
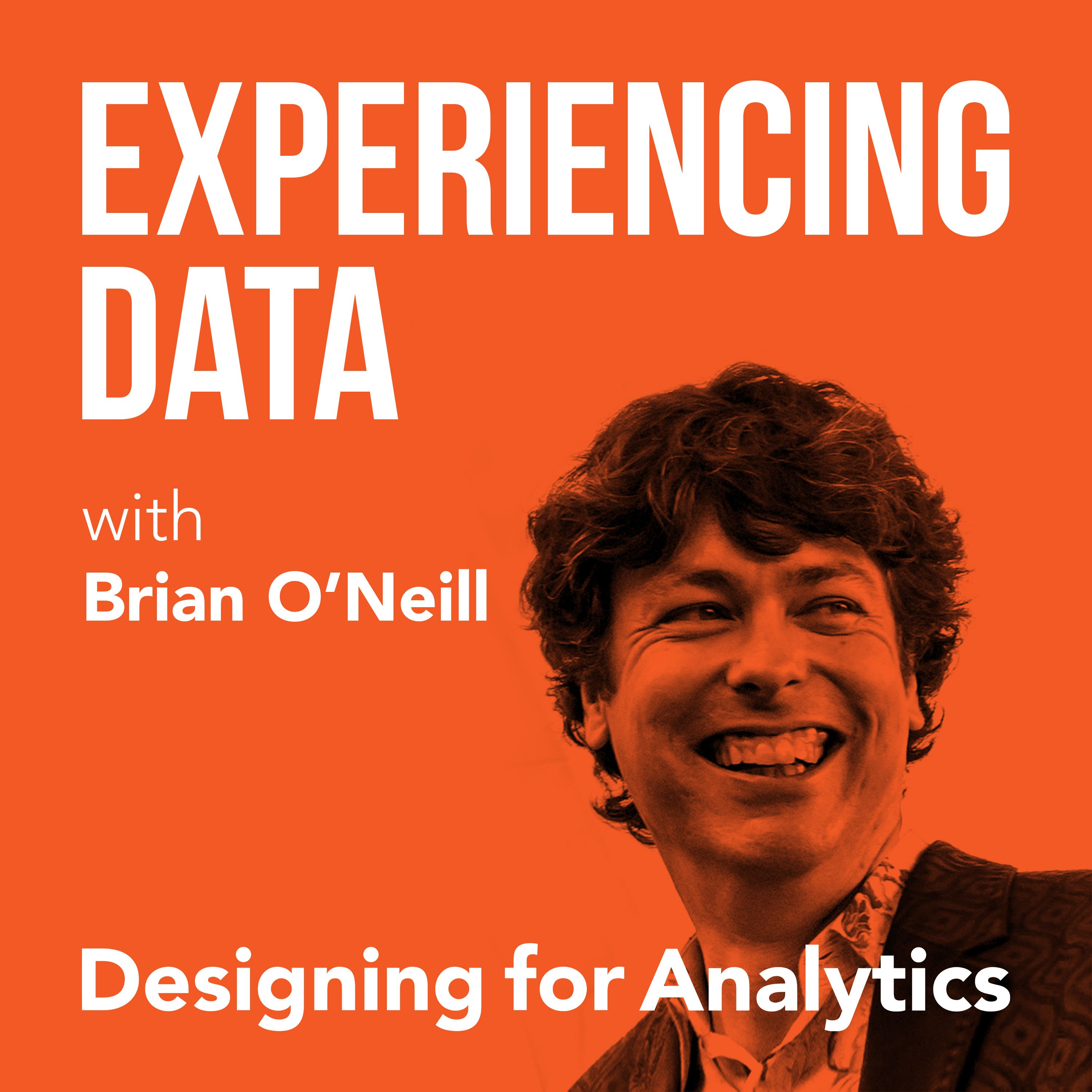
141.5K
Downloads
173
Episodes
Is the value of your enterprise analytics SAAS or AI product not obvious through it’s UI/UX? Got the data and ML models right...but user adoption of your dashboards and UI isn’t what you hoped it would be? While it is easier than ever to create AI and analytics solutions from a technology perspective, do you find as a founder or product leader that getting users to use and buyers to buy seems harder than it should be? If you lead an internal enterprise data team, have you heard that a ”data product” approach can help—but you’re concerned it’s all hype? My name is Brian T. O’Neill, and on Experiencing Data—one of the top 2% of podcasts in the world—I share the stories of leaders who are leveraging product and UX design to make SAAS analytics, AI applications, and internal data products indispensable to their customers. After all, you can’t create business value with data if the humans in the loop can’t or won’t use your solutions. Every 2 weeks, I release interviews with experts and impressive people I’ve met who are doing interesting work at the intersection of enterprise software product management, UX design, AI and analytics—work that you need to hear about and from whom I hope you can borrow strategies. I also occasionally record solo episodes on applying UI/UX design strategies to data products—so you and your team can unlock financial value by making your users’ and customers’ lives better. Hashtag: #ExperiencingData. JOIN MY INSIGHTS LIST FOR 1-PAGE EPISODE SUMMARIES, TRANSCRIPTS, AND FREE UX STRATEGY TIPS https://designingforanalytics.com/ed ABOUT THE HOST, BRIAN T. O’NEILL: https://designingforanalytics.com/bio/
Episodes
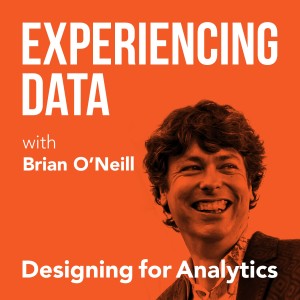
Tuesday Feb 18, 2025
Tuesday Feb 18, 2025
I keep hearing data product, data strategy, and UX teams often struggle to quantify the value of their work. Whether it’s as a team as a whole or on a specific data product initiative, the underlying problem is the same – your contribution is indirect, so it’s harder to measure. Even worse, your stakeholders want to know if your work is creating an impact and value, but because you can’t easily put numbers on it, valuation spirals into a messy problem.
