
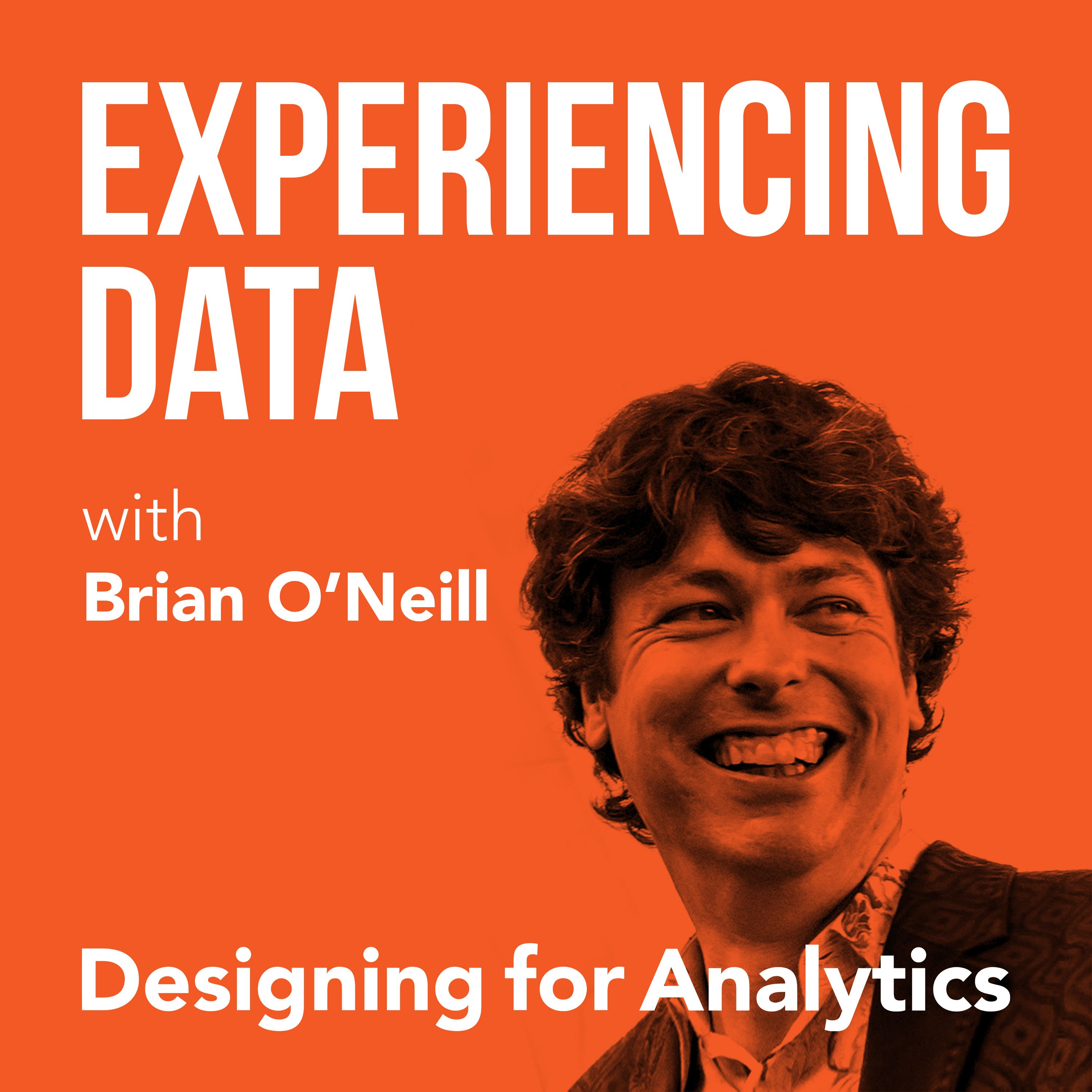
142.2K
Downloads
174
Episodes
Is the value of your enterprise analytics SAAS or AI product not obvious through it’s UI/UX? Got the data and ML models right...but user adoption of your dashboards and UI isn’t what you hoped it would be? While it is easier than ever to create AI and analytics solutions from a technology perspective, do you find as a founder or product leader that getting users to use and buyers to buy seems harder than it should be? If you lead an internal enterprise data team, have you heard that a ”data product” approach can help—but you’re concerned it’s all hype? My name is Brian T. O’Neill, and on Experiencing Data—one of the top 2% of podcasts in the world—I share the stories of leaders who are leveraging product and UX design to make SAAS analytics, AI applications, and internal data products indispensable to their customers. After all, you can’t create business value with data if the humans in the loop can’t or won’t use your solutions. Every 2 weeks, I release interviews with experts and impressive people I’ve met who are doing interesting work at the intersection of enterprise software product management, UX design, AI and analytics—work that you need to hear about and from whom I hope you can borrow strategies. I also occasionally record solo episodes on applying UI/UX design strategies to data products—so you and your team can unlock financial value by making your users’ and customers’ lives better. Hashtag: #ExperiencingData. JOIN MY INSIGHTS LIST FOR 1-PAGE EPISODE SUMMARIES, TRANSCRIPTS, AND FREE UX STRATEGY TIPS https://designingforanalytics.com/ed ABOUT THE HOST, BRIAN T. O’NEILL: https://designingforanalytics.com/bio/
Episodes
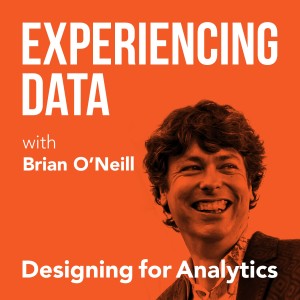
Tuesday Jan 11, 2022
Tuesday Jan 11, 2022
Episode Description
As the conversation around AI continues, Professor Cynthia Rudin, Computer Scientist and Director at the Prediction Analysis Lab at Duke University, is here to discuss interpretable machine learning and her incredible work in this complex and evolving field. To begin, she is the most recent (2021) recipient of the $1M Squirrel AI Award for her work on making machine learning more interpretable to users and ultimately more beneficial to humanity.
