
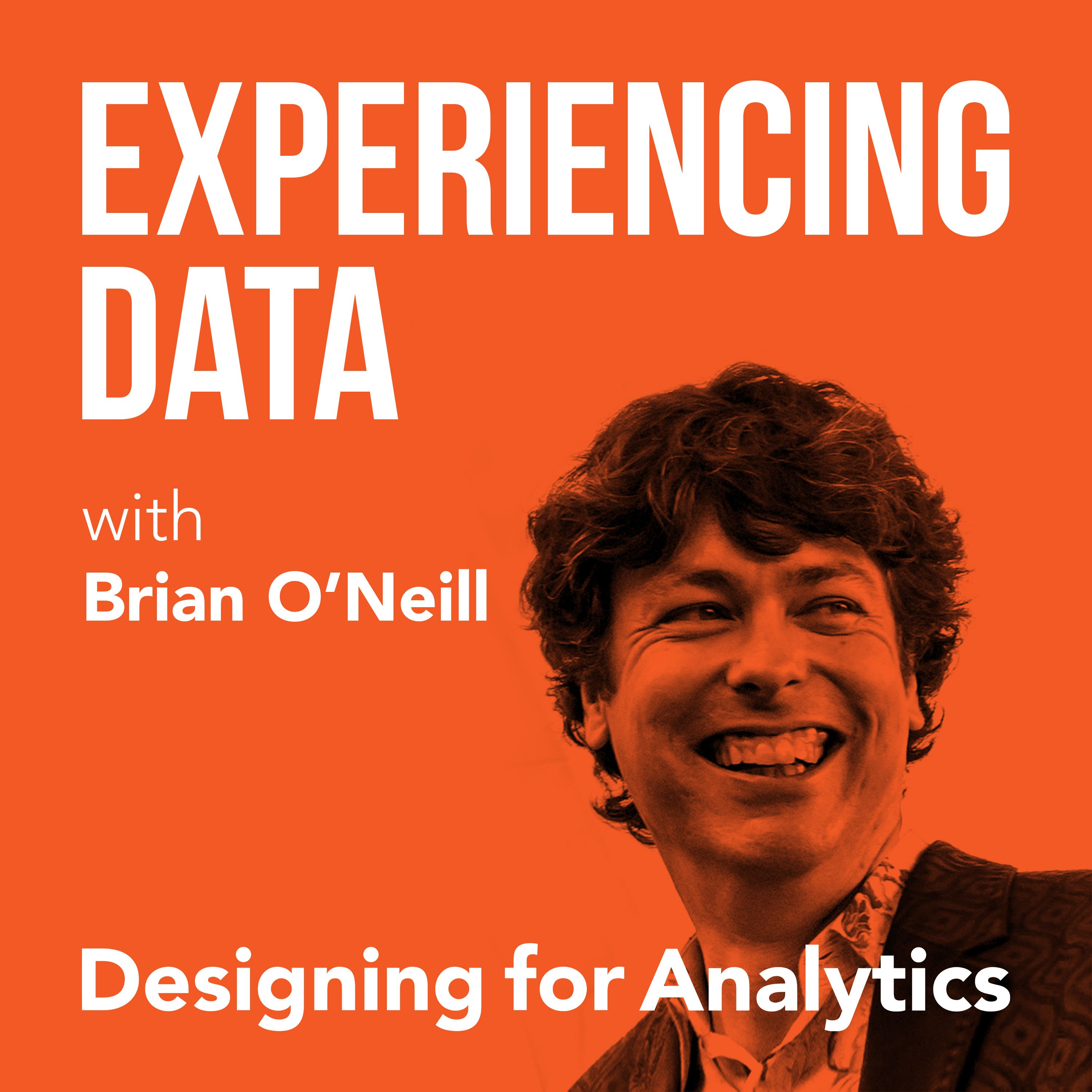
127.9K
Downloads
161
Episodes
If you’re a leader tasked with generating business and org. value through ML/AI and analytics, you’ve probably struggled with low user adoption. Making the tech gets easier, but getting users to use, and buyers to buy, remains difficult—but you’ve heard a ”data product” approach can help. Can it? My name is Brian T. O’Neill, and on Experiencing Data—one of the top 2% of podcasts in the world—I offer you a consulting designer’s perspective on why creating ML and analytics outputs isn’t enough to create business and UX outcomes. How can UX design and product management help you create innovative ML/AI and analytical data products? What exactly are data products—and how can data product management help you increase user adoption of ML/analytics—so that stakeholders can finally see the business value of your data? Every 2 weeks, I answer these questions via solo episodes and interviews with innovative chief data officers, data product management leaders, and top UX professionals. Hashtag: #ExperiencingData. PODCAST HOMEPAGE: Get 1-page summaries, text transcripts, and join my Insights mailing list: https://designingforanalytics.com/ed ABOUT THE HOST, BRIAN T. O’NEILL: https://designingforanalytics.com/bio/
Episodes
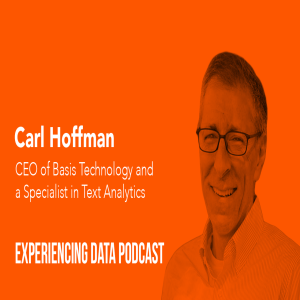
Tuesday Apr 09, 2019
Tuesday Apr 09, 2019
My guest today is Carl Hoffman, the CEO of Basis Technology, and a specialist in text analytics. Carl founded Basis Technology in 1995, and in 1999, the company shipped its first products for website internationalization, enabling Lycos and Google to become the first search engines capable of cataloging the web in both Asian and European languages. In 2003, the company shipped its first Arabic analyzer and began development of a comprehensive text analytics platform. Today, Basis Technology is recognized as the leading provider of components for information retrieval, entity extraction, and entity resolution in many languages. Carl has been directly involved with the company’s activities in support of U.S. national security missions and works closely with analysts in the U.S. intelligence community.
Many of you work all day in the world of analytics: numbers, charts, metrics, data visualization, etc. But, today we’re going to talk about one of the other ingredients in designing good data products: text! As an amateur polyglot myself (I speak decent Portuguese, Spanish, and am attempting to learn Polish), I really enjoyed this discussion with Carl. If you are interested in languages, text analytics, search interfaces, entity resolution, and are curious to learn what any of this has to do with offline events such as the Boston Marathon Bombing, you’re going to enjoy my chat with Carl. We covered:
