
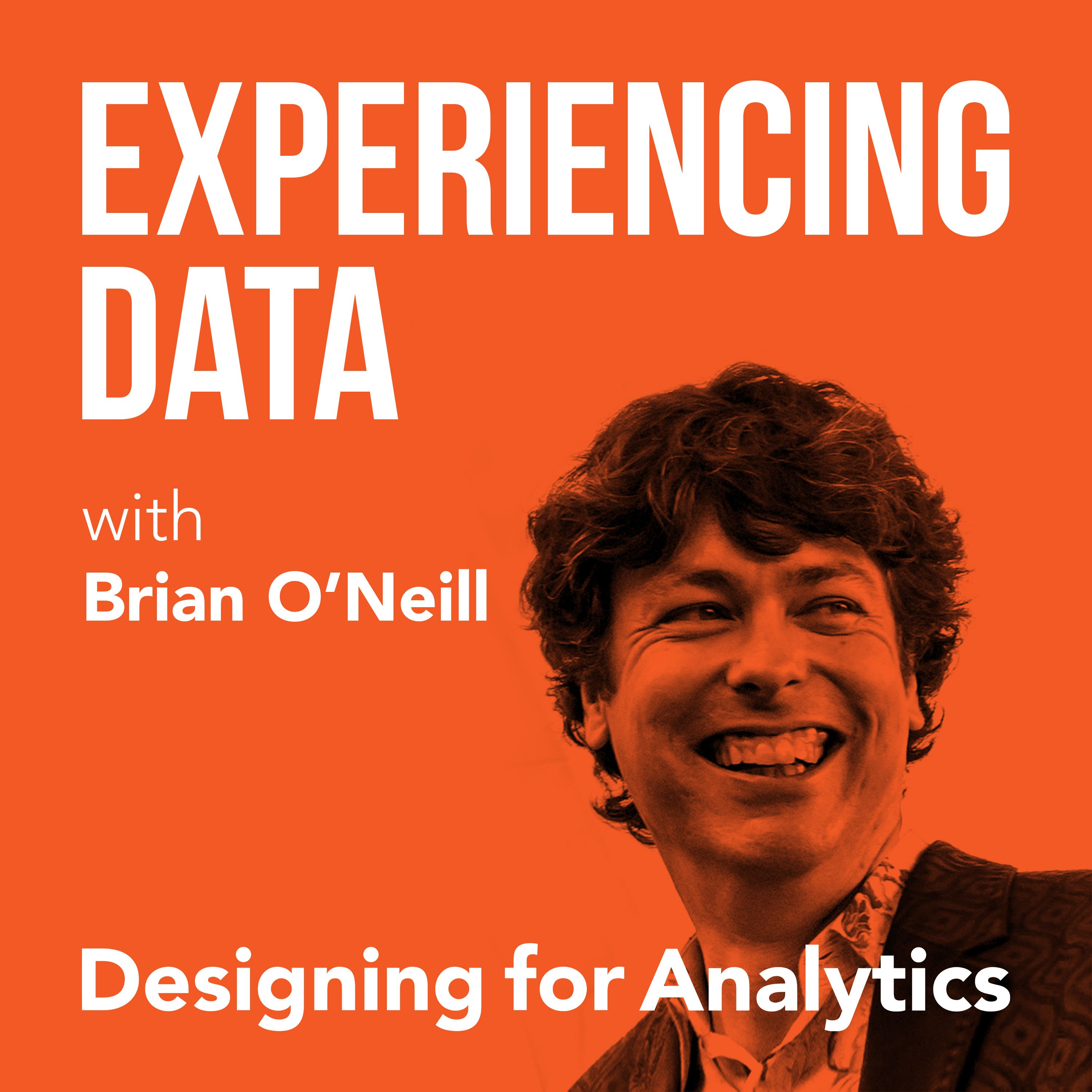
142.2K
Downloads
174
Episodes
Is the value of your enterprise analytics SAAS or AI product not obvious through it’s UI/UX? Got the data and ML models right...but user adoption of your dashboards and UI isn’t what you hoped it would be? While it is easier than ever to create AI and analytics solutions from a technology perspective, do you find as a founder or product leader that getting users to use and buyers to buy seems harder than it should be? If you lead an internal enterprise data team, have you heard that a ”data product” approach can help—but you’re concerned it’s all hype? My name is Brian T. O’Neill, and on Experiencing Data—one of the top 2% of podcasts in the world—I share the stories of leaders who are leveraging product and UX design to make SAAS analytics, AI applications, and internal data products indispensable to their customers. After all, you can’t create business value with data if the humans in the loop can’t or won’t use your solutions. Every 2 weeks, I release interviews with experts and impressive people I’ve met who are doing interesting work at the intersection of enterprise software product management, UX design, AI and analytics—work that you need to hear about and from whom I hope you can borrow strategies. I also occasionally record solo episodes on applying UI/UX design strategies to data products—so you and your team can unlock financial value by making your users’ and customers’ lives better. Hashtag: #ExperiencingData. JOIN MY INSIGHTS LIST FOR 1-PAGE EPISODE SUMMARIES, TRANSCRIPTS, AND FREE UX STRATEGY TIPS https://designingforanalytics.com/ed ABOUT THE HOST, BRIAN T. O’NEILL: https://designingforanalytics.com/bio/
Episodes
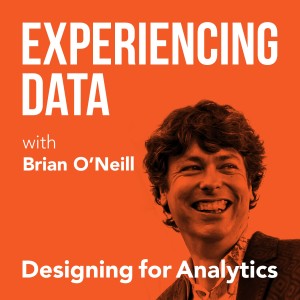
Tuesday Sep 06, 2022
Tuesday Sep 06, 2022
Today I’m sitting down with Jon Cooke, founder and CTO of Dataception, to learn his definition of a data product and his views on generating business value with your data products. In our conversation, Jon explains his philosophy on data products and where design and UX fit in. We also review his conceptual model for data products (which he calls the data product pyramid), and discuss how together, these concepts allow teams to ship working solutions faster that actually produce value.
Highlights/ Skip to:
- Jon’s definition of a data product (1:19)
- Brian explains how UX research and design planning can and should influence data architecture —so that last mile solutions are useful and usable (9:47)
- The four characteristics of a data product in Jon’s model (16:16)
- The idea of products having a lifecycle with direct business/customer interaction/feedback (17:15)
