
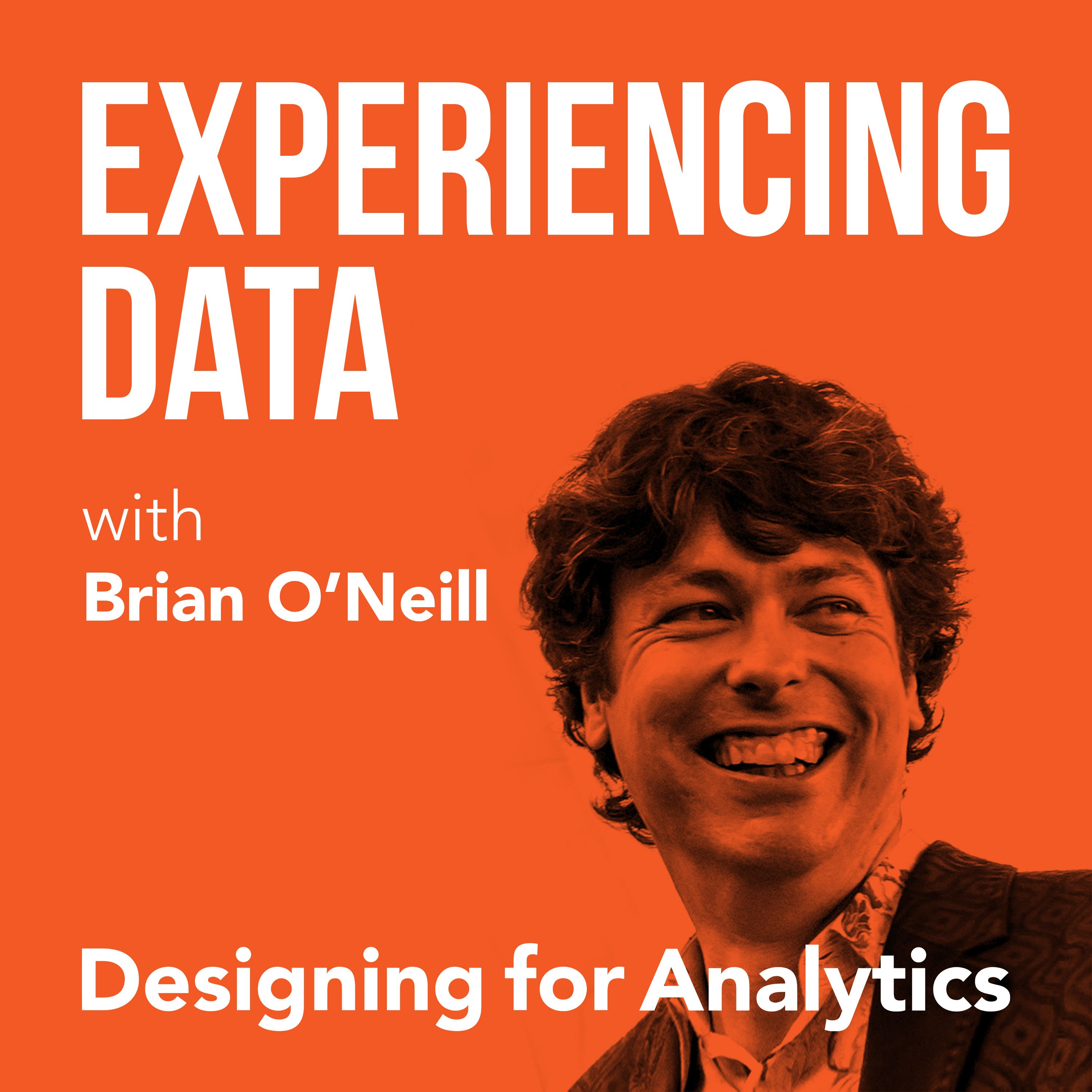
134.4K
Downloads
167
Episodes
Is the value of your enterprise analytics SAAS or AI product not obvious through it’s UI/UX? Got the data and ML models right...but user adoption of your dashboards and UI isn’t what you hoped it would be? While it is easier than ever to create AI and analytics solutions from a technology perspective, do you find as a founder or product leader that getting users to use and buyers to buy seems harder than it should be? If you lead an internal enterprise data team, have you heard that a ”data product” approach can help—but you’re concerned it’s all hype? My name is Brian T. O’Neill, and on Experiencing Data—one of the top 2% of podcasts in the world—I share the stories of leaders who are leveraging product and UX design to make SAAS analytics, AI applications, and internal data products indispensable to their customers. After all, you can’t create business value with data if the humans in the loop can’t or won’t use your solutions. Every 2 weeks, I release interviews with experts and impressive people I’ve met who are doing interesting work at the intersection of enterprise software product management, UX design, AI and analytics—work that you need to hear about and from whom I hope you can borrow strategies. I also occasionally record solo episodes on applying UI/UX design strategies to data products—so you and your team can unlock financial value by making your users’ and customers’ lives better. Hashtag: #ExperiencingData. JOIN MY INSIGHTS LIST FOR 1-PAGE EPISODE SUMMARIES, TRANSCRIPTS, AND FREE UX STRATEGY TIPS https://designingforanalytics.com/ed ABOUT THE HOST, BRIAN T. O’NEILL: https://designingforanalytics.com/bio/
Episodes
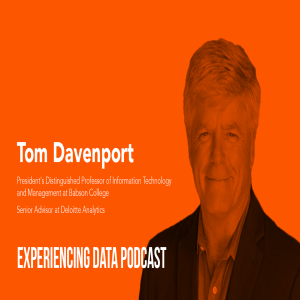
Tuesday Nov 19, 2019
Tuesday Nov 19, 2019
Tom Davenport has literally written the book on analytics. Actually, several of them, to be precise. Over the course of his career, Tom has established himself as the authority on analytics and how their role in the modern organization has evolved in recent years. Tom is a distinguished professor at Babson College, a research fellow at the MIT Initiative on the Digital Economy, and a senior advisor at Deloitte Analytics. The discussion was timely as Tom had just written an article about a financial services company that had trained its employees on human-centered design so that they could ensure any use of AI would be customer-driven and valuable. We discussed their journey and:
- Why on a scale of 1-10, the field of analytics has only gone from a one to about a two in ten years time
- Why so few analytics projects actually make it into production
- Examples of companies who are using design to turn data into useful applications of AI, decision support and product improvements for customers
- Why shadow IT shouldn’t be a bad word
- AI moonshot projects vs. MVPs and how they relate
- Why journey mapping is incredibly useful and important in analytics and data science work
- How human-centered design and ethnography is the tough work that’s required to turn data into decision support
