
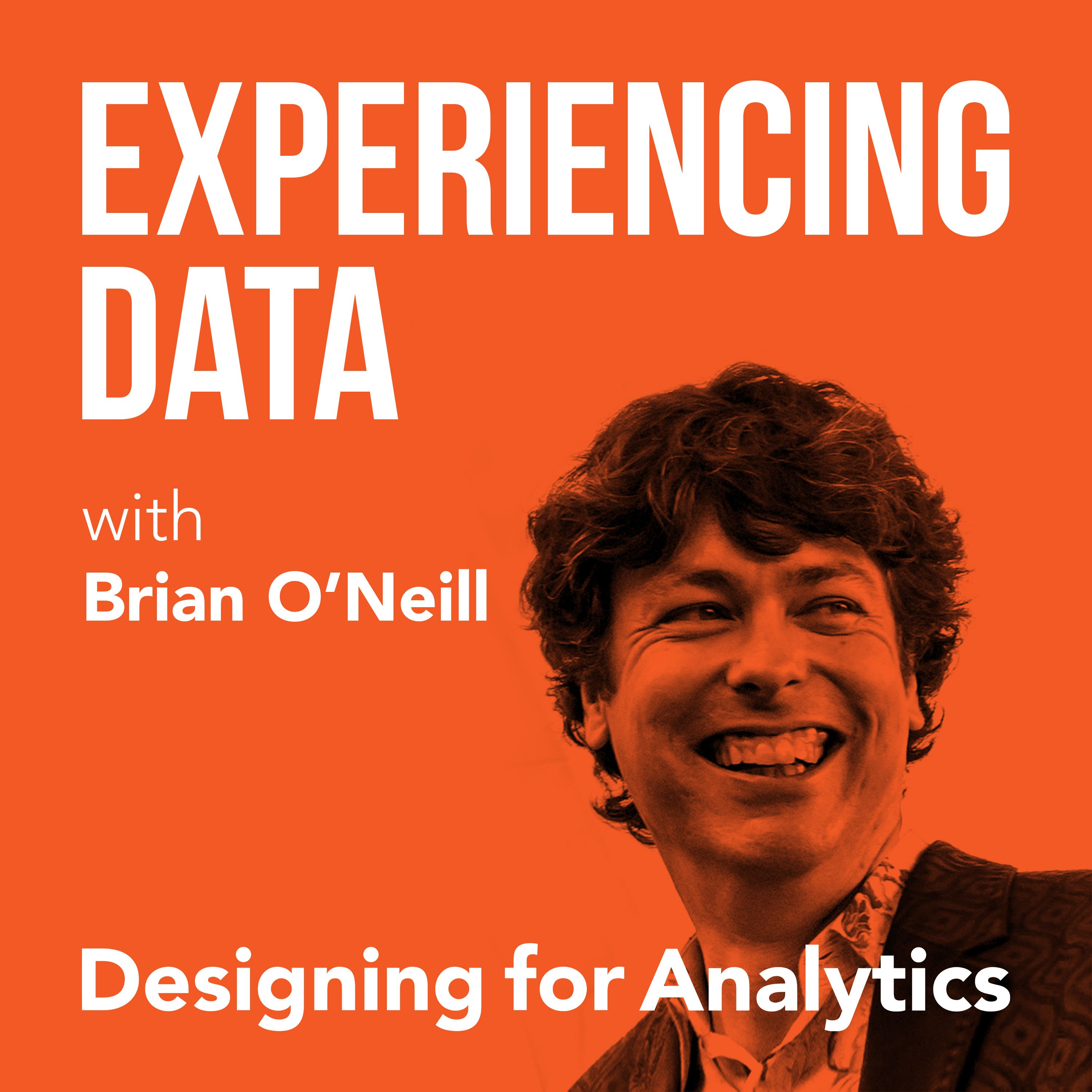
136.1K
Downloads
168
Episodes
Is the value of your enterprise analytics SAAS or AI product not obvious through it’s UI/UX? Got the data and ML models right...but user adoption of your dashboards and UI isn’t what you hoped it would be? While it is easier than ever to create AI and analytics solutions from a technology perspective, do you find as a founder or product leader that getting users to use and buyers to buy seems harder than it should be? If you lead an internal enterprise data team, have you heard that a ”data product” approach can help—but you’re concerned it’s all hype? My name is Brian T. O’Neill, and on Experiencing Data—one of the top 2% of podcasts in the world—I share the stories of leaders who are leveraging product and UX design to make SAAS analytics, AI applications, and internal data products indispensable to their customers. After all, you can’t create business value with data if the humans in the loop can’t or won’t use your solutions. Every 2 weeks, I release interviews with experts and impressive people I’ve met who are doing interesting work at the intersection of enterprise software product management, UX design, AI and analytics—work that you need to hear about and from whom I hope you can borrow strategies. I also occasionally record solo episodes on applying UI/UX design strategies to data products—so you and your team can unlock financial value by making your users’ and customers’ lives better. Hashtag: #ExperiencingData. JOIN MY INSIGHTS LIST FOR 1-PAGE EPISODE SUMMARIES, TRANSCRIPTS, AND FREE UX STRATEGY TIPS https://designingforanalytics.com/ed ABOUT THE HOST, BRIAN T. O’NEILL: https://designingforanalytics.com/bio/
Episodes
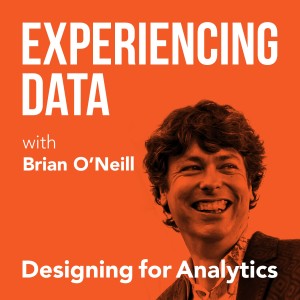
Tuesday Feb 20, 2024
Tuesday Feb 20, 2024
This week, I'm chatting with Karen Meppen, a founding member of the Data Product Leadership Community and a Data Product Architect and Client Services Director at Hakkoda. Today, we're tackling the difficult topic of developing data products in situations where a product-oriented culture and data infrastructures may still be emerging or “at odds” with a human-centered approach. Karen brings extensive experience and a strong belief in how to effectively negotiate the early stages of data maturity. Together we look at the major hurdles that businesses encounter when trying to properly exploit data products, as well as the necessity of leadership support and strategy alignment in these initiatives. Karen's insights offer a roadmap for those seeking to adopt a product and UX-driven methodology when significant tech or cultural hurdles may exist.
Highlights/ Skip to:
- I Introduce Karen Meppen and the challenges of dealing with data products in places where the data and tech aren't quite there yet (00:00)
- Karen shares her thoughts on what it's like working with "immature data" (02:27)
- Karen breaks down what a data product actually is (04:20)
- Karen and I discuss why having executive buy-in is crucial for moving forward with data products (07:48)
- The sometimes fuzzy definition of "data products." (12:09)
- Karen defines “shadow data teams” and explains how they sometimes conflict with tech teams (17:35)
- How Karen identifies the nature of each team to overcome common hurdles of connecting tech teams with business units (18:47)
- How she navigates conversations with tech leaders who think they already understand the requirements of business users (22:48)
- Using design prototypes and design reviews with different teams to make sure everyone is on the same page about UX (24:00)
- Karen shares stories from earlier in her career that led her to embrace human-centered design to ensure data products actually meet user needs (28:29)
