
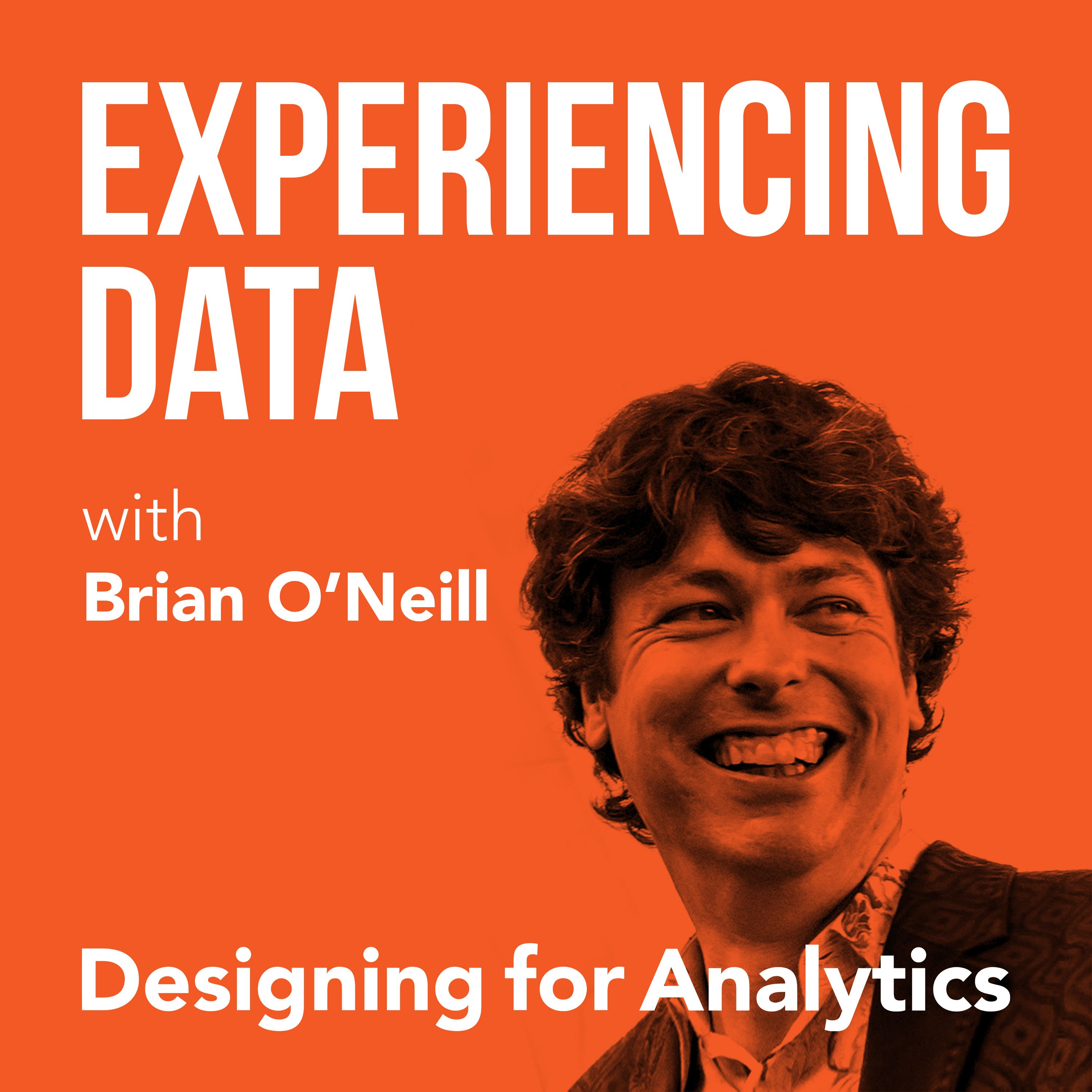
137.9K
Downloads
170
Episodes
Is the value of your enterprise analytics SAAS or AI product not obvious through it’s UI/UX? Got the data and ML models right...but user adoption of your dashboards and UI isn’t what you hoped it would be? While it is easier than ever to create AI and analytics solutions from a technology perspective, do you find as a founder or product leader that getting users to use and buyers to buy seems harder than it should be? If you lead an internal enterprise data team, have you heard that a ”data product” approach can help—but you’re concerned it’s all hype? My name is Brian T. O’Neill, and on Experiencing Data—one of the top 2% of podcasts in the world—I share the stories of leaders who are leveraging product and UX design to make SAAS analytics, AI applications, and internal data products indispensable to their customers. After all, you can’t create business value with data if the humans in the loop can’t or won’t use your solutions. Every 2 weeks, I release interviews with experts and impressive people I’ve met who are doing interesting work at the intersection of enterprise software product management, UX design, AI and analytics—work that you need to hear about and from whom I hope you can borrow strategies. I also occasionally record solo episodes on applying UI/UX design strategies to data products—so you and your team can unlock financial value by making your users’ and customers’ lives better. Hashtag: #ExperiencingData. JOIN MY INSIGHTS LIST FOR 1-PAGE EPISODE SUMMARIES, TRANSCRIPTS, AND FREE UX STRATEGY TIPS https://designingforanalytics.com/ed ABOUT THE HOST, BRIAN T. O’NEILL: https://designingforanalytics.com/bio/
Episodes
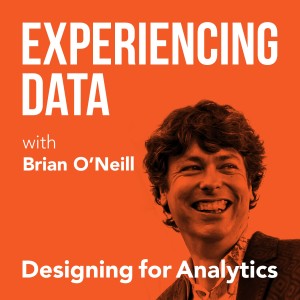
Tuesday May 31, 2022
Tuesday May 31, 2022
Today I’m talking about how to measure data product value from a user experience and business lens, and where leaders sometimes get it wrong. Today’s first question was asked at my recent talk at the Data Summit conference where an attendee asked how UX design fits into agile data product development. Additionally, I recently had a subscriber to my Insights mailing list ask about how to measure adoption, utilization, and satisfaction of data products. So, we’ll jump into that juicy topic as well.