The messy part of this valuation problem is what today’s episode is all about—not math! Value is largely subjective, not objective, and I think this is partly why analytical teams may struggle with this. To improve at how you estimate the value of your data products, you need to leverage other skills—and stop approaching this as a math problem.
As a consulting product designer, estimating value when it’s indirect is something that I’ve dealt with my entire career. It’s not a skill learned overnight, and it’s one you will need to keep developing over time—but the basic concepts are simple. I hope you’ll find some value in applying these along with your other frameworks and tools.
Highlights/ Skip to
- Value is subjective, not objective (5:01)
- Measurability does not necessarily mean valuable (6:36)
- Businesses are made up of humans. Most b2b stakeholders aren’t spending their own money when making business decisions—what does that mean for your work? (9:30)
- Quantifying a data product’s value starts with understanding what is worth measuring in the eye of the beholder(s)—not math calculations (13:44)
- The more difficult it is to show the value of your product (or team) in numbers, the lower that value is to the stakeholder—initially (16:46)
- By simply helping a stakeholder to think through how value should be calculated on a data product, you’re likely already providing additional value (18:02)
- Focus on expressing estimated value via a range versus a single number (19:36)
- Measurement of anything requires that we can observe the phenomenon first—but many stakeholders won’t be able to cite these phenomena without [your!] help (22:16)
- When you are measuring quantitative aspects of value, remember that measurement is not the same as accuracy (precision)—and the precision game can become a trap (25:37)
- How to measure anything—and why estimates often trump accuracy (31:19)
- Why you may need to steer the conversation away from ROI calculations in the short term (35:00)
Quotes from Today’s Episode
- Even when you can easily assign a dollar value to the data product you’re building, that does not necessarily reflect what your stakeholder actually feels about it—or your team’s contribution. So, why do they keep asking you to quantify the value of your work? By actually understanding what a shareholder needs to observe for them to know progress has been made on their initiative or data product, you will be positioned to deliver results they actually care about. While most of the time, you should be able to show some obvious economic value in the work you’re doing, you may be getting hounded about this because you’re not meeting the often unstated qualitative goals. If you can surface the qualitative goals of your stakeholder, then the perception of the value of your team and its work goes up, and you’ll spend less time trying to measure an indirect contribution in quant terms that only has a subjectively right answer. (6:50)
- The more difficult it is for you to show the monetary value of your data product (or team), the lower that value likely is to the stakeholder. This does not mean the value of your work is “low.” It means it’s perceived as low because it cannot be easily quantified in a way that is observable to the person whose judgment matters. By understanding the personal motivations and interests of your stakeholders, you can begin to collaboratively figure out what the correct success metrics should be—and how they’d be measured. By just simply beginning to ask and uncover what they’re trying to measure, you can start to increase your contributions’ perceived value. (17:01)
- Think about expressing “indirect value” as a range, not a precise single value. It’s much easier to refine your estimate (if necessary) once a range has been defined, and you only need to get precise enough for your stakeholder to make a decision with the information. How much time should you spend refining your measurement of the value? Potentially little to none—if the “better math” isn’t going to change anyone’s mind or decision. Spending more time to measure a data product’s value more accurately takes you away from doing actual product work—and if there isn’t much obvious value to the work, maybe the work—not the measurement of the work—needs to change. (19:49)
- Smart leaders know that deriving a simple calculation of indirect contributions is complex—otherwise, the topic wouldn’t keep coming up. There is a “why” behind why they’re asking, and when you understand the “why,” you’ll be better positioned to deliver the value they actually seek, using valuation measurements that are “just enough” in their precision. What do you think it says to a stakeholder if you’re spending an inordinate amount of time simply trying to calculate and explain the value of your data product? (23:22)
- Many organizations for years have invested in things that don’t always have a short term ROI. They know that ROI takes time, and they can’t really measure what it’s worth along the way. Examples include investments in company culture, innovation, brand reputation, and many others. If you’re constantly playing defense and having to justify your existence or methods by quantifying the financial value of your data products (or data product management team, or UX team, or any other indirect contributor/contribution), then either your work truly does lack value, or you haven’t surfaced what the actual success metrics and outcomes are— in the eyes of the stakeholder. As such, the perceived value is “low” or opaque. They might be looking for a hard number to assign to it because they’re not seeing any of the other forms of value that they care about that would indicate positive progress. It’s easier to write [you] a large check for a big, innovative, unproven initiative if your stakeholders know what you and your team can accomplish with a small check. (35:16)
No comments yet. Be the first to say something!