
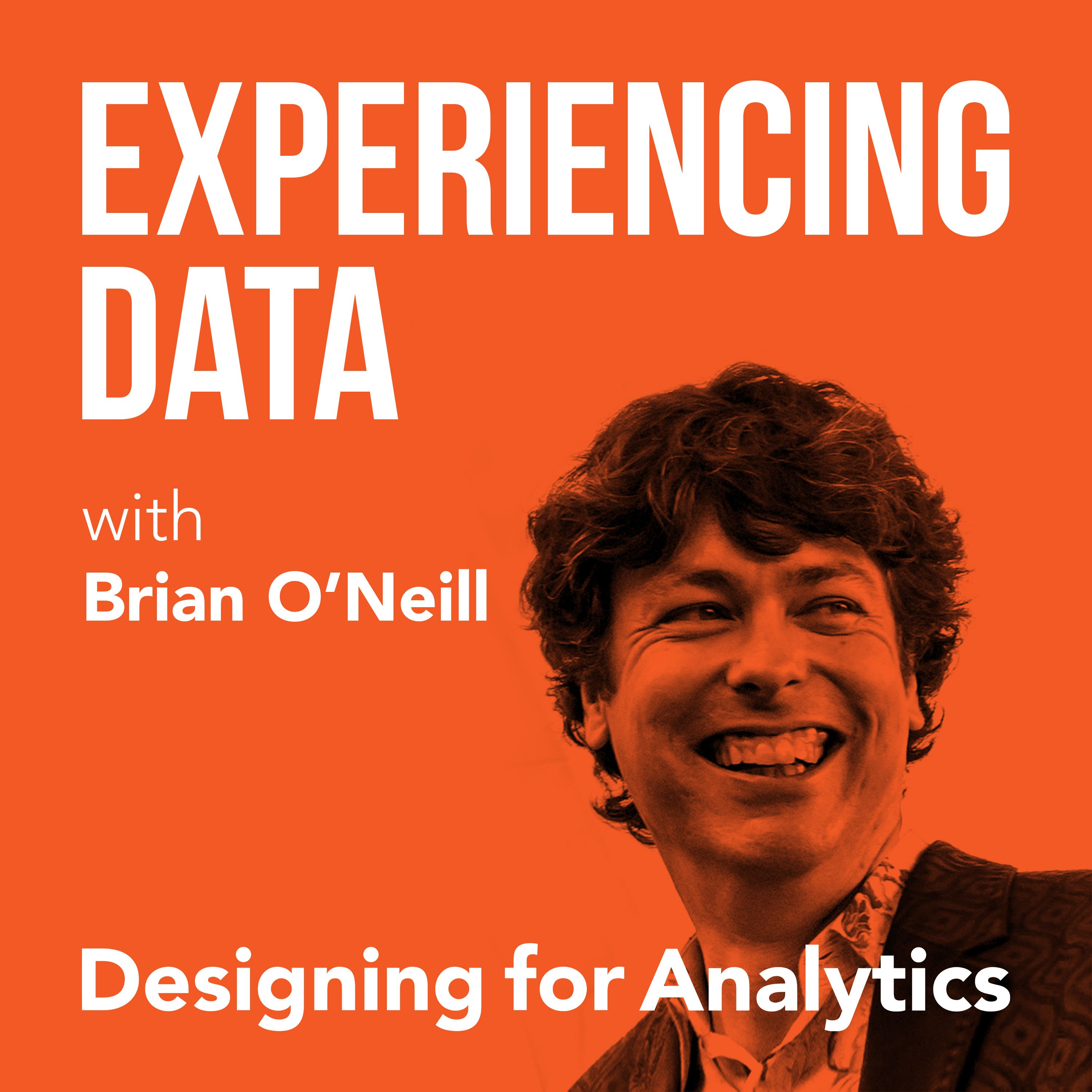
134.4K
Downloads
167
Episodes
Is the value of your enterprise analytics SAAS or AI product not obvious through it’s UI/UX? Got the data and ML models right...but user adoption of your dashboards and UI isn’t what you hoped it would be? While it is easier than ever to create AI and analytics solutions from a technology perspective, do you find as a founder or product leader that getting users to use and buyers to buy seems harder than it should be? If you lead an internal enterprise data team, have you heard that a ”data product” approach can help—but you’re concerned it’s all hype? My name is Brian T. O’Neill, and on Experiencing Data—one of the top 2% of podcasts in the world—I share the stories of leaders who are leveraging product and UX design to make SAAS analytics, AI applications, and internal data products indispensable to their customers. After all, you can’t create business value with data if the humans in the loop can’t or won’t use your solutions. Every 2 weeks, I release interviews with experts and impressive people I’ve met who are doing interesting work at the intersection of enterprise software product management, UX design, AI and analytics—work that you need to hear about and from whom I hope you can borrow strategies. I also occasionally record solo episodes on applying UI/UX design strategies to data products—so you and your team can unlock financial value by making your users’ and customers’ lives better. Hashtag: #ExperiencingData. JOIN MY INSIGHTS LIST FOR 1-PAGE EPISODE SUMMARIES, TRANSCRIPTS, AND FREE UX STRATEGY TIPS https://designingforanalytics.com/ed ABOUT THE HOST, BRIAN T. O’NEILL: https://designingforanalytics.com/bio/
Episodes
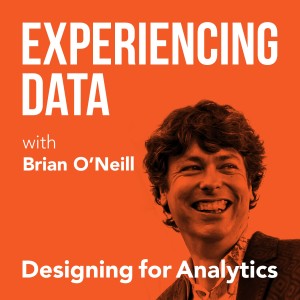
Tuesday Oct 19, 2021
Tuesday Oct 19, 2021
Why do we need or care about design in the work of data science? Jesús Templado, Managing Director at Bedrock, is here to tell us about how Bedrock executes their mantra, “data by design.”