- Tom’s new book and his thoughts on the future of data science and analytics
Resources and Links:
- Website: Tomdavenport.com
- LinkedIn: Tom Davenport
- Twitter: @tdav
- Designingforanalytics.com/seminar
- Designingforanalytics.com
Quotes from Today’s Episode
“If you survey organizations and ask them, ‘Does your company have a data-driven culture?’ they almost always say no. Surveys even show a kind of negative movement over recent years in that regard. And it's because nobody really addresses that issue. They only address the technology side.” — Tom Eventually, I think some fraction of [AI and analytics solutions] get used and are moderately effective, but there is not nearly enough focus on this. A lot of analytics people think their job is to create models, and whether anybody uses it or not is not their responsibility...We don't have enough people who make it their jobs to do that sort of thing. —Tom I think we need this new specialist, like a data ethnographer, who could sort of understand much more how people interact with data and applications, and how many ways they get screwed up.—Tom I don't know how you inculcate it or teach it in schools, but I think we all need curiosity about how technology can make us work more effectively. It clearly takes some investment, and time, and effort to do it.— Tom TD Wealth’s goal was to get [its employees] to experientially understand what data, analytics, technology, and AI are all about, and then to think a lot about how it related to their customers. So they had a lot of time spent with customers, understanding what their needs were to make that match with AI. [...] Most organizations only address the technology and the data sides, so I thought this was very refreshing.—Tom “So we all want to do stuff with data. But as you know, there are a lot of poor solutions that get provided from technical people back to business stakeholders. Sometimes they fall on deaf ears. They don't get used.” — Brian “I actually had a consultant I was talking to recently who said you know the average VP/director or CDO/CAO has about two years now to show results, and this gravy train may be slowing down a little bit.“ — Brian “One of the things that I see in the kind of the data science and analytics community is almost this expectation that ‘I will be handed a well-crafted and well-defined problem that is a data problem, and then I will go off and solve it using my technical skills, and then provide you with an answer.’” — Brian
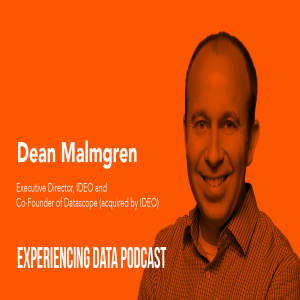
Tuesday Nov 05, 2019
025 - Treating Data Science at IDEO as a Discipline of Design with Dean Malmgren
Tuesday Nov 05, 2019
Tuesday Nov 05, 2019
Dean Malmgren cut his teeth as a chemical and biological engineer. In grad school, he studied complex systems and began telling stories about them through the lens of data algorithms. That led him to co-found Datascope Analytics, a data science consulting company which was purchased by IDEO, a global design firm. Today, Dean is an executive director at IDEO and helps teams use data to build delightful products and experiences.
