
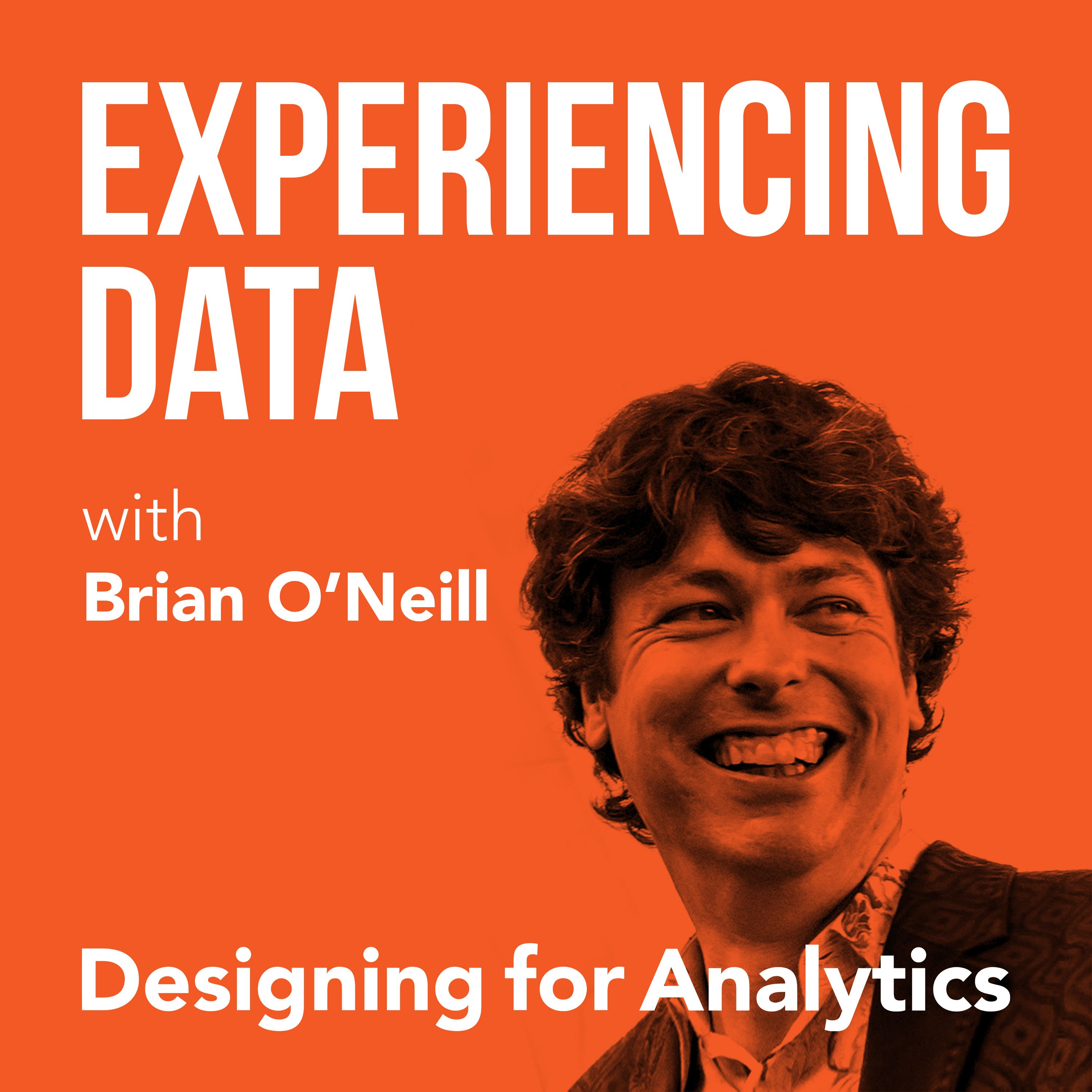
137.1K
Downloads
169
Episodes
Is the value of your enterprise analytics SAAS or AI product not obvious through it’s UI/UX? Got the data and ML models right...but user adoption of your dashboards and UI isn’t what you hoped it would be? While it is easier than ever to create AI and analytics solutions from a technology perspective, do you find as a founder or product leader that getting users to use and buyers to buy seems harder than it should be? If you lead an internal enterprise data team, have you heard that a ”data product” approach can help—but you’re concerned it’s all hype? My name is Brian T. O’Neill, and on Experiencing Data—one of the top 2% of podcasts in the world—I share the stories of leaders who are leveraging product and UX design to make SAAS analytics, AI applications, and internal data products indispensable to their customers. After all, you can’t create business value with data if the humans in the loop can’t or won’t use your solutions. Every 2 weeks, I release interviews with experts and impressive people I’ve met who are doing interesting work at the intersection of enterprise software product management, UX design, AI and analytics—work that you need to hear about and from whom I hope you can borrow strategies. I also occasionally record solo episodes on applying UI/UX design strategies to data products—so you and your team can unlock financial value by making your users’ and customers’ lives better. Hashtag: #ExperiencingData. JOIN MY INSIGHTS LIST FOR 1-PAGE EPISODE SUMMARIES, TRANSCRIPTS, AND FREE UX STRATEGY TIPS https://designingforanalytics.com/ed ABOUT THE HOST, BRIAN T. O’NEILL: https://designingforanalytics.com/bio/
Episodes
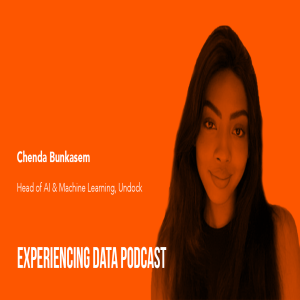
Tuesday Nov 03, 2020
Tuesday Nov 03, 2020
Chenda Bunkasem is head of machine learning at Undock, where she is focusing on using quantitative methods to influence ethical design. In this episode of Experiencing Data, Chenda and I explore her actual methods to designing ethical AI solutions as well as how she works with UX and product teams on ML solutions.
We covered:
- How data teams can actually design ethical ML models, after understanding if ML is the right approach to begin with
- How Chenda aligns her data science work with the desired UX, so that technical choices are always in support of the product and user instead of “what’s cool”
