
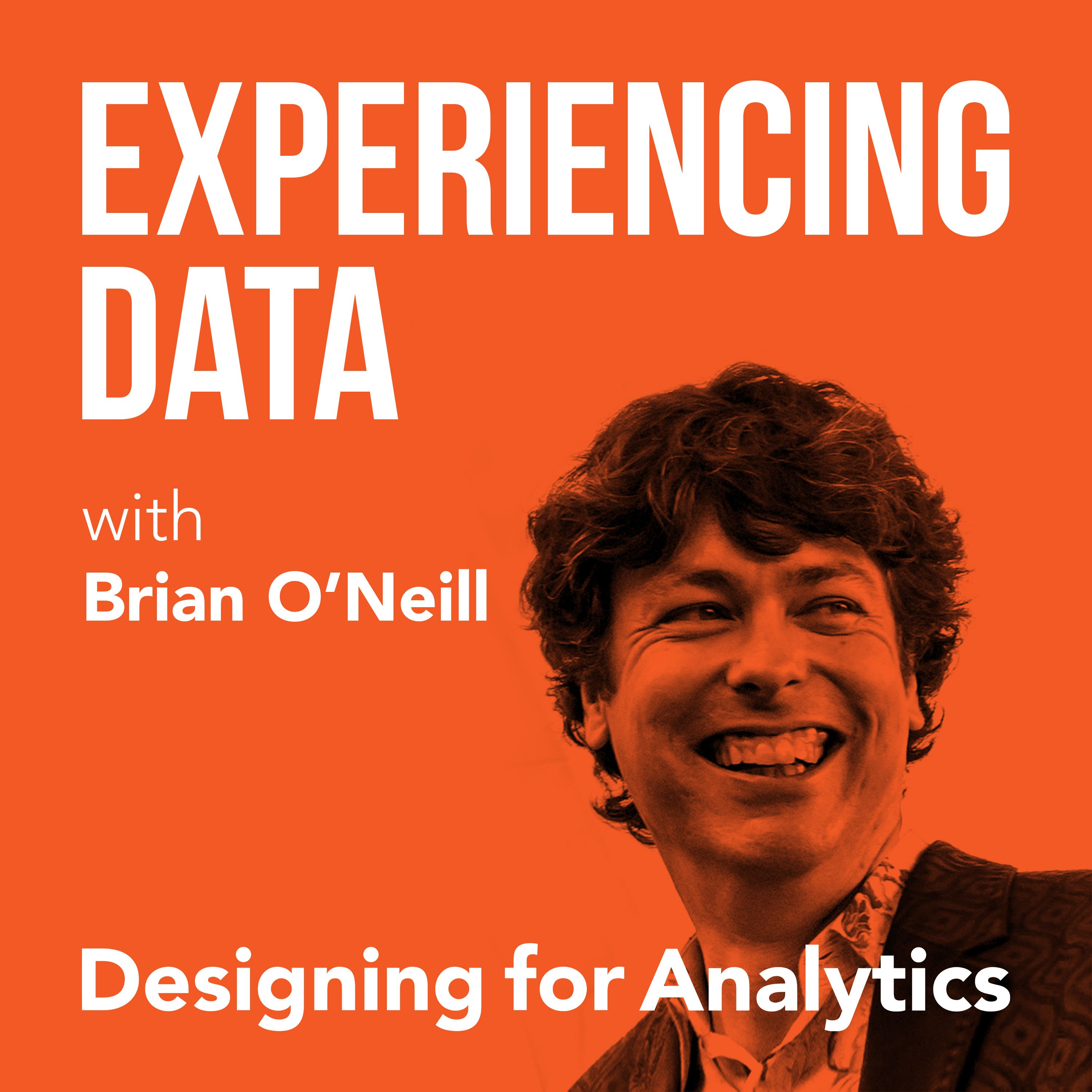
127.8K
Downloads
161
Episodes
If you’re a leader tasked with generating business and org. value through ML/AI and analytics, you’ve probably struggled with low user adoption. Making the tech gets easier, but getting users to use, and buyers to buy, remains difficult—but you’ve heard a ”data product” approach can help. Can it? My name is Brian T. O’Neill, and on Experiencing Data—one of the top 2% of podcasts in the world—I offer you a consulting designer’s perspective on why creating ML and analytics outputs isn’t enough to create business and UX outcomes. How can UX design and product management help you create innovative ML/AI and analytical data products? What exactly are data products—and how can data product management help you increase user adoption of ML/analytics—so that stakeholders can finally see the business value of your data? Every 2 weeks, I answer these questions via solo episodes and interviews with innovative chief data officers, data product management leaders, and top UX professionals. Hashtag: #ExperiencingData. PODCAST HOMEPAGE: Get 1-page summaries, text transcripts, and join my Insights mailing list: https://designingforanalytics.com/ed ABOUT THE HOST, BRIAN T. O’NEILL: https://designingforanalytics.com/bio/
Episodes
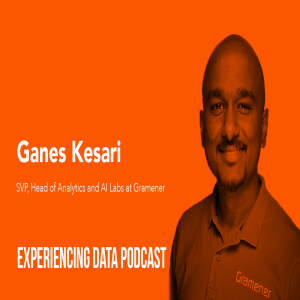
Tuesday Jan 28, 2020
Tuesday Jan 28, 2020
Ganes Kesari is the co-founder and head of analytics and AI labs at Gramener, a software company that helps organizations tell more effective stories with their data through robust visualizations. He’s also an advisor, public speaker, and author who talks about AI in plain English so that a general audience can understand it. Prior to founding Gramener, Ganes worked at companies like Cognizant, Birlasoft, and HCL Technologies serving in various management and analyst roles.
Join Ganes and I as we talk about how design, as a core competency, has enabled Gramener’s analytics and machine learning work to produce better value for clients. We also touched on:
- Why Ganes believes the gap between the business and data analytics organizations is getting smaller
- How AI (and some other buzzwords) are encouraging more and more investments in understanding data
- Ganes’ opinions about the “analytics translator” role
- How companies might think they are unique for not using “traditional agile”—when in fact that’s what everyone is doing
- Ganes’ thoughts on the similarities of use cases across verticals and the rise of verticalized deep data science solutions
- Why Ganes believes organizations are increasingly asking for repeatable data science solutions