Join Dean and I as we explore the intersection of data science and design and discuss:
- Human-centered design and why it’s important to data science
- What it was like for a data science company to get ingested into a design firm and why it’s a perfect match
- Why data science isn’t always good at creating things that have never existed before
- Why teams need to prototype rapidly and why data scientists should hesitate to always use the latest tools
- What data scientists can learn from design team and vice-versa
- Why data scientists need to talk to end users early and often, and the importance of developing empathy
- The difference between data scientists and algorithm designers
- Dean’s opinions on why many data analytics projects fail
Resources and Links
Quotes from Today’s Episode
“One of the things that we learned very, very quickly, and very early on, was that designing algorithms that are useful for people involves a lot more than just data and code.” — Dean
“In the projects that we do at IDEO, we are designing new things that don’t yet exist in the world. Designing things that are new to the world is pretty different than optimizing existing processes or business units or operations, which tends to be the focus of a lot of data science teams.” — Dean
“The reality is that designing new-to-the-world things often involves a different mindset than optimizing the existing things.” — Dean
“You know if somebody rates a movie incorrectly, it’s not like you’d throw out Siskel and Ebert’s recommendations for the rest of your life. You just might not pay as much attention to them. But that’s very different when it comes to algorithmic recommendations. We have a lot less tolerance for machines making mistakes.” — Dean
“The key benefit here is the culture that design brings in terms of creating early and getting feedback early in the process, as opposed to waiting you know three, five, six, seven months working on some model, getting it 97% accurate but 10% utilized.” — Brian
“You can do all the best work in the world. But at the end of the day, if there’s a human in the loop, it’s that last mile or last hundred feet, whatever you want to call it, where you make it or break it.” — Brian
“Study after study shows that 10 to 20% of big data analytics projects and AI projects succeed. I’ve actually been collecting them as a hobby in a single article, because they keep coming out.” — Brian
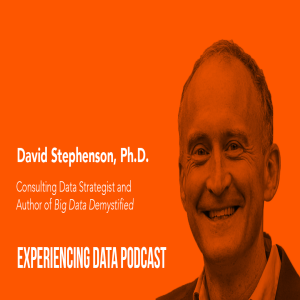
Tuesday Oct 22, 2019
Tuesday Oct 22, 2019
David Stephenson, Ph.D., is the author of Big Data Demystified, a guide for executives that explores the transformative nature of big data and data analytics. He’s also a data strategy consultant and professor at the University of Amsterdam. In a previous life, David worked in various data science roles at companies like Adidas, Coolblue, and eBay.