Bedrock has found ways to bring to their clients a design-driven, human-centered approach by utilizing a “hybrid model” to synthesize technical possibilities with human needs. In this episode, we explore Bedrock’s vision for how to achieve this synthesis as part of the firm’s DNA, and how Bedrock adopted their vision to make data more approachable with the client being central to their design efforts. Jesús also discusses a time when he championed making “data by design” a successful strategy with a large chain of hotels, and he offers insight on how making clients feel validated and heard plays a part.
In our chat, we also covered:
- “Data by design” and how Bedrock implements this design-driven approach. (00:43)
- Bedrock’s vision for how they support their clients and why design has always been part of their DNA. (08:53)
- Jesús shares a time when he successfully implemented a design process for a large chain of hotels, and some of the challenges that came with that approach. (14:47)
- The importance of making clients feel heard by dedicating time to research and UX and how the team navigates conversations about risk with customers. (24:12)
- More on the client experience and how Bedrock covers a large spectrum of areas to ensure that they deliver a product that makes sense for the customer. (33:01)
- Jesús’ opinion on why companies should consider change management when building products and systems - and a look at the Data Stand-Up podcast (35:42)
Quotes from Today’s Episode
“Many people in corporations don’t have the technical background to understand the possibilities when it comes to analyzing or using data. So, bringing a design-based framework, such as design thinking, is really important for all of the work that we do for our clients.” - Jesús Templado (2:33)
“We’ve mentioned “data by design” before as our mantra; we very much prefer building long-lasting relationships based on [understanding] our clients' business and their strategic goals. We then design and ideate an implementation roadmap with them and then based on that, we tackle different periods for building different models. But we build the models because we understand what’s going to bring us an outcome for the business—not because the business brings us in to deliver only a model for the sake of predicting what the weather is going to be in two weeks.”- Jesús Templado (14:07)
“I think as consultants and people in service, it’s always nice to make friends. And, I like when I can call a client a friend, but I feel like I’m really here to help them deliver a better future state [...] And the road may be bumpy, especially if design is a new thing. And it is often new; in the context of data science and analytics projects.”- Brian T. O’Neill (@rhythmspice) (26:49)
“When we do data science [...] that’s a means to an end. We do believe it’s important that the client understands the reasoning behind everything that we do and build, but at the end of the day, it’s about understanding that business problem, understanding the challenge that the company is facing, knowing what the expected outcome is, and knowing how you will deliver or predict that outcome to be used for something meaningful and relevant for the business.”- Jesús Templado (33:06)
“The appetite for innovation is high, but a lot of the companies that want to do it are more concerned about risk. Risk and innovation are at opposite ends of the spectrum. And so, if you want to be innovative, by definition—you’re signing up for failure on the way to success. [...] It’s about embracing an iterative process, it’s about getting feedback along the way, it’s about knowing that we don’t know everything, and we’re signing up for that ambiguity along the way to something better.”- Brian T. O’Neill (@rhythmspice) (38:20)
Links Referenced
- Bedrock: https://bedrockdbd.com
- Data Stand-Up podcast: https://bedrockdbd.com/podcast/
- LinkedIn: https://www.linkedin.com/in/Jesústg/
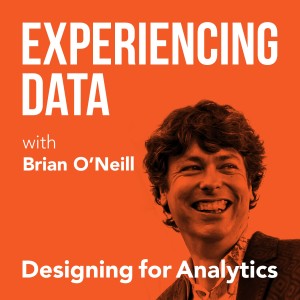
Tuesday Oct 05, 2021
Tuesday Oct 05, 2021
How do we get the most breadth out of design and designers when building data products? One way is to have designers be at the front leading the charge when it comes to creating data products that must be useful, usable, and valuable.
For this episode Prasad Vadlamani, CDW’s Director of Data Science and Advanced Analytics, joins us for a chat about how they are making design a larger focus of how they create useful, usable data products. Prasad talks about the importance of making technology—including AI-driven solutions—human centered, and how CDW tries to keep the end user in mind.
Prasad and I also discuss his perspectives on how to build designers into a data product team and how to successfully navigate the grey areas between various areas of expertise. When this is done well, then the entire team can work with each other's strengths and advantages to create a more robust product. We also discuss the role a UI-free user experience plays in some data products, some differences between external and internally-facing solutions, and some of Prasad’s valuable takeaways that have helped to shape the way he thinks design, data science, and analytics can collaborate.