- How text analytics software is used by Border patrol agencies and its limitations.
- The role of humans in the loop, even with good text analytics in play
- What actually happened in the case of the Boston Marathon Bombing?
- Carl’s article“Exact Match” Isn’t Just Stupid. It’s Deadly.
- The 2 lessons Carl has learned regarding working with native tongue source material.
- Why Carl encourages Unicode Compliance when working with text, why having a global perspective is important, and how Carl actually implements this at his company
- Carl’s parting words on why hybrid architectures are a core foundation to building better data products involving text analytics
Resources and Links:
- Basis Technology
- Carl’s article: “Exact Match” isn’t Just Stupid. It’s Deadly.
- Carl Hoffman on LinkedIn
Quotes from Today’s Episode
“One of the practices that I’ve always liked is actually getting people that aren’t like you, that don’t think like you, in order to intentionally tease out what you don’t know. You know that you’re not going to look at the problem the same way they do…” — Brian O’Neill
“Bias is incredibly important in any system that tries to respond to human behavior. We have our own innate cultural biases that we’re sometimes not even aware of. As you [Brian] point out, it’s impossible to separate human language from the underlying culture and, in some cases, geography and the lifestyle of the people who speak that language…” — Carl Hoffman
“What I can tell you is that context and nuance are equally important in both spoken and written human communication…Capturing all of the context means that you can do a much better job of the analytics.” — Carl Hoffman
“It’s sad when you have these gaps like what happened in this border crossing case where a name spelling is responsible for not flagging down [the right] people. I mean, we put people on the moon and we get something like a name spelling [entity resolution] wrong. It’s shocking in a way.” — Brian O’Neill
“We live in a world which is constantly shades of gray and the challenge is getting as close to yes or no as we can.”– Carl Hoffman
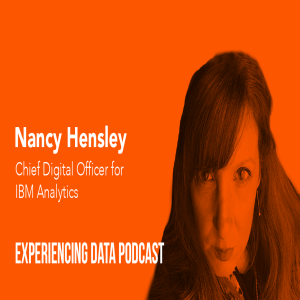
Tuesday Mar 26, 2019
Tuesday Mar 26, 2019
Nancy Hensley is the Chief Digital Officer for IBM Analytics, a multi-billion dollar IBM software business focused on helping customers transform their companies with data science and analytics. Nancy has over 20 years of experience working in the data business in many facets from development, product management, sales, and marketing.