Join David and I as we discuss what makes data science projects succeed and explore:
- The non-technical issues that lead to ineffective data science and analytics projects
- The specific type of communication that is critical to the success of data science and analytics initiatives (and how working in isolation from your stakeholder or business sponsor creates risk))
- The power of showing value early, starting small/lean, and one way David applies agile to data science projects
- The problems that emerge when data scientists only want to do “interesting data science”
- How design thinking can help data scientists and analytics practitioners make their work resonate with stakeholders who are not “data people”
- How David now relies on design thinking heavily, and what it taught him about making “cool” prototypes nobody cared about
- What it’s like to work on a project without understanding who’s sponsoring it
Resources and Links
Connect with David on LinkedIn
David’s book: Big Data Demystified
Quotes from Today’s Episode
“You see a lot of solutions being developed very well, which were not designed to meet the actual challenge that the industry is facing.” — David
“You just have that whole wasted effort because there wasn’t enough communication at inception.” — David
“I think that companies are really embracing agile, especially in the last few years. They’re really recognizing the value of it from a software perspective. But it’s really challenging from the analytics perspective—partly because the data science and analytics. They don’t fit into the scrum model very well for a variety of reasons.” — David
“That for me was a real learning point—to understand the hardest thing is not necessarily the most important thing.” — David
“If you’re working with marketing people, an 80% solution is fine. If you’re working with finance, they really need exact numbers. You have to understand what your target audience needs in terms of precision.” — David
“I feel sometimes that when we talk about “the business” people don’t understand that the business is a collection of people—just like a government is a collection of real humans doing jobs and they have goals and needs and selfish interests. So there’s really a collection of end customers and the person that’s paying for the solution.” — Brian
“I think it’s always important—whether you’re a consultant or you’re internal—to really understand who’s going to be evaluating the value creation.”— Brian
“You’ve got to keep those lines of communication open and make sure they’re seeing the work you’re doing and evaluating and giving feedback on it. Throw this over the wall is a very high risk model.” — Brian
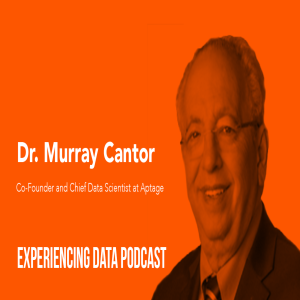
Tuesday Oct 08, 2019
Tuesday Oct 08, 2019
Dr. Murray Cantor has a storied career that spans decades. Recently, he founded Aptage, a company that provides project risk management tools using Bayesian Estimation and machine learning. He’s also the chief scientist at Hail Sports, which focuses on applying precision medicine techniques to sports performance. In his spare time, he’s a consulting mathematician at Pattern Computer, a firm that engineers state-of-the-art pattern recognition solutions for industrial customers.
Join Murray and I as we explore the cutting edge of AI and cover:
- Murray’s approach to automating processes that humans typically do, the role humans have in the design phase, and how he thinks about designing affordances for human intervention in automated systems
- Murray’s opinion on causal modeling (explainability/interpretability), the true stage we are in with XAI, and what’s next for causality in AI models
- Murray’s opinions about the 737 Max’s automated trim control system interface (or lack thereof) and how it should have been designed The favorite method Murray has for predicting outcomes from small data sets
- The major skill gaps Murray sees with young data scientists in particular
- How using science fiction stories can stimulate creative thinking and help kick off an AI initiative successfully with clients, customers and stakeholders
Resources and Links
New York Times Expose article on the Boeing 737 Max
New Your Times Article on the 737 Max whistleblower
Quotes from Today’s Episode
“We’re in that stage of this industrial revolution we’re going through with augmenting people’s ability with machine learning. Right now it’s more of a craft than a science. We have people out there who are really good at working with these techniques and algorithms. But they don’t necessarily understand they’re essentially a solution looking for a problem.” — Murray
“A lot of design principles are the same whether or not you have AI. AI just raises the stakes.” — Murray
“The big companies right now are jumping the guns and saying they have explainable AI when they don’t. It’s going to take a while to really get there.” — Murray
“Sometimes, it’s not always understood by non-designers, but you’re not testing the people. You’re actually testing the system. In fact, sometimes they tell you to avoid using the word test when you’re talking to a participant, and you tell them it’s a study to evaluate a piece of software, or in this case a cockpit, to figure out if it’s the right design or not. It’s so that they don’t feel like they’re a rat in the maze. In reality, we’re studying the maze.” — Brian
“Really fundamental to understanding user experience and design is to ask the question, who is the population of people who are going to use this and what is their range of capability?” – Murray
“Take the implementation hats off and come up with a moonshot vision. From the moonshot, you might find out there are these little tangents that are actually feasible increments. If you never let yourself dream big, you’ll never hit the small incremental steps that you may be able to take.” — Brian
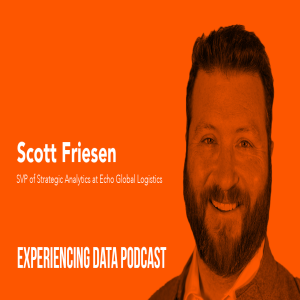
Tuesday Sep 24, 2019
Tuesday Sep 24, 2019
Scott Friesen’s transformation into a data analytics professional wasn’t exactly linear. After graduating with a biology degree and becoming a pre-med student, he switched gears and managed artists in the music industry. After that, he worked at Best Buy, eventually becoming their Senior Director of Analytics for the company’s consumer insights unit. Today, Scott is the SVP of Strategic Analytics at Echo Global Logistics, a provider of technology-enabled transportation and supply chain management services. He also advises for the International Institute for Analytics.
