of cock an eyebrow at me and ask, ‘Why would design be involved when it comes to working with machine learning?’” — Di
“Software applications of machine learning is a relatively nascent space and we have a lot to learn from in terms of designing for it. The People + AI Guidebook is a starting point and we want to understand what works, what doesn’t, and what’s missing so that we can continue to build best practices around AI product decisions together.” — Di
“The key value proposition that design brings is we want to work with you to help make sure that when we’re utilizing machine learning, that we’re utilizing it to solve a problem for a user in a way that couldn’t be done through other technologies or through heuristics or rules-based programming—that we’re really using machine learning where it’s most needed.” — Di
“A key piece that I hear again and again from internal Google product teams and external product teams that I work with is that it’s very, very easy for a lot of teams to default to a tech-first kind of mentality. It’s like, ‘Oh, well you know, machine learning, should we ML this?’ That’s a very common problem that we hear. So then, machine learning becomes this hammer for which everything is a nail—but if only a hammer were as easy to construct as a piece of wood and a little metal anvil kind of bit.” — Di
“A lot of folks are still evolving their own mental model around what machine learning is and what it’s good for. But closely in relation—because this is something that I think people don’t talk as much about maybe because it’s less sexy to talk about than machine learning—is that there are often times a lot of organizational or political or cultural uncertainties or confusion around even integrating machine learning.” — Di
“I think there’s a valid promise that there’s a real opportunity with AI. It’s going to change businesses in a significant way and there’s something to that. At the same time, it’s like go purchase some data scientists, throw them in your team, and have them start whacking stuff. And they’re kind of waiting for someone to hand them a good problem to work on and the business doesn’t know and they’re just saying, ‘What is our machine learning strategy?’ And so someone in theory hopefully is hunting for a good problem to solve.” — Brian
“Everyone’s trying to move fast all the time and ship code and a lot of times we focus on the shipping of code and the putting of models into production as our measurement—as opposed to the outcomes that come from putting something into production.” — Brian
“The difference between the good and the great designer is the ability to merge the business objectives with ethically sound user-facing and user-centered principles.” — Brian
No comments yet. Be the first to say something!