Today’s episode is probably going to appeal to those of you in product management or working on SAAS/cloud analytics tools. It is a bit different than our previous episodes in that we focused a lot on what “big blue” is doing to simplify its analytics suite as well as facilitating access to those tools. IBM has many different analytics-related products and they rely on good design to make sure that there is a consistent feel and experience across the suite, whether it’s Watson, statistics, or modeling tools. She also talked about how central user experience is to making IBM’s tools more cloud-like (try/buy online) vs. forcing customers to go through a traditional enterprise salesperson.
If you’ve got a “dated” analytics product or service that is hard to use or feels very “enterprisey” (in that not-so-good way), then I think you’ll enjoy the “modernization” theme of this episode. We covered:
- How Nancy is taking a 50-year old product such as SPSS and making it relevant and accessible for an audience that is 60% under 25 years of age
- The two components Nancy’s team looks at when designing an analytics product
- What “Metrics Monday” is all about at IBM Analytics
- How IBM follows-up with customers, communicates with legacy users, and how the digital market has changed consumption models
- Nancy’s thoughts on growth hacking and the role of simplification
- Why you should always consider product-market fit first and Nancy’s ideas on MVPs
- The role design plays in successful onboarding customers into IBM Analytics’ tools and what Brian refers to as the “honeymoon” experience
Resources and Links:
Quotes:
“It’s really never about whether it’s a great product. It’s about whether the client thinks it’s great when they start using it.” –Nancy
“Every time we add to the tool, we’re effectively reducing the simplicity of everything else around it.”–Brian
“The design part of it for us is so eye-opening, because again, we’ve built a lot of best in class enterprise products for years and as we shift into this digital go-to-market, it is all about the experience…”–Nancy
“Filling in that “why” piece is really important if you’re going to start changing design because you may not really understand the reasons someone’s abandoning.”–Brian
“Because a lot of our products weren’t born in the cloud originally, they weren’t born to be digitally originally, doesn’t mean they can’t be digitally consumed. We just have to really focus on the experience and one of those things is onboarding.” –Nancy
“If they [users] can’t figure out how to jump in and use the product, we’re not nailing it. It doesn’t matter how great the product is, if they can’t figure out how to effectively interact with it. –Nancy
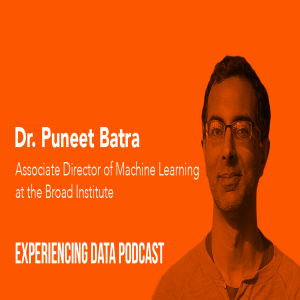
Tuesday Mar 12, 2019
Tuesday Mar 12, 2019
Dr. Puneet Batra is the Associate Director of Machine Learning at the Broad Institute, where his team builds machine learning algorithms and pipelines to help discover new biological insights and impact disease. Puneet has spent his career stitching together data-driven solutions: in location data analysis, as cofounder of LevelTrigger; in health care, as Chief Data Scientist at Kyruus; as lead Analytic Scientist at Aster Data (Acq by Teradata); and in fundamental models of particle physics, developing theories for Fermilab’s Tevatron and CERN’s Large Hadron Collider. He has held research positions at Harvard, Stanford and Columbia Universities. Puneet completed his BA at Harvard University and has a Ph.D. from Stanford University.
A friend of mine introduced me to Puneet because he was kicking off a side project using machine learning to dig into what creativity is through the lens of jazz. Since Puneet is not a musician by training, he was looking for some domain-specific knowledge to inform his experiment, and I really liked his design-oriented thinking. While jazz kicks off our conversation, we went a lot more deeply into the contemporary role of the data scientist in this episode including:
- The discussions that need to happen between users, stakeholders, and subject matter experts so teams get a clear image of the problem that actually needs to be solved.
- Dealing with situations where the question you start with isn’t always the question that is answered in the end.
- When to sacrifice model quality for the sake of user experience and higher user engagement (i.e. the “good enough” approach)
- The role of a data scientist in product design.
Resources and Links:
Quotes from Puneet Batra
"Sometimes, accuracy isn't the most important thing you should be optimizing for; it’s the rest of the package…if you can make that a good process, then I think you're more on the road to making users happy [vs.] trying to live in this idealized world where you never make a mistake at all."