In this episode, Scott shares what he thinks data scientists and analytics leaders need to do to become a trustworthy and indispensable part of an organization. Scott and I both believe that designing good decision support applications and creating useful data science solutions involve a lot more than technical knowledge. We cover:
- Scott’s trust equation, why it’s critical for analytics professionals, and how he uses it to push transformation across the organization
- Scott’s “jazz” vs “classical” approach to creating solutions
- How to develop intimacy and trust with your business partners (e.g., IT) and executives, and the non-technical skills analytics teams need to develop to be successful
- Scott’s opinion about design thinking and analytics solutions
- How to talk about risk to business stakeholders when deploying data science solutions
- How the success of Scott’s new pricing model was impeded by something that had nothing to do with the data—and how he addressed it
- Scott’s take on the emerging “analytics translator” role
- The two key steps to career success—and volcanos
Resources and Links
Quotes from Today's Episode
“You might think it is more like classical music, but truly great analytics are more like jazz. ” — Scott
“If I'm going to introduce change to an organization, then I'm going to introduce perceived risk. And so the way for me to drive positive change—the way for me to drive adding value to the organizations that I'm a part of—is the ability to create enough credibility and intimacy that I can get away with introducing change that benefits the organization.” — Scott
“I categorize the analytic pursuit into three fundamental activities: The first is to observe, the second is to relate, and the third is to predict. ” — Scott
“It's not enough to just understand the technology part and how to create great models. You can get all that stuff right and still fail in the last mile to deliver value.” — Brian
“I tend to think of this is terms of what you called ‘intimacy.’ I don’t know if you equate that to empathy, which is really understanding the thing you are talking about from the perspective of the other person. When we do UX research, the questions themselves are what form this intimacy. An easy way to do that is by asking open-ended questions that require open-ended answers to get that person to open up to you. ” — Brian
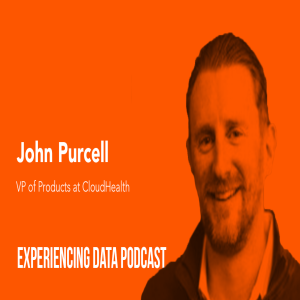
Tuesday Sep 10, 2019
Tuesday Sep 10, 2019
John Purcell has more than 20 years of experience in the technology world. Currently, he’s VP of Products at CloudHealth, a company that helps organizations manage their increasingly complex cloud infrastructure effectively. Prior to this role, he held the same position at SmartBear Software, makers of application performance monitoring solutions. He’s also worn several hats at companies like LogMeIn and Red Bend Software.
In today’s episode, John and I discuss how companies are moving more and more workloads to the cloud and how John and his team at CloudHealth builds a platform that makes it easy for all users—even non-technical ones—to analyze and manage data in the cloud and control their financial spending. In addition to exploring the overall complexity of using analytics to inform the management of cloud environments, we also covered:
- How CloudHealth designs for multiple personas from the financial analyst to the DevOps operator when building solutions into the product
- Why John has “maniacal point of view” and “religion” around design and UX and how they have the power to disrupt a market
- How design helps turn otherwise complex data sets that might require an advanced degree to understand into useful decision support
- How data can lead to action, and how CloudHealth balances automation vs. manual action for its customers using data to make decisions
- Why John believes user experience is a critical voice at the table during the very earliest stages of any new analytics/data initiative
Resources and Links
Twitter: @PurcellOutdoors
Quotes from Today’s Episode
“I think that’s probably where the biggest point of complexity and the biggest point of challenge is for us: trying to make sure that the platform is flexible enough to be able to inject datasets we’ve never seen before and to be able to analyze and find correlations between unknown datasets that we may not have a high degree of familiarity with—so that we can generate insight that’s actionable, but deliver it in a way that’s [easy for anyone to understand].” — John
“My core philosophy is that you need UX at the table early and at every step along the way as you’re contemplating product delivery, and I mean all the way upstream at the strategic phase, at the identification of what you want to go tackle next including product strategy, pain identification, persona awareness, and who are we building for—all the way through solving the problem, what should the product be capable of, and user validation.” — John