In our chat, we covered:
- Prasad’s first introduction to designers and how he leverages the disciplines of design and product in his data science and analytics work (1:09)
- The terminology behind product manager and designer and how these functions play a role in an enterprise AI team (5:18)
- How teams can use their wide range of competencies to their advantage (8:52)
- A look at one UI-less experience and the value of the “invisible interface” (14:58)
- Understanding the model development process and why the model takes up only a small percentage of the effort required to successfully bring a data product to end users (20:52)
- The differences between building an internal vs external product, what to consider, and Prasad’s “customer zero” approach. (29.17)
- Expectations Prasad sets with customers (stakeholders) about the life expectancy of data products when they are in their early stage of development (35:02)
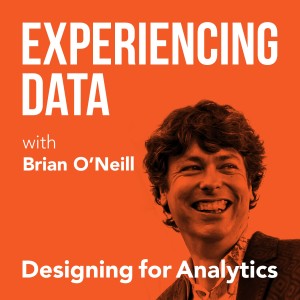
Tuesday Sep 21, 2021
Tuesday Sep 21, 2021
Episode Description
The challenges of design and AI are exciting ones to face. The key to being successful in that space lies in many places, but one of the most important is instituting the right design language.
For Abhay Agarwal, Founder of Polytopal, when he began to think about design during his time at Microsoft working on systems to help the visually impared, he realized the necessity of a design language for AI. Stepping away from that experience, he leaned into how to create a new methodology of design centered around human needs. His efforts have helped shift the lens of design towards how people solve problems.
In this episode, Abhay and I go into details on a snippet from his course page for the Stanford d. where he claimed that “the foreseeable future would not be well designed, given the difficulty of collaboration between disciplines.” Abhay breaks down how he thinks his design language for AI should work and how to build it out so that everyone in an organization can come to a more robust understanding of AI. We also discuss the future of designers and AI and the ebb and flow of changing, learning, and moving forward with the AI narrative.
In our chat, we covered:
- Abhay’s background in AI research and what happened to make him move towards design as a method to produce intelligence from messy data. (1:01)
- Why Abhay has come up with a new design language called Lingua Franca for machine learning products [and his course on this at Stanford’s d.school]. (3:21)
- How to become more human-centered when building AI products, what ethnographers can uncover, and some of Abhay’s real-world examples. (8:06)
- Biases in design and the challenges in developing a shared language for both designers and AI engineers. (15:59)
- Discussing interpretability within black box models using music recommendation systems, like Spotify, as an example. (19:53)
- How “unlearning” solves one of the biggest challenges teams face when collaborating and engaging with each other. (27:19)
- How Abhay is shaping the field of design and ML/AI -- and what’s in store for Lingua Franca. (35:45)
Quotes from Today's Episode
“I certainly don’t think that one needs to hit the books on design thinking or listen to a design thinker describe their process in order to get the fundamentals of a human-centered design process. I personally think it’s something that one can describe to you within the span of a single conversation, and someone who is listening to that can then interpret that and say, ‘Okay well, what am I doing that could be more human-centered?’ In the AI space, I think this is the perennial question.” - Abhay Agarwal (@Denizen_Kane) (6:30)
“Show me a company where designers feel at an equivalent level to AI engineers when brainstorming technology? It just doesn’t happen. There’s a future state that I want us to get to that I think is along those lines. And so, I personally see this as, kind of, a community-wide discussion, engagement, and multi-strategy approach.” - Abhay Agarwal (@Denizen_Kane) (18:25)
“[Discussing ML data labeling for music recommenders] I was just watching a video about drum and bass production, and they were talking about, “Or you can write your bass lines like this”—and they call it reggaeton. And it’s not really reggaeton at all, which was really born in Puerto Rico. And Brazil does the same thing with their versions of reggae. It’s not the one-drop reggae we think of Bob Marley and Jamaica. So already, we’ve got labeling issues—and they’re not even wrong; it’s just that that’s the way one person might interpret what these musical terms mean” - Brian O’Neill (@rhythmspice) (25:45)
“There is a new kind of hybrid role that is emerging that we play into...which is an AI designer, someone who is very proficient with understanding the dynamics of AI systems. The same way that we have digital UX designers, app designers—there had to be apps before they could be app designers—there is now AI, and then there can thus be AI designers.” - Abhay Agarwal (@Denizen_Kane) (33:47)
Links Referenced
- Lingua Franca: https://linguafranca.polytopal.ai
- Polytopal.ai: https://polytopal.ai
- Polytopal email: hello@polytopal.ai
- LinkedIn: https://www.linkedin.com/in/abhaykagarwal/
- Personal Twitter: https://twitter.com/Denizen_Kane
- Polytopal Twitter: https://twitter.com/polytopal_ai
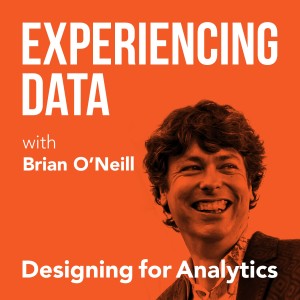
Tuesday Sep 07, 2021
Tuesday Sep 07, 2021
Episode Description
Simply put, data products help users make better decisions and solve problems with information. But how effective can data products be if designers don’t take the time to explore the complete needs of users?
To Param Venkataraman, Chief Design Officer at Fractal Analytics, having an understanding of the “human dimension” of a problem is crucial to creating data solutions that create impact.