- We reflect on our chat about UX, data products, and the “producty” approach to ML and analytics solutions (42:11)
Quotes from Today’s Episode
- "It’s not really fair to get really excited about what we hear about or see on LinkedIn, at conferences, etc. We get excited about the shiny things, and then want to go straight to it when [our] organization [may not be ] ready to do that, for a lot of reasons." - Karen Meppen (03:00)
- "If you do not have support from leadership and this is not something [they are] passionate about, you probably aren’t a great candidate for pursuing data products as a way of working." - Karen Meppen (08:30)
- "Requirements are just friendly lies." - Karen, quoting Brian about how data teams need to interpret stakeholder requests (13:27)
- "The greatest challenge that we have in technology is not technology, it’s the people, and understanding how we’re using the technology to meet our needs." - Karen Meppen (24:04)
- "You can’t automate something that you haven’t defined. For example, if you don’t have clarity on your tagging approach for your PII, or just the nature of all the metadata that you’re capturing for your data assets and what it means or how it’s handled—to make it good, then how could you possibly automate any of this that hasn’t been defined?" - Karen Meppen (38:35)
- "Nothing upsets an end-user more than lifting-and-shifting an existing report with the same problems it had in a new solution that now they’ve never used before." - Karen Meppen (40:13)
- “Early maturity may look different in many ways depending upon the nature of business you’re doing, the structure of your data team, and how it interacts with folks.” (42:46)
Links
- Data Product Leadership Community https://designingforanalytics.com/community/
- Karen Meppen on LinkedIn: https://www.linkedin.com/in/karen--m/
- Hakkōda, Karen's company, for more insights on data products and services:https://hakkoda.io/
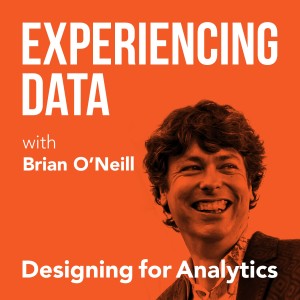
Tuesday Feb 06, 2024
Tuesday Feb 06, 2024
This week I’m chatting with Caroline Zimmerman, Director of Data Products and Strategy at Profusion. Caroline shares her journey through the school of hard knocks that led to her discovery that incorporating more extensive UX research into the data product design process improves outcomes. We explore the complicated nature of discovering and building a better design process, how to engage end users so they actually make time for research, and why understanding how to navigate interdepartmental politics is necessary in the world of data and product design. Caroline reveals the pivotal moment that changed her approach to data product design, as well as her learnings from evolving data products with the users as their needs and business strategies change. Lastly, Caroline and I explore what the future of data product leadership looks like and Caroline shares why there's never been a better time to work in data.
Highlights/ Skip to:
- Intros and Caroline describes how she learned crucial lessons on building data products the hard way (00:36)
- The fundamental moment that helped Caroline to realize that she needed to find a different way to uncover user needs (03:51)
- How working with great UX researchers influenced Caroline’s approach to building data products (08:31)
- Why Caroline feels that exploring the ‘why’ is foundational to designing a data product that gets adopted (10:25)
- Caroline’s experience building a data model for a client and what she learned from that experience when the client’s business model changed (14:34)
- How Caroline addresses the challenge of end users not making time for user research (18:00)