“in the cloud, we’re just at the very early stages of [automation based on analytics] from a pure DevOps point of view. We’re still in the world of show me your math. Show me why this is the recommendation you’re making.” — John
“When making decisions using data, some IT people don’t like the system taking action without them being involved because they don’t trust that any product would be smart enough to make all the right decisions, and they don’t want applications going down.” — Brian
“I think the distinction you made between what I would call user interface design, which is the surface layer, buttons, fonts, colors, all that stuff often gets conflated in the world of analytics as being, quote ‘design.’ And as I think our audience is hearing from John here, is that it [design] goes much beyond that. It can get into something like, ‘how do you design a great experience around API documentation? Where’s the demo code? How do I run the demo?’ All of that can definitely be designed as well.” — Brian
“I hear frequently in my conversations with clients and people in the industry that there are a lot of data scientists who just want to use the latest models, and they want to work on model quality and predictive accurateness, etc. But they’re not thinking about how someone is going to use this model to make a decision, and whether will there be some business value created at the end.” — Brian
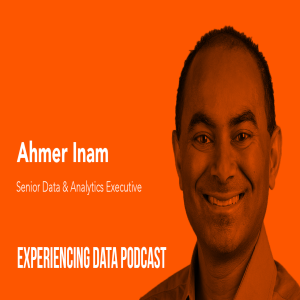
Tuesday Aug 27, 2019
Tuesday Aug 27, 2019
Ahmer Inam considers himself an evangelist of data science who’s been “doing data science since before it was called data science. With more than 20 years of leadership experience in the data science and analytics field at companies including Nike and Cambia health, Ahmer knows a thing or two about what makes data science projects succeed—and what makes them fail.
In today’s episode, Ahmer and I discuss his experiences using design thinking and his “human-centered AI” process to ensure that internal analytics and data science initiatives actually produce usable, useful outputs that turn into business value. Much of this was formed while Ahmer was a Senior Director and Head of Advanced Analytics at Nike, a company that is known as a design-mature organization. We covered:
- The role of empathy in data science teams and how it helps data people connect with non-technical users who may not welcome “yet another IT tool”
- Ahmer’s thoughts on Lean Coaching, Scrum Teams, and getting outside help to accelerate the design and creation of your first data products and predictive models
- The role of change management in the process of moving data products into production
- Ahmer’s two-week process to kick-start data product initiatives used at Nike
- How model accuracy isn’t as important early on as other success metrics when prototyping solutions with customers
Resources and Links
How Analytics Are Informing Change At Nike
Quotes from Today’s Episode
“Build data products with the people, for the people…and bring a sense of vulnerability to the table.” — Ahmer
“What I have seen is that a lot of times we can build models, we can bring the best of the technologies on optimal technology it’s in the platforms, but in the end, if the business process and the people are not ready to take it and use it, that’s where it fails.” — Ahmer
“If we don’t understand people in the process, essentially, the adoption is not going to work. In the end, when it comes to a lot of these data science exercises or projects or development of data products, we have to really think about it as a change management exercise and nothing short of that.” — Ahmer
“Putting humans at the center of these initiatives drives better value and it actually makes sure that these tools and data products that we’re making actually get used, which is what ultimately is going to determine whether or not there’s any business value—because the data itself doesn’t have any value until it’s acted upon.” — Brian
“One of these that’s been stuck in my ear like an earworm is that a lot of the models fail to get to production still. And so this is the ongoing theme of basically large analytics projects, whether you call it big data analytics or AI, it’s the same thing. We’re throwing a lot of money at these problems, and we’re still creating poor solutions that end up not doing anything.” — Brian
“I think the really important point here is that early on with these initiatives, it’s important to figure out, What is going to stop this person from potentially engaging with my service?” — Brian
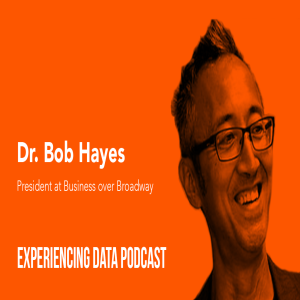
Tuesday Aug 13, 2019
Tuesday Aug 13, 2019
Dr. Bob Hayes, will be the first to tell you that he’s a dataphile. Ever since he took a stats course in college in the 80s, Bob’s been hooked on data. Currently, Bob is the Research and Analytics Director at Indigo Slate. He’s also the president of Business over Broadway, a consultancy he founded in 2007.In a past life, Bob served as Chief Research Officer at Appuri and AnalyticsWeek, Chief Customer Officer at TCELab, and a contributing analyst at Gleanster, among many other roles.