On this episode of Experiencing Data, Param and I talk more about his concept of ‘attractive non-conscious design,’ the core skills of a professional designer, and why Fractal has a c-suite design officer and is making large investments in UX.
In our chat, we covered:
- Param's role as Chief Design Officer at Fractal Analytics, and the company's sharp focus on the 'human dimension' of enterprise data products. (2:04)
- 'Attractive non-conscious design': Creating easy-to-use, 'delightful' data products that help end-users make better decisions by focusing on their needs. (5:32)
- The importance of understanding the 'emotional need' of users when designing enterprise data products. (9:07)
- Why designers as well as data science and analytics teams should focus more on the emotional and human element when building data products. (16:15)
- 'The next version of design': Why and how Param believes the classic design thinking model must adapt to the 'post-data science world.' (21:39)
- The core competencies of a professional designer and how it relates to data products. (25:59)
- Why non-designers should learn the principles of good design — and how Fractal’s internal Phi Design System helps frame problems from the perspective of a data product's end-user, leading to better solutions. (27:51)
- Why Param believes the coming together of design and data still needs time to mature. (33:40)
Quotes from Today’s Episode
“When you look at analytics and the AI space … there is so much that is about how do you use ... machine learning … [or] any other analytics technology or solutions — and how do you make better effective decisions? That’s at the heart of it, which is how do we make better decisions?” - Param Venkataraman (@onwardparam) (6:23)
“[When it comes to business software,] most of it should be invisible; you shouldn’t really notice it. And if you’re starting to notice it, you’re probably drawing attention to the wrong thing because you’re taking people out of flow.” - Brian O’Neill (@rhythmspice) (8:57)
“Design is kind of messy … there’s sort of a process ... but it’s not always linear, and we don’t always start at step zero. … You might come into something that’s halfway done and the first thing we do is run a usability study on a competitor’s thing, or on what we have now, and then we go back to step two, and then we go to five. It’s not serial, and it’s kind of messy, and that’s normal.” - Brian O’Neill (@rhythmspice) (16:18)
“Just like design is iterative, data science also is very iterative. There’s the idea of hypothesis, and there’s an idea of building and experimenting, and then you sort of learn and your algorithm learns, and then you get better and better at it.” - Param Venkataraman (@onwardparam) (18:05)
“The world of data science is not used to thinking in terms of emotion, experience, and the so-called softer aspects of things, which in my opinion, is not actually the softer; it’s actually the hardest part. It’s harder to dimensionalize emotion, experience, and behavior, which is … extremely complex, extremely layered, [and] extremely unpredictable. … I think the more we can bring those two worlds together, the world of evidence, the world of data, the world of quantitative information with the qualitative, emotional, and experiential, I think that’s where the magic is.” - Param Venkataraman (@onwardparam) (21:02)
“I think the coming together of design and data is... a new thing. It’s unprecedented. It’s a bit like how the internet was a new thing back in the mid ’90s. We were all astounded by it, we didn’t know what to do with it, and everybody was just fascinated with it. And we just knew that it’s going to change the world in some way. … Design and data will take some time to mature, and what’s more important is to go into it with an open mind and experiment. And I’m saying this for both designers as well as data scientists, to try and see how the right model might evolve as we experiment and learn.” - Param Venkataraman (@onwardparam) (33:58)
Links Referenced
- Fractal Analytics: https://fractal.ai
- LinkedIn: https://www.linkedin.com/in/parameswaranv/
- Twitter: https://twitter.com/onwardparam
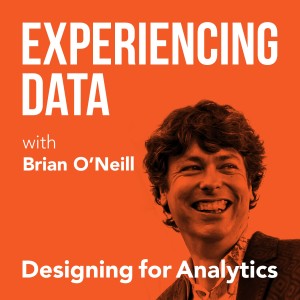
Tuesday Aug 24, 2021
Tuesday Aug 24, 2021
Episode Description
How do you extract the real, unarticulated needs from a stakeholder or user who comes to you asking for AI, a specific app feature, or a dashboard?
On this episode of Experiencing Data, Cindy Dishmey Montgomery, Head of Data Strategy for Global Real Assets at Morgan Stanley, was gracious enough to let me put her on the spot and simulate a conversation between a data product leader and customer.
I played the customer, and she did a great job helping me think differently about what I was asking her to produce for me — so that I would be getting an outcome in the end, and not just an output. We didn’t practice or plan this exercise, it just happened — and she handled it like a pro! I wasn’t surprised; her product and user-first approach told me that she had a lot to share with you, and indeed she did!
A computer scientist by training, Cindy has worked in data, analytics and BI roles at other major companies, such as Revantage, a Blackstone real estate portfolio company, and Goldman Sachs. Cindy was also named one of the 2021 Notable Women on Wall Street by Crain’s New York Business.
Cindy and I also talked about the “T” framework she uses to achieve high-level business goals, as well as the importance for data teams to build trust with end-users.