- A high-level overview of the UX research process when Caroline’s team starts working with a new client (22:28)
- The biggest challenges that Caroline faces as a Director of Data Products, and why data products require the ability to navigate company politics and interests (29:58)
- Caroline describes the nuances of working with different stakeholder personas (35:15)
- Why data teams need to embrace a more human-led approach to designing data products and focus less on metrics and the technical aspects (38:10)
- Caroline’s closing thoughts on what she’d like to share with other data leaders and how you can connect with her (40:48)
Quotes from Today’s Episode
- “When I was first starting out, I thought that you could essentially take notes on what someone was asking for, go off and build it to their exact specs, and be successful. And it turns out that you can build something to exact specs and suffer from poor adoption and just not be solving problems because I did it as a wish fulfillment, laundry-list exercise rather than really thinking through user needs.” — Caroline Zimmerman (01:11)
- “People want a thing. They’re paying for a thing, right? And so, just really having that reflex to try to gently come back to that why and spending sufficient time exploring it before going into solution build, even when people are under a lot of deadline pressure and are paying you to deliver a thing [is the most important element of designing a data product].” – Caroline Zimmerman (11:53)
- “A data product evolves because user needs change, business models change, and business priorities change, and we need to evolve with it. It’s not like you got it right once, and then you’re good for life. At all.” – Caroline Zimmerman (17:48)
- “I continue to have lots to learn about stakeholder management and understanding the interplay between what the organization needs to be successful, but also, organizations are made up of people with personal interests, and you need to understand both.” – Caroline Zimmerman (30:18)
- “Data products are built in a political context. And just being aware of that context is important.” – Caroline Zimmerman (32:33)
- “I think that data, maybe more than any other function, is transversal. I think data brings up politics because, especially with larger organizations, there are those departmental and team silos. And the whole thing about data is it cuts through those because it touches all the different teams. It touches all the different processes. And so in order to build great data products, you have to be navigating that political context to understand how to get things done transversely in organizations where most stuff gets done vertically.” – Caroline Zimmerman (34:37)
- “Data leadership positions are data product expertise roles. And I think that often it’s been more technical people that have advanced into those roles. If you follow the LinkedIn-verse in data, it’s very much on every data leader’s mind at the moment: how do you articulate benefits to your CEO and your board and try to do that before it’s too late? So, I’d say that’s really the main thing and that there’s just never been a better time to be a data product person.” – Caroline Zimmerman (37:16)
Links
- Profusion: https://profusion.com/
- Caroline Zimmerman LinkedIn: https://www.linkedin.com/in/caroline-zimmerman-4a531640/
- Nick Zervoudis LinkedIn: https://www.linkedin.com/in/nzervoudis/
- Email: mailto:carolinez@profusion.com
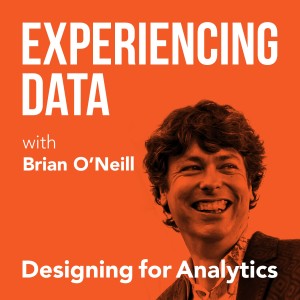
Tuesday Jan 23, 2024
Tuesday Jan 23, 2024