Answering these inquiries also got me on a related tangent about the UX challenges associated with abstracting your platform to support multiple, but often theoretical, user needs—and the importance of collaboration to ensure your whole team is operating from the same set of assumptions or definitions about success. I conclude the episode with the concept of “game framing” as a way to conceptualize these ideas at a high level.
Key topics and cues in this episode include:
- An overview of the questions I received (:45)
- Measuring change once you’ve established a benchmark (7:45)
- The challenges of working in abstractions (abstracting your platform to facilitate theoretical future user needs) (10:48)
- The value of having shared definitions and understanding the needs of different stakeholders/users/customers (14:36)
- The importance of starting from the “last mile” (19:59)
- The difference between success metrics and progress metrics (24:31)
- How measuring feelings can be critical to measuring success (29:27)
- “Game framing” as a way to understand tracking progress and success (31:22)
Quotes from Today’s Episode
- “Once you’ve got your benchmark in place for a data product, it’s going to be much easier to measure what the change is because you’ll know where you’re starting from.” - Brian (7:45)
- “When you’re deploying technology that’s supposed to improve people’s lives so that you can get some promise of business value downstream, this is not a generic exercise. You have to go out and do the work to understand the status quo and what the pain is right now from the user's perspective.” - Brian (8:46)
- “That user perspective—perception even—is all that matters if you want to get to business value. The user experience is the perceived quality, usability, and utility of the data product.” - Brian (13:07)
- “A data product leader’s job should be to own the problem and not just the delivery of data product features, applications or technology outputs. ” - Brian (26:13)
- “What are we keeping score of? Different stakeholders are playing different games so it’s really important for the data product team not to impose their scoring system (definition of success) onto the customers, or the users, or the stakeholders.” - Brian (32:05)
- “We always want to abstract once we have a really good understanding of what people do, as it’s easier to create more user-centered abstractions that will actually answer real data questions later on. ” - Brian (33:34)
Links
- https://designingforanalytics.com/community
Comments (0)
To leave or reply to comments, please download free Podbean or
No Comments
To leave or reply to comments,
please download free Podbean App.