"The question you think you're answering from the beginning probably isn't the one that you're going to stay answering the entire time and you've just got to be flexible around that."
"Even data scientists and engineers should be able to listen with empathy and ask questions. I got a good number of tips from people teaching me how to do things like that. Basically, we ask a question or basically shut up and hear what their answer is."
"I'm not really sure what creativity is. I'm not really sure if machines will ever be creative. A good experiment to try to prove that out is to try to get a machine to be as creative as possible and see where it falls flat."

Tuesday Feb 26, 2019
Tuesday Feb 26, 2019
Jim Psota is the Co-Founder and CTO of Panjiva, which was named one of the top 10 Most Innovative Data Science Companies in the World by Fast Company in 2018. Panjiva has mapped the global supply chain using a combination of over 1B shipping transactions and machine learning, and recently the company was acquired by S&P Global.
Jim has spoken about artificial intelligence and entrepreneurship at Harvard Business School, MIT, and The White House, and at numerous academic and industry conferences. He also serves on the World Economic Forum’s Working Group for Artificial Intelligence and has done Ph.D. research in computer science at MIT. Some of the topics we discuss in today’s episode include:
- What Jim learned from starting Panjiva from a data-first approach
- Brian and Jim’s thoughts on problem solving driven by use cases and people vs. data and AI
- 3 things Jim wants teams to get right with data products
- Jim and Brian’s thoughts on “blackbox” analytics that try to mask complex underlying data to make the UX easier
- How Jim balances the messiness of 20+ third-party data sources, designing a good product, and billions of data points
Resources and Links:
Quotes from Jim Psota
“When you’re dealing with essentially resolving 1.5 billion records, you could think of that you need to compute 1.5 billion squared pairs of potential similarities.”
“It’s much more fulfilling to be building for a person or a set of people that you’ve actually talked to… The engineers are going to develop a much better product and frankly be much happier having that connection to the user.”
“We have crossed a pretty cool threshold where a lot of value can be created now because we have this nice combination of data availability, strong algorithms, and compute power.”
“In our case and many other company’s cases, taking third-party data, no matter where you’re getting your data, there’s going to be issues with it, there’s going to be delays, format changes, granularity differences.”
“As much as possible, we try to use the tools of data science to actually correct the data deficiency or impute or whatever technique is actually going to be better than nothing, but then say this was imputed or this is reported versus imputed…then over time, the user starts to understand if it’s gray italics [the data] was imputed, and if it’s black regular text, that’s reported data, for example.”
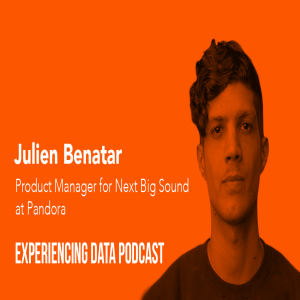
Wednesday Feb 13, 2019
Wednesday Feb 13, 2019
We’re back with a special music-related analytics episode! Following Next Big Sound’s acquisition by Pandora, Julien Benatar moved from engineering into product management and is now responsible for the company’s analytics applications in the Creator Tools division. He and his team of engineers, data scientists and designers provide insights on how artists are performing on Pandora and how they can effectively grow their audience. This was a particularly fun interview for me since I have music playing on Pandora and occasionally use Next Big Sound’s analytics myself. Julien and I discussed:
- How Julien’s team accounts for designing for a huge range of customers (artists) that have wildly different popularity, song plays, and followers
- How the service generates benchmark values in order to make analytics more useful to artists
- How email notifications can be useful or counter-productive in analytics services
How Julien thinks about the Data Pyramid when building out their platform - Having a “North Star” and driving analytics toward customer action
- The types of predictive analytics Next Big Sound is doing
Resources and Links:
Quotes from Julien Benatar
"I really hope we get to a point where people don’t need to be data analysts to look at data."
"People don’t just want to look at numbers anymore, they want to be able to use numbers to make decisions."
"One of our goals was to basically check every artist in the world and give them access to these tools and by checking millions of artists, it allows us to do some very good and very specific benchmarks"
“The way it works is you can thumb up or thumb down songs. If you thumb up a song, you’re giving us a signal that this is something that you like and something you want to listen to more. That’s data that we give back to artists.”
“I think the great thing today is that, compared to when Next Big Sound started in 2009, we don’t need to make a point for people to care about data. Everyone cares about data today.”