This week, I’m chatting with Steve Portigal, who is the Principal of Portigal Consulting and the Author of Interviewing Users. We discuss the changes that prompted him to release a second version of his book 10 years after its initial release, and dive into the best practices that any team can implement to start unlocking the value of data product UX research. Steve explains that the key to making time for user research is knowing what business value you’re after, not simply having a list of research questions. We then role-play through some in-depth examples of real-life experiences we’ve seen from both end users and leadership when it comes to implementing a user research strategy. Thhroughout our conversation, we come back to the idea that even taking imperfect action towards doing user research can lead to increased data product adoption and business value.
Highlights/ Skip to:
- I introduce Steve Portigal, Principal of Portigal Consulting and Author of Interviewing Users (00:38)
- What changes caused Steve to release a second edition of his book (00:58)
- Steve and I discuss the importance of understanding how to conduct effective user research (03:44)
- Steve explains why it’s crucial to understand that the business challenge and the research questions are two different things (08:16)
- Brian and Steve role-play a common scenario that comes up in user research, and Steve explains an optimal workflow for user research (11:50)
- The importance of provocation in performing user research (21:02)
- How Steve would handle a situation where a member of leadership is preventing research being done with end users (24:23)
- Why a consultative approach is valuable when getting buy-in for conducting user research (35:04)
- Steve shares some of the major benefits of taking imperfect action towards starting user research (36:59)
- The impact and value even easy wins in user research can have (42:54)
- Steve describes the exploratory nature of user research and how to maximize the chance of finding the most valuable insights (46:57)
- Where you can connect with Steve and get a copy of v2 of his book, Interviewing Users (49:35)
Quotes from Today’s Episode
- “If you don’t know what you’re doing, and you don’t know what you should be investing effort-wise, that’s the inexperience in the approach. If you don’t know how to plan, what should we be trying to solve in this research? What are we trying to learn? What are we going to do with it in the organization? Who should we be talking to? How do we find them? What do we ask them? And then a really good one: how do we make sense of that information so that it has impact that we can take away?” — Steve Portigal (07:15)
- “What do people get [from user research]? I think the chance for a team to align around something that comes in from the outside.” – Steve Portigal (41:36)
- On the impact user research can have if teams embrace it: “They had a product that did a thing that no one [understood], and they had to change the product, but also change how they talked about it, change how they built it, and change how they packaged it. And that was a really dramatic turnaround. And it came out of our research, but [mostly] because they really leaned into making use of this stuff.” – Steve Portigal (42:35)
- "If we knew all the questions to ask, we would just write a survey, right? It’s a lower time commitment from the participant to do that. But we’re trying to get at what we don’t know that we don’t know. For some of us, that’s fun!" – Steve Portigal (48:36)
Links
- Interviewing Users (use code DATA20 to get 20% off the list price): https://rosenfeldmedia.com/books/interviewing-users-second-edition/
- Personal website: https://portigal.com
- Publisher website: https://rosenfeldmedia.com
- LinkedIn: https://www.linkedin.com/in/steveportigal/
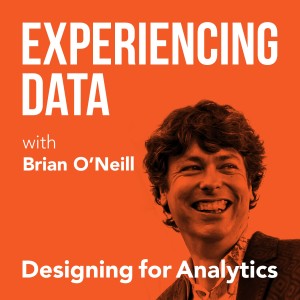
Tuesday Jan 09, 2024
134 - What Sanjeev Mohan Learned Co-Authoring “Data Products for Dummies”