In this episode, we explore the distinction between explainable and interpretable machine learning and how black boxes aren’t necessarily “better” than more interpretable models. Cynthia offers up real-world examples to illustrate her perspective on the role of humans and AI, shares takeaways from her previous work which ranges from predicting criminial recidivism to predicting manhole cover explosions in NYC (yes!). I loved this chat with her because, for one, Cynthia has strong, heavily informed opinions from her concentrated work in this area, and secondly, because Cynthia is thinking about both the end users of ML applications as well as the humans who are “out of the loop,” but nonetheless impacted by the decisions made by the users of these AI systems.
In this episode, we cover:
- Background on the Squirrel AI Award – and Cynthia unpacks the differences between Explainable and Interpretable ML. (00:46)
- Using real-world examples, Cynthia demonstrates why black boxes should be replaced. (04:49)
- Cynthia’s work on the New York City power grid project, exploding manhole covers, and why it was the messiest dataset she had ever seen. (08:20)
- A look at the future of machine learning and the value of human interaction as it moves into the next frontier. (15:52)
- Cynthia’s thoughts on collecting end-user feedback and keeping humans in the loop. (21:46)
- The current problems Cynthia and her team are exploring—the Roshomon Set, optimal sparse decision trees, sparse linear models, causal inference, and more. (32:33)
Quotes from Today’s Episode
- “I’ve been trying to help humanity my whole life with AI, right? But it’s not something I tried to earn because there was no award like this in the field while I was trying to do all of this work. But I was just totally amazed, and honored, and humbled that they chose me.”- Cynthia Rudin on receiving the AAAI Squirrel AI Award. (@cynthiarudin) (1:03)
- “Instead of trying to replace the black boxes with inherently interpretable models, they were just trying to explain the black box. And when you do this, there's a whole slew of problems with it. First of all, the explanations are not very accurate—they often mislead you. Then you also have problems where the explanation methods are giving more authority to the black box, rather than telling you to replace them.”- Cynthia Rudin (@cynthiarudin) (03:25)
- “Accuracy at all costs assumes that you have a static dataset and you’re just trying to get as high accuracy as you can on that dataset. [...] But that is not the way we do data science. In data science, if you look at a standard knowledge discovery process, [...] after you run your machine learning technique, you’re supposed to interpret the results and use that information to go back and edit your data and your evaluation metric. And you update your whole process and your whole pipeline based on what you learned. So when people say things like, ‘Accuracy at all costs,’ I’m like, ‘Okay. Well, if you want accuracy for your whole pipeline, maybe you would actually be better off designing a model you can understand.’”- Cynthia Rudin (@cynthiarudin) (11:31)
- “When people talk about the accuracy-interpretability trade-off, it just makes no sense to me because it’s like, no, it’s actually reversed, right? If you can actually understand what this model is doing, you can troubleshoot it better, and you can get overall better accuracy.“- Cynthia Rudin (@cynthiarudin) (13:59)
- “Humans and machines obviously do very different things, right? Humans are really good at having a systems-level way of thinking about problems. They can look at a patient and see things that are not in the database and make decisions based on that information, but no human can calculate probabilities really accurately in their heads from large databases. That’s why we use machine learning. So, the goal is to try to use machine learning for what it does best and use the human for what it does best. But if you have a black box, then you’ve effectively cut that off because the human has to basically just trust the black box. They can’t question the reasoning process of it because they don’t know it.”- Cynthia Rudin (@cynthiarudin) (17:42)
- “Interpretability is not always equated with sparsity. You really have to think about what interpretability means for each domain and design the model to that domain, for that particular user.”- Cynthia Rudin (@cynthiarudin) (19:33)
- “I think there's sometimes this perception that there's the truth from the data, and then there's everything else that people want to believe about whatever it says.”- Brian T. O’Neill (@rhythmspice) (23:51)
- “Surveys have their place, but there's a lot of issues with how we design surveys to get information back. And what you said is a great example, which is 7 out of 7 people said, ‘this is a serious event.’ But then you find out that they all said serious for a different reason—and there's a qualitative aspect to that. […] The survey is not going to tell us if we should be capturing some of that information if we don't know to ask a question about that.”- Brian T. O’Neill (@rhythmspice) (28:56)
Links
- Squirrel AI Award: https://aaai.org/Pressroom/Releases/release-21-1012.php
- “Machine Bias”: https://www.propublica.org/article/machine-bias-risk-assessments-in-criminal-sentencing
- Users.cs.duke.edu/~cynthia: https://users.cs.duke.edu/~cynthia
- Teaching: https://users.cs.duke.edu/~cynthia/teaching.html
No comments yet. Be the first to say something!