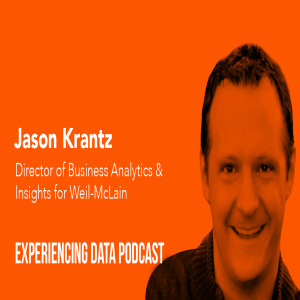
Tuesday Jan 29, 2019
Tuesday Jan 29, 2019
Jason Krantz is the Director of Business Analytics & Insights for the 135-year old company, Weil McLain and Marley Engineered Products. While the company is responsible for helping keeping homes and businesses warm, Jason is responsible for the creation and growth of analytical capabilities at Weil McLain, and was recognized in 2017 as a “Top 40 Under 40” in the HVAC industry. I’m not surprised given his posts on LinkedIn; Jason seems very focused on satisfying his internal customers and ensuring that there is practical business value anchoring their analytics initiatives. We talked about:
- How Jason’s team keeps their data accessible and relevant to the issue they need to solve for their customer.
- How Jason strives to keep the information simple and clean for the customer.
- How does Jason help drive analytics in a company culture with a lot of legacy (from its people to its parts)
- The importance of focusing on context
- How Jason drives his team to be business partners, and not report generators
Resources and Links:
Quotes from Jason Krantz:
“You realize that small quick wins are very effective because, at its core, it’s really important to get executive buy-in.”
“I’m a huge fan of simplicity. As analytics pros, we could very easily make very complex, very intricate models, and just, ‘Oh, look at how smart we are.’ It doesn’t help our customers. …we only use about two or three different visual types and we use mostly the exact same visual set-up. I can train a sales rep for probably five minutes on all of our reporting because if you understand one, you’re going to understand everything. That gets to the theme again of just simplicity. Don’t over complicate, keep it simple, keep it clean.”
“…To get buy-in, you really got to have your business case, even to your internal customers, really dialed in. If you just bring them a bunch of crap, that’s how you’re going to lose credibility. They’re going to be like, “I don’t have the time to waste with you,” even though we’re trying to be helpful.”
“What my team and I do is we really help companies weaponize their data assets.”

Tuesday Jan 15, 2019
Tuesday Jan 15, 2019
Vinay Seth Mohta is Managing Director at Manifold, an artificial intelligence engineering services firm with offices in Boston and Silicon Valley. Vinay has helped develop Manifold’s Lean AI process to build useful and accurate machine learning apps for a wide variety of customers.
During today’s episode, Vinay and I discuss common misconceptions about machine learning. Some of the other topics we cover are:
- The 3 buckets of machine learning problems and applications.
- Differences between traditional product development and developing apps with machine learning from Vinay’s perspective.
- Vinay’s opinion of what will change as a result of growth in the machine learning industry
- Maintaining a vision of a product while building it
Resources and Links:
- CRISP-DM
- Ways to Think About Machine Learning by Benedict Evans
- The Lean AI process
- Vinay Seth Mohta on LinkedIn
- Big Data, Big Dupe: A little book about a big bunch of nonsense by Stephen Few
Quotes from Vinay on today’s episode:
“We want to try and get them to dial back a little bit on the enthusiasm and the pixie dust aspect of AI and really, start thinking about it, more like a tool, or set of tools, or set of ideas that enable them with some new capabilities.”
“We have a process we called Lean AI and what we’ve incorporated into that is this idea of a feedback loop between a business understanding, a data understanding, then doing some engineering – so this is the data engineering, and then doing some modeling and then putting something in front of users.”
“Usually, team members who have domain knowledge [also] have pretty good intuition of what the data should show. And that is a good way to normalize everybody’s expectations.”
“You can really bring in some of the intuition that [clients] already have around their data and bring that into the conversation and that becomes an almost shared decision about what to do [with the data].”
