
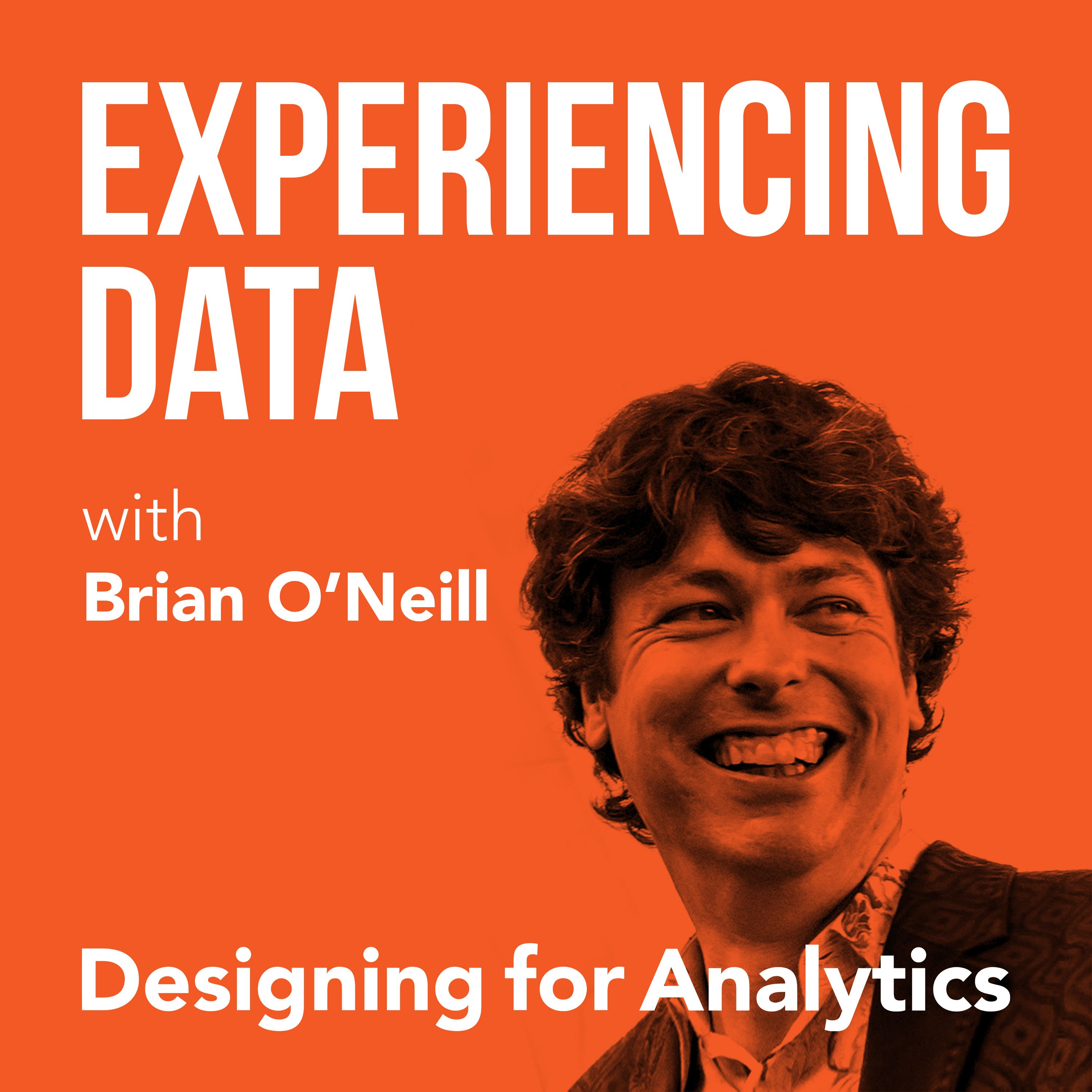
127.5K
Downloads
161
Episodes
If you’re a leader tasked with generating business and org. value through ML/AI and analytics, you’ve probably struggled with low user adoption. Making the tech gets easier, but getting users to use, and buyers to buy, remains difficult—but you’ve heard a ”data product” approach can help. Can it? My name is Brian T. O’Neill, and on Experiencing Data—one of the top 2% of podcasts in the world—I offer you a consulting designer’s perspective on why creating ML and analytics outputs isn’t enough to create business and UX outcomes. How can UX design and product management help you create innovative ML/AI and analytical data products? What exactly are data products—and how can data product management help you increase user adoption of ML/analytics—so that stakeholders can finally see the business value of your data? Every 2 weeks, I answer these questions via solo episodes and interviews with innovative chief data officers, data product management leaders, and top UX professionals. Hashtag: #ExperiencingData. PODCAST HOMEPAGE: Get 1-page summaries, text transcripts, and join my Insights mailing list: https://designingforanalytics.com/ed ABOUT THE HOST, BRIAN T. O’NEILL: https://designingforanalytics.com/bio/
Episodes
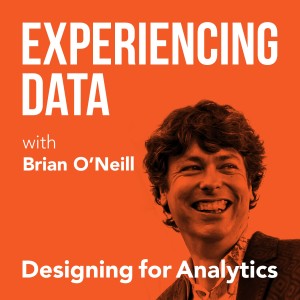
Tuesday Sep 05, 2023
Tuesday Sep 05, 2023
Today I’m joined by Vera Liao, Principal Researcher at Microsoft. Vera is a part of the FATE (Fairness, Accountability, Transparency, and Ethics of AI) group, and her research centers around the ethics, explainability, and interpretability of AI products. She is particularly focused on how designers design for explainability. Throughout our conversation, we focus on the importance of taking a human-centered approach to rendering model explainability within a UI, and why incorporating users during the design process informs the data science work and leads to better outcomes. Vera also shares some research on why example-based explanations tend to out-perform [model] feature-based explanations, and why traditional XAI methods LIME and SHAP aren’t the solution to every explainability problem a user may have.
Highlights/ Skip to:
- I introduce Vera, who is Principal Researcher at Microsoft and whose research mainly focuses on the ethics, explainability, and interpretability of AI (00:35)
- Vera expands on her view that explainability should be at the core of ML applications (02:36)
- An example of the non-human approach to explainability that Vera is advocating against (05:35)
- Vera shares where practitioners can start the process of responsible AI (09:32)
- Why Vera advocates for doing qualitative research in tandem with model work in order to improve outcomes (13:51)
- I summarize the slides I saw in Vera’s deck on Human-Centered XAI and Vera expands on my understanding (16:06)
- Vera’s success criteria for explainability (19:45)
- The various applications of AI explainability that Vera has seen evolve over the years (21:52)
- Why Vera is a proponent of example-based explanations over model feature ones (26:15)
- Strategies Vera recommends for getting feedback from users to determine what the right explainability experience might be (32:07)
- The research trends Vera would most like to see technical practitioners apply to their work (36:47)
- Summary of the four-step process Vera outlines for Question-Driven XAI design (39:14)
Comments (0)
To leave or reply to comments, please download free Podbean or
No Comments
To leave or reply to comments,
please download free Podbean App.