In today’s episode, Bob and I discuss a recent Kaggle survey that highlighted several key non-technical impediments to effective data science projects. In addition to outlining what those challenges are and exploring potential solutions to them, we also covered:
- The three key skills successful data science teams have
- Why improving customer loyalty involves analyzing several metrics, not just one
- Why Bob feels the scientific method is just as important today as it’s been for hundreds of years
- The importance of repeatable results
- How prototyping early can save time and drive adoption of data science projects
- Bob’s advice on how to move data science projects forward (and one key skill he feels most business leaders lack)
- The role of the analytics translator
Resources and Links:
Quotes from Today’s Episode
“I’ve always loved data. I took my first stats course in college over 30 years ago and I was hooked immediately. I love data. Sometimes I introduce myself as a dataholic. I love it.” — Bob
“I’m a big fan of just kind of analyzing data, just getting my hands on data, just exploring it. But that can lead you down a path of no return where you’re just analyzing data just to analyze it. What I try to tell my clients is that when you approach a data set, have a problem that you’re trying to solve. The challenge there I think it stems from the fact that a lot of data science teams don’t have a subject matter expert on the team to pose the right questions.” — Bob
“The three findings that I found pretty interesting were, number one, a lack of a clear question to be answering or a clear direction to go in with the available data. The second one was that data science results were not used by the business decision makers. And the third one was an inability to integrate findings into the organization’s decision making processes.” — Brian
“It makes you wonder,‘if you didn’t have a good problem to solve, maybe that’s why [the findings] didn’t get used in the first place.’” — Brian
“That part isn’t so much the math and the science. That’s more the psychology and knowing how people react. Because you’re going to have certain business stakeholders that still want to kind of shoot from the hip and their experience. Their gut tells them something. And sometimes that gut is really informed.” — Brian
“If executives are looking at data science and AI as a strategic initiative, it seems really funny to me that someone wouldn’t be saying, ‘What do we get out of this? What are the next steps?’ when the data teams get to the end of a project and just moves on to the next one.” — Brian
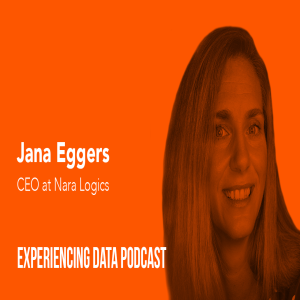
Tuesday Jul 30, 2019
Tuesday Jul 30, 2019
Jana Eggers, a self-proclaimed math and computer nerd, is CEO of Nara Logics, a company that helps organizations use AI to eliminate data silos and unlock the full value of their data, delivering predictive personalized experiences to their customers along the way. The company leverages the latest neuroscience research to model data the same way our brains do. Jana also serves on Fannie Mae’s digital advisory board, which is tasked with finding affordable housing solutions across the United States. Prior to joining Nara Logics, Jana wore many different hats, serving as CEO of Spreadshirt, and General Manager of QuickBase at Intuit, among other positions. She also knows about good restaurants in PDX!
In today’s episode, Jana and I explore her approaches to using AI to help enterprises make interesting and useful predictions that drive better business outcomes and improve customer experience. In addition to discussing how AI can help strengthen personalization and support smarter decision making, we also covered:
- The power of showing the whys when providing predictions (i.e., explainable AI or XAI).