- The pivotal role that empathy plays in convincing someone to use your software or data model
- How Ganes’ team approaches client requests for data science projects, the process they follow to identify use cases for AI, and how they use AI to identify the biggest business problem that can be solved
- What Ganes believes practitioners should consider when moving data projects forward at their organizations
Resources and Links
Ganes Kesari on Twitter: @Kesaritweets
Ganes Kesari on LinkedIn: https://www.linkedin.com/in/ganes-kesari/
Quotes from Today’s Episode
“People tend to have some in-house analytics capability. They’re reaching out for design. Then it’s more of where people feel that the adoption hasn’t happened. They have that algorithm but no one understands its use. And then they try to buy some license or some exploratory visualization tools and they try their hand at it and they’ve figured out that it probably needs a lot more than some cute charts or some dashboards. It can’t be an afterthought. That’s when they reach out.” — Ganes
“Now a lot more enquiries, a lot more engagements are happening centrally at the enterprise level where they have realized the need for data science and they want to run it centrally so it’s no longer isolated silos.” — Ganes
“I see that this is a slightly broader movement where people are understanding the value of data and they see that it is something that they can’t avoid or they can’t prioritize it lower anymore.“ — Ganes
“While we have done a few hundred consulting engagements and help with bespoke solutions, there is still an element of commonality. So that’s where we abstracted some of those, the common or technology requirements and common solutions into our platform.” — Ganes
“My general perception is that most data science and analytics firms don’t think about design as a core competency or part of analytics and data science—at least not beyond perhaps data visualization.” —Brian
“I was in a LinkedIn conversation today about this and some comments that Tom Davenport had made on this show a couple of episodes ago. He was talking about how we need this type of role that goes out and understands how data is used and how systems and software are used such that we can better align the solutions with what people are doing. And I was like, ‘amen.’ That’s actually not a new role though; it’s what good designers do!” — Brian
Comments (0)
To leave or reply to comments, please download free Podbean or
No Comments
To leave or reply to comments,
please download free Podbean App.