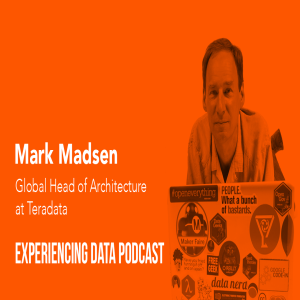
Thursday Jan 03, 2019
Thursday Jan 03, 2019
In Episode #003, I talked to Mark Madsen of Teradata on the common interests of analytics software architecture and product design. Mark spent most of the past 25 years working in the analytics field, and he is currently the global head of architecture for Teradata Consulting. He is a true analytics pioneer and a regular international speaker who also chairs several conferences and is on the O’Reilly Strata, Accelerate, and TDWI conference committees. If I only looked at job titles, Mark would be an odd fit for Experiencing Data, but the reality is that Mark has many of the traits of a good design thinker including a good sense of empathy about what users need in the world of analytics and decision support software. It’s a rare combination in my experience, so I hope you enjoy the interview. Besides, Mark is also highly entertaining
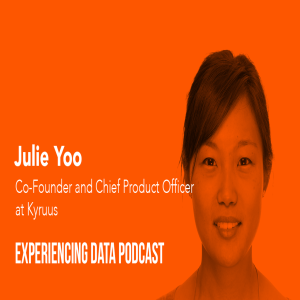
Tuesday Dec 04, 2018
Tuesday Dec 04, 2018
Julie Yoo is the co-founder of Kyruus, a medical technology company that is the developer of ProviderMatch. One of the most frustrating things about the healthcare system is the tendency for patients to be sent to the wrong type of doctor for their health issue. The industry term for this problem is patient access paradox.
ProviderMatch is software that directs patients to the proper medical specialist for their specific needs.
During today’s episode, Julie and I discuss the components that make ProviderMatch an effective tool. Some of the topics we touch on are:
- How ProviderMatch has changed the customer service side of healthcare.
- How ProviderMatch helps combat physician burnout.
- The 3 major user bases served by the application.
- The 3 types of tests Kyruus uses to test new and upgraded product features.
- The 3 levels of analytics that Kyruus uses to measure RIO and value.
Resources and Links:
- Kyruus
- Kyruus on Facebook
- Kyruus on LinkedIn
- Kyruus on Twitter
- Julie Yoo on Twitter
- Julie Yoo on LinkedIn
Thank you for joining us for today’s episode of Experiencing Data. Keep coming back for more episodes with great conversations about the world through the lens of analytics and design.
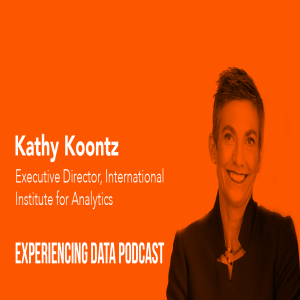
Wednesday Nov 21, 2018
Wednesday Nov 21, 2018
Kathy Koontz is the Executive Director of the Analytics Leadership Consortium at the International Institute for Analytics and my guest for today’s episode. The International Institute of Analytics is a research and advisory firm that discusses the latest trends and the best practices within the analytics field. We touch on how these strategies are used to build accurate and useful custom data products for businesses.
Kathy breaks down the steps of making analytics more accessible, especially since data products and analytics applications are more frequently being utilized by front-line workers and not PhDs and analytics experts. She uses her experience with a large property and casualty insurance company to illustrate her point about shifting your company’s approach to analytics to make it more accessible. Small adjustments to a data application make the process effective and comprehensible.
Kathy brings some great insights to today’s show about incorporating analytic techniques and user feedback to get the most value from your analytics and the data products you build for the information.
Conversation highlights:
- What is The International Institution of Analytics?
- What is the analytics leadership consortium?
- The “squishy” parts of analytics and how to compensate for them.
- The real value of analytics and how to use it on all levels of a company.
- How beta testers give perspective on data.
- The 3 steps to finding the ideal beta tester.
- Learning from the feedback and implementing it.
- How to keep ROI in mind during your project.
- Kathy’s parting advice for the audience.
Resources and Links:
Thank you for joining us for today’s episode of Experiencing Data. Keep coming back for more episodes with great conversations about the world of analytics and data.
Quotes from today’s episode:
“Oftentimes data scientists see the world through data and algorithms and predictions and they get enamored with the complexity of the model and the strength of its predictions and not so much with how easy it is for somebody to use it.” — Kathy Koontz
“You are not fully deployed until after you have received this [user] feedback and implemented the needed changes in the application.” — Kathy Koontz
“Analytics especially being deployed pervasively is maybe not a project but more of a transformation program.” — Kathy Koontz
“Go out and watch your user group that you want to utilize this data or this analytics to improve the performance.” — Kathy Koontz
“Obviously, it’s always cheaper to adjust things in pixels, and pencils than it is to adjust it in working code.” — Kathy Koo