In our chat, we covered:
- Bringing product management strategies to the creation of data products to build adoption and drive value. (0:56)
- Why the first data hire when building an internal data product should be a senior leader who is comfortable with pushing back. (3:54)
- The "T" Framework: How Cindy, as Head of Data Strategy, Global Real Assets at Morgan Stanley, works to achieve high-level business goals. (8:48)
- How building trust with internal stakeholders by creating valuable and smaller data products is key to eventually working on bigger data projects. (12:38)
- How data's role in business is still not fully understood. (18:17)
- The importance for data teams to understand a stakeholder's business problem and also design a data product solution in collaboration with them. (24:13)
- 'Where's the why': Cindy and Brian roleplay as a data product manager and a customer, respectively, and simulate how to successfully identify a customer’s problem and also open them up to new solutions. (28:01)
- The benefits of a data product management role — and why 'everyone should understand product.' (33:49)
Quotes from Today’s Episode
“There’s just so many good constructs in the product management world that we have not yet really brought very close to the data world. We tend to start with the skill sets, and the tools, and the ML/AI … all the buzzwords. [...]But brass tacks: when you have a happy set of consumers of your data products, you’re creating real value.” - Cindy Dishmey Montgomery (1:55)
“The path to value lies through adoption and adoption lies through giving people something that actually helps them do their work, which means you need to understand what the problem space is, and that may not be written down anywhere because they’re voicing the need as a solution.” - Brian O’Neill (@rhythmspice) (4:07)
“I think our data community tends to over-promise and under-deliver as a way to get the interest, which it’s actually quite successful when you have this notion of, ‘If you build AI, profit will come.’ But that is a really, really hard promise to make and keep.” - Cindy Dishmey Montgomery (12:14)
“[Creating a data product for a stakeholder is] definitely something where you have to be close to the business problem and design it together. … The struggle is making sure organizations know when the right time and what the right first hire is to start that process.” - Cindy Dishmey Montgomery (23:58)
“The temporal aspect of design is something that’s often missing. We talk a lot about the artifacts: the Excel sheet, the dashboard, the thing, and not always about when the thing is used.” - Brian O’Neill (@rhythmspice) (27:27)
“Everyone should understand product. And even just creating the language of product is very helpful in creating a center of gravity for everyone. It’s where we invest time, it’s how it’s meant to connect to a certain piece of value in the business strategy. It’s a really great forcing mechanism to create an environment where everyone thinks in terms of value. And the thing that helps us get to value, that’s the data product.” - Cindy Dishmey Montgomery (34:22)
Links Referenced
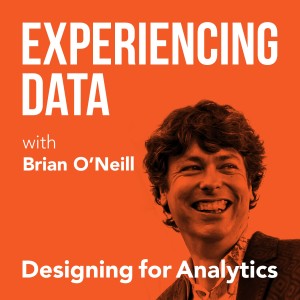
Tuesday Aug 10, 2021
Tuesday Aug 10, 2021
There are many benefits in talking with end users and stakeholders about their needs and pain points before designing a data product.
Just take it from Bill Albert, executive director of the Bentley University User Experience Center, author of Measuring the User Experience, and my guest for this week’s episode of Experiencing Data. With a career spanning more than 20 years in user experience research, design, and strategy, Bill has some great insights on how UX research is pivotal to designing a useful data product, the different types of customer research, and how many users you need to talk to to get useful info.
In our chat, we covered:
- How UX research techniques can help increase adoption of data products. (1:12)
- Conducting 'upfront research': Why talking to end users and stakeholders early on is crucial to designing a more valuable data product. (8:17)
- 'A participatory design process': How data scientists should conduct research with stakeholders before and during the designing of a data product. (14:57)
- How to determine sample sizes in user experience research -- and when to use qualitative vs. quantitative techniques. (17:52)
- How end user research and design improvements helped Boston Children's Hospital drastically increase the number of recurring donations. (24:38)
- How a person's worldview and experiences can shape how they interpret data. (32:38)
- The value of collecting metrics that reflect the success and usage of a data product. (38:11)
Quotes from Today’s Episode
“Teams are constantly putting out dashboards and analytics applications — and now it’s machine learning and AI— and a whole lot of it never gets used because it hits all kinds of human walls in the deployment part.” - Brian (3:39)
“Dare to be simple. It’s important to understand giving [people exactly what they] want, and nothing more. That’s largely a reflection of organizational maturity; making those tough decisions and not throwing out every single possible feature [and] function that somebody might want at some point.” - Bill (7:50)
“As researchers, we need to more deeply understand the user needs and see what we’re not observing in the lab [and what] we can’t see through our analytics. There’s so much more out there that we can be doing to help move the experience forward and improve that in a substantial way.” - Bill (10:15)
“You need to do the upfront research; you need to talk to stakeholders and the end users as early as possible. And we’ve known about this for decades, that you will get way more value and come up with a better design, better product, the earlier you talk to people.” - Bill (13:25)
“Our research methods don’t change because what we’re trying to understand is technology-agnostic. It doesn’t matter whether it’s a toaster or a mobile phone — the questions that we’re trying to understand of how people are using this, how can we make this a better experience, those are constant.” - Bill (30:11)
“I think, what’s called model interpretability sometimes or explainable AI, I am seeing a change in the market in terms of more focus on explainability, less on model accuracy at all costs, which often likes to use advanced techniques like deep learning, which are essentially black box techniques right now. And the cost associated with black box is, ‘I don’t know how you came up with this and I’m really leery to trust it.’” - Brian (31:56)
Resources and Links:
- Bentley University User Experience Center: https://www.bentley.edu/centers/user-experience-center
- Measuring the User Experience: https://www.amazon.com/Measuring-User-Experience-Interactive-Technologies/dp/0124157815
- www.bentley.edu/uxc: https://www.bentley.edu/uxc
- LinkedIn: https://www.linkedin.com/in/walbert/
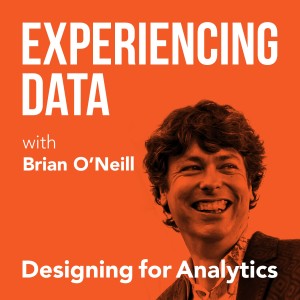
Tuesday Jul 27, 2021
Tuesday Jul 27, 2021
As much as AI has the ability to change the world in very positive ways, it also can be incredibly destructive. Sean McGregor knows this well, as he is currently developing the Partnership on AI’s AI Incident Database, a searchable collection of news articles that covers questionable use, failures, and other incidents that affect people when AI solutions are poorly designed.