- Jana’s thoughts on why some data scientists struggle with inflated expectations around AI
- Brian’s #facepalm about lipstick and data
- The power of what-if simulations and being able to remove factors from predictions
- The power of context and how Nara Logics weighs recent data vs. long-term historical data in its predictions
- How Nara Logics leverages the wiring of the brain—the connectome—to inspire the models they build and the decision support help they provide to customers
- Why AI initiatives need to consider the “AI trinity”: data, the algorithm, and the results an organization is aiming for
Resources and Links:
Quotes from Today’s Episode
“We have a platform that is really built for decision support. How do you go from having […]20 to having about 500 to 2,000 decision factors coming in? Once we get that overload of information, our tool is used to help people with those decisions. And yes, we’re using a different approach than the traditional neural net, which is what deep learning is based on. While we use that in our tool, we’re more on the cognitive side. […]I’ve got a lot of different signals coming in, how do I understand how those signals relate to each other and then make decisions based on that?” — Jana
“One of the things that we do that also stands us apart is that our AI is transparent—meaning that when we provide an answer, we also give the reasons why that is the right answer for this context. We think it is important to know what was taken into account and what factors weigh more heavily in this context than other contexts.” — Jana
“It is extremely unusual—and I can even say that I’ve never really seen it—that people just say, Okay, I trust the machine. I’m comfortable with that. It knows more than me. That’s really unusual. The only time I’ve seen that is when you’re really doing something new and no one there has any idea what it should be.” — Jana
“With regards to tech answering “why,” I’ve worked on several monitoring and analytics applications in the IT space. When doing root cause analysis, we came up with this idea of referring to monitored objects as being abnormally critical and normally critical. Because at certain times of day, you might be running a backup job and so the IO is going crazy, and maybe the latency is higher. But the IO is supposed to be that way at that time. So how do you knock down that signal and not throw up all the red flags and light up the dashboard when it’s supposed to be operating that way? Answering “why” is difficult. ” — Brian
“We’ve got lipstick, we’ve got kissing. I’m going to get flagged as ‘parental advisory’ on this episode in iTunes probably. ;-)” — Brian
“You can’t just live in the closet and do your math and hope that everyone is going to see the value of it. Anytime we’re building these complex tools and services —what I call human-in-the-loop applications–you’re probably going to have to go engage with other humans, whether it’s customers or your teammates or whatever.” — Brian
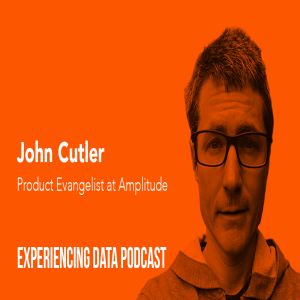
Tuesday Jul 16, 2019
Tuesday Jul 16, 2019
John Cutler is a Product Evangelist for Amplitude, an analytic platform that helps companies better understand users behavior, helping to grow their businesses. John focuses on user experience and evidence-driven product development by mixing and matching various methodologies to help teams deliver lasting outcomes for their customers. As a former UX researcher at AppFolio, a product manager at Zendesk, Pendo.io, AdKeeper and RichFX, a startup founder, and a product team coach, John has a perspective that spans individual roles, domains, and products.
In today’s episode, John and I discuss how productizing storytelling in analytics applications can be a powerful tool for moving analytics beyond vanity metrics. We also covered the importance of understanding customers’ jobs/tasks, involving cross-disciplinary teams when creating a product/service, and:
- John and Amplitude’s North Star strategy and the (3) measurements they care about when tracking their own customers’ success
- Why John loves the concept of analytics “notebooks” (also a particular feature of Amplitude’s product) vs. the standard dashboard method
- Understanding relationships between metrics through “weekly learning users” who share digestible content
- John’s opinions on involving domain experts and cross-discipline teams to enable products focused on outcomes over features
- Recognizing whether your product/app is about explanatory or exploratory analytics
- How Jazz relates to business – how you don’t know what you don’t know yet
Resources and Links:
Quotes from Today’s Episode
“It’s like you know in your heart you should pair with domain experts and people who know the human problem out there and understand the decisions being made. I think organizationally, there’s a lot of organizational inertia that discourages that, unfortunately, and so you need to fight for it. My advice is to fight for it because you know that that’s important and you know that this is not just a pure data science problem or a pure analytics problem. There’s probably there’s a lot of surrounding information that you need to understand to be able to actually help the business.” – John
“We definitely ‘dogfood’ our product and we also ‘dogfood’ the advice we give our customers.” – John
“You know in your heart you should pair with domain experts and people who know the human problem out there and understand the decisions being made. […] there’s a lot of organizational inertia that discourages that, unfortunately, and so you need to fight for it. I guess my advice is, fight for it, because you know that it is important, and you know that this is not just a pure data science problem or a pure analytics problem.” – John
“It’s very easy to create assets and create code and things that look like progress. They mask themselves as progress and improvement, and they may not actually return any business value or customer value explicitly. We have to consciously know what the outcomes are that we want.” – Brian
“We got to get the right bodies in the room that know the right questions to ask. I can smell when the right questions aren’t being asked, and it’s so powerful” – Brian
“Instead of thinking about what are all the right stats to consider, [I sometimes suggest teams] write in plain English, like in prose format, what would be the value that we could possibly show in the data.’ maybe it can’t even technically be achieved today. But expressing the analytics in words like, ‘you should change this knob to seven instead of nine because we found out X, Y, and Z happened. We also think blah, blah, blah, blah, blah, and here is how we know that, and there’s your recommendation.’ This method is highly prescriptive, but it’s an exercise in thinking about the customer’s experience.” – Brian