- Understanding Jon’s data product pyramid (19:30)
- The challenges when customers/users don’t know what they want from data product teams - and who should be doing the work to surface requirements (24:44)
- Mitigating risk and the importance of having management buy-in when adopting a product-driven approach (33:23)
- Does the data product pyramid account for UX? (35:02)
- What needs to change in an org model that produces data products that aren’t delivering good last mile UXs (39:20)
Quotes from Today’s Episode
- “A data product is something that specifically solves a business problem, a piece of analytics, data use case, a pipeline, datasets, dashboard, that type that solves a business use case, and has a customer, and as a product lifecycle to it.” - Jon (2:15)
- “I’m a fan of any definition that includes some type of deployment and use by some human being. That’s the end of the cycle, because the idea of a product is a good that has been made, theoretically, for sale.” - Brian (5:50)
- “We don’t build a lot of stuff around cloud anymore. We just don’t build it from scratch. It’s like, you know, we don’t generate our own electricity, we don’t mill our own flour. You know, the cloud—there’s a bunch of composable services, which I basically pull together to build my application, whatever it is. We need to apply that thinking all the way through the stack, fundamentally.” - Jon (13:06)
- “It’s not a data science problem, it’s not a business problem, it’s not a technology problem, it’s not a data engineering problem, it’s an everyone problem. And I advocate small, multidisciplinary teams, which have a business value person in it, have an SME, have a data scientist, have a data architect, have a data engineer, as a small pod that goes in and answer those questions.” - Jon (26:28)
- “The idea is that you’re actually building the data products, which are the back-end, but you’re actually then also doing UX alongside that, you know? You’re doing it in tandem.” - Jon (37:36)
- “Feasibility is one of the legs of the stools. There has to be market need, and your market just may be the sales team, but there needs to be some promise of value there that this person is really responsible for at the end of the day, is this data product going to create value or not?” - Brian (42:35)
- “The thing about data products is sometimes you don’t know how feasible it is until you actually look at the data…You’ve got to do what we call data archaeology. You got to go and find the data, you got to brush it off, and you’re looking at and go, ‘Is it complete?’” - Jon (44:02)
No comments yet. Be the first to say something!