
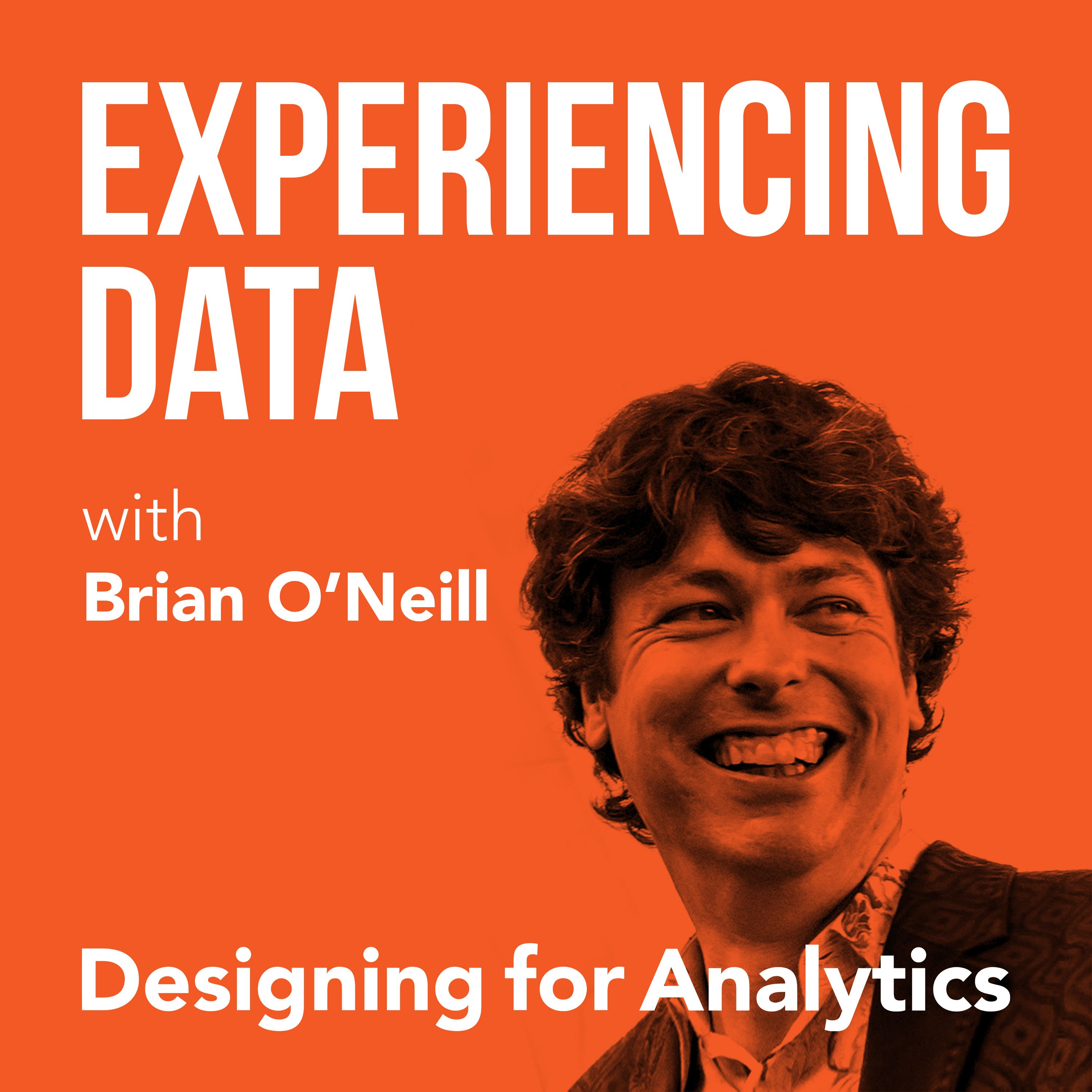
136.1K
Downloads
168
Episodes
Is the value of your enterprise analytics SAAS or AI product not obvious through it’s UI/UX? Got the data and ML models right...but user adoption of your dashboards and UI isn’t what you hoped it would be? While it is easier than ever to create AI and analytics solutions from a technology perspective, do you find as a founder or product leader that getting users to use and buyers to buy seems harder than it should be? If you lead an internal enterprise data team, have you heard that a ”data product” approach can help—but you’re concerned it’s all hype? My name is Brian T. O’Neill, and on Experiencing Data—one of the top 2% of podcasts in the world—I share the stories of leaders who are leveraging product and UX design to make SAAS analytics, AI applications, and internal data products indispensable to their customers. After all, you can’t create business value with data if the humans in the loop can’t or won’t use your solutions. Every 2 weeks, I release interviews with experts and impressive people I’ve met who are doing interesting work at the intersection of enterprise software product management, UX design, AI and analytics—work that you need to hear about and from whom I hope you can borrow strategies. I also occasionally record solo episodes on applying UI/UX design strategies to data products—so you and your team can unlock financial value by making your users’ and customers’ lives better. Hashtag: #ExperiencingData. JOIN MY INSIGHTS LIST FOR 1-PAGE EPISODE SUMMARIES, TRANSCRIPTS, AND FREE UX STRATEGY TIPS https://designingforanalytics.com/ed ABOUT THE HOST, BRIAN T. O’NEILL: https://designingforanalytics.com/bio/
Episodes
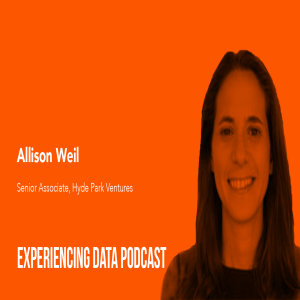
Tuesday Jun 30, 2020
Tuesday Jun 30, 2020
“What happened in Minneapolis and Louisville and Chicago and countlessother cities across the United States is unconscionable (and to be clear, racist). But what makes me the maddest is how easy this problem is to solve, just by the police deciding it’s a thing they want to solve.” - Allison Weil on Medium Before Allison Weil became an investor and Senior Associate at Hyde Park Ventures, she was a co-founder at Flag Analytics, an early intervention system for police departments designed to help identify officers at risk of committing harm. Unfortunately, Flag Analytics—as a business—was set up for failure from the start, regardless of its predictive capability. As Allison explains so candidly and openly in her recent Medium article (thanks Allison!), the company had “poor product-market fit, a poor problem-market fit, and a poor founder-market fit.” The technology was not the problem, and as a result, it did not help them succeed as a business or in producing the desired behavior change because the customers were not ready to act on the insights. Yet, the key takeaways from her team’s research during the design and validation of their product — and the uncomfortable truths they uncovered — are extremely valuable, especially now as we attempt to understand why racial injustice and police brutality continue to persist in law enforcement agencies. As it turns out, simply having the data to support a decision doesn’t mean the decision will be made using the data. This is what Allison found out while in her interactions with several police chiefs and departments, and it’s also what we discussed in this episode. I asked Allison to go deeper into her Medium article, and she agreed. Together, we covered:
- How Allison and a group of researchers tried to streamline the identification of urban police officers at risk of misconduct or harm using machine learning.