- An overview of Chenda’s role at Undock, where she works very closely with product and marketing teams, advising them on uses for machine learning
- How Chenda’s approaches to using AI may change when there are humans in the loop
- What NASA’s Technology Readiness Level (TRL) evaluation is, and how Chenda uses it in her machine learning work
- What ethical pillars are and how they relate to building AI solutions
- What the Delphi method is and how it relates to creating and user-testing ethical machine learning solutions
Quotes From Today’s Episode
“There's places where machine learning should be used and places where it doesn't necessarily have to be.” - Chenda
“The more interpretability, the better off you always are.” - Chenda
“The most advanced AI doesn't always have to be implemented. People usually skip past this, and they're looking for the best transformer or the most complex neural network. It's not the case. It’s about whether or not the product sticks and the product works alongside the user to aid whatever their endeavor is, or whatever the purpose of that product is. It can be very minimalist in that sense.” - Chenda
“First we bring domain experts together, and then we analyze the use case at hand, and whatever goes in the middle — the meat, between that — is usually decided through many iterations after meetings, and then after going out and doing some sort of user testing, or user research, coming back, etc.” - Chenra, explaining the Delphi method.
“First you're taking answers on someone's ethical pillars or a company's ethical pillars based off of their intuition, and then you're finding how that solution can work in a more engineering or systems-design fashion. “ - Chenda
“I'm kind of very curious about this area of prototyping, and figuring out how fast can we learn something about what the problem space is, and what is needed, prior to doing too much implementation work that we or the business don't want to rewind and throw out.” - Brian
“There are a lot of data projects that get created that end up not getting used at all.”- Brian
Links
Connect with Chenda on LinkedIn
Comments (0)
To leave or reply to comments, please download free Podbean or
No Comments
To leave or reply to comments,
please download free Podbean App.