On this episode of Experiencing Data, Sean takes us through his notable work around using machine learning in the domain of fire suppression, and how human-centered design is critical to ensuring these decision support solutions are actually used and trusted by the users. We also covered the social implications of new decision-making tools leveraging AI, and:
- Sean's focus on ensuring his models and interfaces were interpretable by users when designing his fire-suppression system and why this was important. (0:51)
- How Sean built his fire suppression model so that different stakeholders can optimize the system for their unique purposes. (8:44)
- The social implications of new decision-making tools. (11:17)
- Tailoring to the needs of 'high-investment' and 'low-investment' people when designing visual analytics. (14:58)
- The AI Incident Database: Preventing future AI deployment harm by collecting and displaying examples of the unintended and negative consequences of AI. (18:20)
- How human-centered design could prevent many incidents of harmful AI deployment — and how it could also fall short. (22:13)
- 'It's worth the time and effort': How taking time to agree on key objectives for a data product with stakeholders can lead to greater adoption. (30:24)
Quotes from Today’s Episode
“As soon as you enter into the decision-making space, you’re really tearing at the social fabric in a way that hasn’t been done before. And that’s where analytics and the systems we’re talking about right now are really critical because that is the middle point that we have to meet in and to find those points of compromise.” - Sean (12:28)
“I think that a lot of times, unfortunately, the assumption [in data science is], ‘Well if you don’t understand it, that’s not my problem. That’s your problem, and you need to learn it.’ But my feeling is, ‘Well, do you want your work to matter or not? Because if no one’s using it, then it effectively doesn’t exist.’” - Brian (17:41)
“[The AI Incident Database is] a collection of largely news articles [about] bad things that have happened from AI [so we can] try and prevent history from repeating itself, and [understand] more of [the] unintended and bad consequences from AI....” - Sean (19:44)
“Human-centered design will prevent a great many of the incidents [of AI deployment harm] that have and are being ingested in the database. It’s not a hundred percent thing. Even in human-centered design, there’s going to be an absence of imagination, or at least an inadequacy of imagination for how these things go wrong because intelligent systems — as they are currently constituted — are just tremendously bad at the open-world, open-set problem.” - Sean (22:21)
“It’s worth the time and effort to work with the people that are going to be the proponents of the system in the organization — the ones that assure adoption — to kind of move them through the wireframes and examples and things that at the end of the engineering effort you believe are going to be possible. … Sometimes you have to know the nature of the data and what inferences can be delivered on the basis of it, but really not jumping into the principal engineering effort until you adopt and agree to what the target is. [This] is incredibly important and very often overlooked.” - Sean (31:36)
“The things that we’re working on in these technological spaces are incredibly impactful, and you are incredibly powerful in the way that you’re influencing the world in a way that has never, on an individual basis, been so true. And please take that responsibility seriously and make the world a better place through your efforts in the development of these systems. This is right at the crucible for that whole process.” - Sean (33:09)
Links Referenced
- seanbmcgregor.com: https://seanbmcgregor.com
- AI Incident Database: https://incidentdatabase.ai
- Partnership on AI: https://www.partnershiponai.org
Twitter: https://twitter.com/seanmcgregor
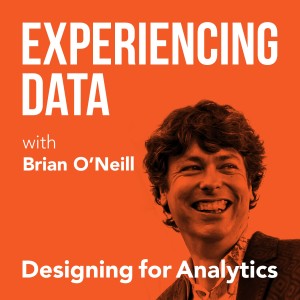
Tuesday Jul 13, 2021
Tuesday Jul 13, 2021
Doug Laney is the preeminent expert in the field of infonomics — and it’s not just because he literally wrote the book on it.
As the Data & Analytics Strategy Innovation Fellow at consulting firm West Monroe, Doug helps businesses use infonomics to measure the economic value of their data and monetize it. He also is a visiting professor at the University of Illinois at Urbana-Champaign where he teaches classes on analytics and infonomics.
On this episode of Experiencing Data, Doug and I talk about his book Infonomics, the many different ways that businesses can monetize data, the role of creativity and product management in producing innovative data products, and the ever-evolving role of the Chief Data Officer.