Tuesday Jan 09, 2024
Tuesday Jan 09, 2024
In this episode, I’m chatting with former Gartner analyst Sanjeev Mohan who is the Co-Author of Data Products for Dummies. Throughout our conversation, Sanjeev shares his expertise on the evolution of data products, and what he’s seen as a result of implementing practices that prioritize solving for use cases and business value. Sanjeev also shares a new approach of structuring organizations to best implement ownership and accountability of data product outcomes. Sanjeev and I also explore the common challenges of product adoption and who is responsible for user experience. I purposefully had Sanjeev on the show because I think we have pretty different perspectives from which we see the data product space.
Highlights/ Skip to:
- I introduce Sanjeev Mohan, co-author of Data Products for Dummies (00:39)
- Sanjeev expands more on the concept of writing a “for Dummies” book (00:53)
- Sanjeev shares his definition of a data product, including both a technical and a business definition (01:59)
- Why Sanjeev believes organizational changes and accountability are the keys to preventing the acceleration of shipping data products with little to no tangible value (05:45)
- How Sanjeev recommends getting buy-in for data product ownership from other departments in an organization (11:05)
- Sanjeev and I explore adoption challenges and the topic of user experience (13:23)
- Sanjeev explains what role is responsible for user experience and design (19:03)
- Who should be responsible for defining the metrics that determine business value (28:58)
- Sanjeev shares some case studies of companies who have adopted this approach to data products and their outcomes (30:29)
- Where companies are finding data product managers currently (34:19)
- Sanjeev expands on his perspective regarding the importance of prioritizing business value and use cases (40:52)
- Where listeners can get Data Products for Dummies, and learn more about Sanjeev’s work (44:33)
Quotes from Today’s Episode
- “You may slap a label of data product on existing artifact; it does not make it a data product because there’s no sense of accountability. In a data product, because they are following product management best practices, there must be a data product owner or a data product manager. There’s a single person [responsible for the result]. — Sanjeev Mohan (09:31)
- “I haven’t even mentioned the word data mesh because data mesh and data products, they don’t always have to go hand-in-hand. I can build data products, but I don’t need to go into the—do all of data mesh principles.” – Sanjeev Mohan (26:45)
- “We need to have the right organization, we need to have a set of processes, and then we need a simplified technology which is standardized across different teams. So, this way, we have the benefit of reusing the same technology. Maybe it is Snowflake for storage, DBT for modeling, and so on. And the idea is that different teams should have the ability to bring their own analytical engine.” – Sanjeev Mohan (27:58)
- “Generative AI, right now as we are recording, is still in a prototyping phase. Maybe in 2024, it’ll go heavy-duty production. We are not in prototyping phase for data products for a lot of companies. They’ve already been experimenting for a year or two, and now they’re actually using them in production. So, we’ve crossed that tipping point for data products.” – Sanjeev Mohan (33:15)
- “Low adoption is a problem that’s not just limited to data products. How long have we had data catalogs, but they have low adoption. So, it’s a common problem.” – Sanjeev Mohan (39:10)