- Allison’s experience of trying to build a company and program to solve a critical societal issue, and dealing with police departments that weren’t ready to take action on the analytical insights her product revealed
- How she went about creating a “single pane of glass,” where officers could monitor known problem officers and also discover officers who may be in danger of committing harm.
- The barriers that prevented the project from being a success, from financial ones to a general unwillingness among certain departments to take remedial action against officers despite historical or predicted data
- The key factors and predictors Allison’s team found in the data set of thousands of officers that correlated highly with poor officer behavior in the future—and how it seemed to fall on deaf ears
- How Allison and her team approached the sensitive issue of race in the data, and a [perhaps unexpected] finding they discovered about how prevalent racism seemed to be in departments in general.
- Allison’s experience of conducting “ride-alongs” (qualitative 1x1 research) where she went on patrol with officers to observe their work and how the experience influenced how her team designed the product and influenced her perspective while analyzing the police officer data set.
Resources and Links:
Quotes from Today’s Episode
“The folks at the police departments that we were working with said they were well-intentioned, and said that they wanted to talk through, and fix the problem, but when it came to their actions, it didn't seem like [they were] really willing to make the choices that they needed to make based off of what the data said, and based off of what they knew already.” - Allison “I don't come from a policing background, and neither did any of my co-founders. And that made it really difficult to relate to different officers, and relate to departments. And so the combination of all of those things really didn't set me up for a whole lot of business success in that way.”- Allison “You can take a whole lot of data and do a bunch of analysis, but what I saw was the data didn't show anything that the police department didn't know already. It amplified some of what they knew, but [the problem here] wasn't about the data.” - Allison “It was really frustrating for me, as a founder, sure, because I was putting all this energy into trying to build a software and trying to build a company, but also just frustrating for me as a person and a citizen… you fundamentally want to solve a problem, or help a community solve a problem, and realize that the people at the center of it just aren't ready for it to be solved.” - Allison “...We did have race data, but race was not the primary predictor or reason for [brutality]. It may have been a factor, but it was not that there were racist cops wandering around, using force only against people of particular races. What we found was….” - Allison “The way complaints are filed department to department is really, really different. And so that results in complaints looking really, really different from department to department and counts looking different. But how many are actually reviewed and sustained? And that looks really, really different department to department.” - Allison “...Part of [diversity] is asking the questions you don't know to ask. And that's part of what you get out of having a diverse team— they're going to surface questions that no one else is asking about. And then you can have the discussion about what to do about them.” - Brian
Comments (0)
To leave or reply to comments, please download free Podbean or
No Comments
To leave or reply to comments,
please download free Podbean App.