In our chat, we covered:
- Why Doug's book Infonomics argues that measuring data for its value potential is key to effectively managing and monetizing it. (2:21)
- A 'regenerative asset': Innovative methods for deploying and monetizing data — and the differences between direct, indirect, and inverted data monetization. (5:10)
- The responsibilities of a Chief Data Officer (CDO) — and how taking a product management approach to data can generate additional value. (13:28)
- Why Doug believes that a 'lack of vision and leadership' is partly behind organizational hesitancy of data monetization efforts. (17:10)
- ‘A pretty unique skill’: The importance of bringing in people with experience creating and marketing data products when monetizing data. (19:10)
- Insurance and torrenting: Creative ways companies have leveraged their data to generate additional value. (24:27)
- Ethical data monetization: Why Doug believes consumers must receive a benefit when organizations leverage their data for profit. (27:14)
- The data monetization workshops Doug runs for businesses looking to generate new value streams from its data. (29:42)
Quotes from Today’s Episode
“Many organizations [endure] a vicious cycle of not measuring [their data], and therefore not managing, and therefore not monetizing their data as well as they can. The idea behind my book Infonomics is, flip that. I’ll just start with measuring your data, understanding what you have, its quality characteristics, and its value potential. But vision is important as well, and so that’s where we start with monetization, and thinking more broadly about the ways to generate measurable economic benefits from data.” - Doug (4:13)
“A lot of people will compare data to oil and say that ‘Data is the new oil.’ But you can only use a drop of oil one way at a time. When you consume a drop of oil, it creates heat and energy and pollution, and when you use a drop of oil, it doesn’t generate more oil. Data is very different. It has unique economic qualities that economists would call a non-rivalrous, non-depleting, and regenerative asset.” - Doug (7:52)
“The Chief Data Officer (CDO) role has come on strong in organizations that really want to manage their data as an actual asset, ensure that it is accounted for as generating value and is being managed and controlled effectively. Most CDOs play both offense and defense in controlling and governing data on one side and in enabling it on the other side to drive more business value.”- Doug (14:17)
“The more successful teams that I read about and I see tend to be of a mixed skill set, they’re cross-functional; there’s a space for creativity and learning, there’s a concept of experimentation that’s happening there.” - Brian (19:10)
“Companies that become more data-driven have a market-to-book value that’s nearly two times higher than the market average. And companies that make the bulk of their revenue by selling data products or derivative data have a market-to-book value that’s nearly three times the market average. So, there's a really compelling reason to do this. It’s just that not a lot of executives are really comfortable with it. Data continues to be something that’s really amorphous and they don’t really have their heads around.” - Doug (21:38)
“There’s got to be a benefit to the consumer in the way that you use their data. And that benefit has to be clear, and defined, and ideally measured for them, that we’re able to reduce the price of this product that you use because we’re able to share your data, even if it’s anonymously; this reduces the price of your product.” - Doug (28:24)
Links referenced
- Infonomics: https://www.amazon.com/Infonomics-Monetize-Information-Competitive-Advantage/dp/1138090387
- Email: dlaney@westmonroe.com
- LinkedIn: https://www.linkedin.com/in/douglaney/
- Westmonroe.com: https://westmonroe.com
- Coursera: https://www.coursera.org/instructor/dblaney
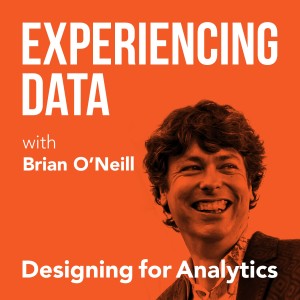
Tuesday Jun 29, 2021
Tuesday Jun 29, 2021
Drew Smith knows how much value data analytics can add to a business when done right.
Having worked at the IKEA Group for 17 years, Drew helped the company become more data-driven, implementing successful strategies for data analytics and governance across multiple areas of the company.
Now, Drew serves as the Executive Vice President of the Analytics Leadership Consortium at the International Institute for Analytics, where he helps Fortune 1000 companies successfully leverage analytics and data science.
On this episode of Experiencing Data, Drew and I talk a lot about the factors contributing to low adoption rates of data products, how product and design-thinking approaches can help, and the value of proper one-on-one research with customers.
In our chat, we covered:
- 'It’s bad and getting worse': Drew's take on the factors behind low adoption of data products. (1:08)
- Decentralizing data analytics: How understanding a user's business problems by including them in the design process can lead to increased adoption of data products. (6:22)
- The importance for business leaders to have a conceptual understanding of the algorithms used in decision-making data products. (14:43)
- Why data analysts need to focus more on providing business value with the models they create. (18:14)
- Looking for restless curiosity in new hires for data teams — and the importance of nurturing new learning through training. (22:19)
- The value of spending one-on-one time with end-users to research their decision-making process before creating a data product. (27:00)
- User-informed data products: The benefits of design and product-thinking approaches when creating data analytics solutions. (33:04)
- How Drew's view of data analytics has changed over 15 years in the field . (45:34)
Quotes from Today’s Episode
“I think as we [decentralize analytics back to functional areas] — as firms keep all of the good parts of centralizing, and pitch out the stuff that doesn’t work — I think we’ll start to see some changes [when it comes to the adoption of data products.]” - Drew (10:07)
“I think data people need to accept that the outcome is not the model — the outcome is a business performance which is measurable, material, and worth the change.” - Drew (21:52)
“We talk about the concept of outcomes over outputs a lot on this podcast, and it’s really about understanding what is the downstream [effect] that emerges from the thing I made. Nobody really wants the thing you made; they just want the result of the thing you made. We have to explore what that is earlier in the process — and asking, “Why?” is very important.” - Brian (22:21)
“I have often said that my favorite people in the room, wherever I am, aren’t the smartest, it’s the most curious.” - Drew (23:55)
“For engineers and people that make things, it’s a lot more fun to make stuff that gets used. Just at the simplest level, the fact that someone cared and it didn’t just get shelved, and especially when you spent half your year on this thing, and your performance review is tied to it, it’s just more enjoyable to work on it when someone’s happy with the outcome.” - Brian (33:04)
“Product thinking starts with the assumption that ‘this is a good product,’ it’s usable and it’s making our business better, but it’s not finished. It’s a continuous loop. It’s feeding back in data through its exhaust. The user is using it — maybe even in ways I didn’t imagine — and those ways are better than I imagined, or worse than I imagined, or different than I imagined, but they inform the product.” - Drew (36:35)
Links Referenced
- Email: dsmith@iiaanalytics.com
- Company site: https://iiaanalytics.com
- LinkedIn: https://www.linkedin.com/in/andrewjsmithknownasdrew/
Analytics Leadership Consortium: https://iianalytics.com/services/analytics-leadership-consortium
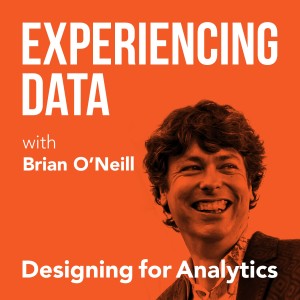
Tuesday Jun 15, 2021
Tuesday Jun 15, 2021
On today’s episode of Experiencing Data, I’m so excited to have Omar Khawaja on to talk about how his team is integrating user-centered design into data science, BI and analytics at Roche Diagnostics.
In this episode, Omar and I have a great discussion about techniques for creating more user-centered data products that produce value — as well as how taking such an approach can lead to needed change management on how data is used and interpreted.
In our chat, we covered:
- What Omar is responsible for in his role as Head of BI & Analytics at Roche Diagnostics — and why a human-centered design approach to data analytics is important to him. (0:57)
- Understanding the end-user's needs: Techniques for creating more user-centric products — and the challenges of taking on such an approach. (6:10)
- Dissecting 'data culture': Why Omar believes greater implementation of data-driven decision-making begins with IT 'demonstrating' the approach's benefits. (9:31)
- Understanding user personas: How Roche is delivering better outcomes for medical patients by bringing analytical insights to life. (15:19)
- How human-centered design yields early 'actionable insights' that can lead to needed change management on how data is used and interpreted. (22:12)
- The journey of learning: Why 'it's everybody's job' to be focused on user experience — and how field research can help determine an end-users needs. (27:26)
- Omar's love of cricket and the statistics collected about the sport! (31:23)
Resources and Links:
- Roche Diagnostics: https://www.roche.com/
- LinkedIn: https://www.linkedin.com/in/kmaomar/
- Twitter: https://twitter.com/kmaomar
Quotes from Today’s Episode
“I’ve been in the area of data and analytics since two decades ago, and out of my own learning — and I’ve learned it the hard way — at the end of the day, whether we are doing these projects or products, they have to be used by the people. The human factor naturally comes in.” - Omar (2:27)
“Especially when we’re talking about enterprise software, and some of these more complex solutions, we don’t really want people noticing the design to begin with. We just want it to feel valuable, and intuitive, and useful right out of the box, right from the start.” - Brian (4:08)
“When we are doing interviews with [end-users] as part of the whole user experience [process], you learn to understand what’s being said in between the lines, and then you learn how to ask the right questions. Those exploratory questions really help you understand: What is the real need?” - Omar (8:46)
“People are talking about data-driven [cultures], data-informed [cultures] — but at the end of the day, it has to start by demonstrating what change we want. ... Can we practice what we are trying to preach? Am I demonstrating that with my team when I’m making decisions in my day-to-day life? How do I use the data? IT is very good at asking our business colleagues and sometimes fellow IT colleagues to use various enterprise IT and business tools. Are we using, ourselves, those tools nicely?” - Omar (11:33)
“We focus a lot on what’s technically possible, but to me, there’s often a gap between the human need and what the data can actually support. And the bigger that gap is, the less chance things get used. The more we can try to close that gap when we get into the implementation stage, the more successful we probably will be with getting people to care and to actually use these solutions.” - Brian (22:20)
“When we are working in the area of data and analytics, I think it’s super important to know how this data and insights will be used — which requires an element of putting yourself in the user’s shoes. In the case of an enterprise setup, it’s important for me to understand the end-user in different roles and personas: What they are doing and how their job is. [This involves] sitting with them, visiting them, visiting the labs, visiting the factory floors, sitting with the finance team, and learning what they do in the system. These are the places where you have your learning.” - Omar (29:09)