- “That emphasis on technology first is a wrong approach. I tell people that I’m sorry to burst your bubble, but there are no technology projects, there are only business projects. Technology is an enabler. You don’t do technology for the sake of technology; you have to serve a business cause, so let’s start with that and keep that front and center.” – Sanjeev Mohan (43:03)
Links
- Data Products for Dummies: https://www.dataops.live/dataproductsfordummies
- “What Exactly is A Data Product” article: https://medium.com/data-mesh-learning/what-exactly-is-a-data-product-7f6935a17912
- It Depends: https://www.youtube.com/@SanjeevMohan
- Chief Data Analytics and Product Officer of Equifax: https://www.youtube.com/watch?v=kFY7WGc-jFM
- SanjMo Consulting: https://www.sanjmo.com/
- dataops.live: https://dataops.live
- dataops.live/dataproductsfordummies: https://dataops.live/dataproductsfordummies
- LinkedIn: https://www.linkedin.com/in/sanjmo/
- Medium articles: https://sanjmo.medium.com
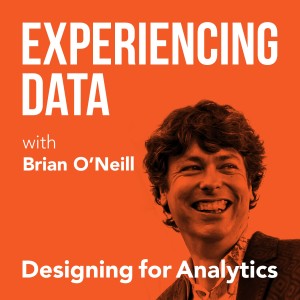
Tuesday Dec 26, 2023
133 - New Experiencing Data Interviews Coming in January 2024
Tuesday Dec 26, 2023
Tuesday Dec 26, 2023
Today I am sharing some highlights for 2023 from the podcast, and also letting you all know I’ll be taking a break from the podcast for the rest of December, but I’ll be back with a new episode on January 9th, 2024. I’ve also got two links to share with you—details inside!
Transcript
Greetings everyone - I’m taking a little break from Experiencing Data over December of 2023, but I’ll be back in January with more interviews and insights on leveraging UX design and product management to create indispensable data products, machine learning apps, and decision support tools.
Experiencing Data turned this year five years old back in November, with over 130 episodes to date! I still can’t believe it’s been going that long and how far we’ve come.
Some highlights for me in 2023 included launching the Data Product Leadership Community, finding out that the show is now in the top 2% of all podcasts worldwide according to ListenNotes, and most of all, hearing from you that the podcast, and my writing, and the guests that I have brought on are having an impact on your work, your careers, and hopefully the lives of your customers, users, and stakeholders as well!
So, for now, I’ve got just two links for you:
If you’re wondering how to either:
- support the show yourself with a really fast review on Apple Podcasts,
- to record a quick audio question for me to answer on the show,
- or if you want to join my free Insights mailing lists where I share my bi-weekly ideas and thoughts and 1-page episode summaries of all the show drops that I put out here on Experiencing Data.
…just head over to designingforanalytics.com/podcast and you’ll get links to all those things there.
And secondly, if you need help increasing customer adoption, delight, the business value, or the usability of your analytics and machine learning applications in 2024, I invite you to set up a free discovery call with me 1 on 1.
You bring the questions, I’ll bring my ears, and by the end of the call, I’ll give you my best advice on how to move forward with your situation – whether it’s working with me or not. To schedule one of those free discovery calls, visit designingforanalytics.com/go
And finally, there will be some news coming out next year with the show, as well as my business, so I hope you’ll hop on the mailing list and stay tuned, that’s probably the best place to do that. And if you celebrate holidays in December and January, I hope they’re safe, enjoyable, and rejuvenating. Until 2024, stay tuned right here - and in the words of the great Arnold Schwarzenegger, I’ll be back.
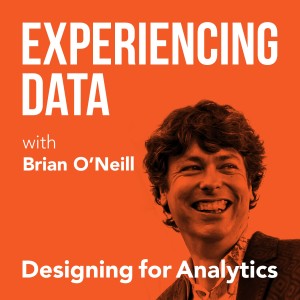
Tuesday Dec 12, 2023
Tuesday Dec 12, 2023
In this conversation with Klara Lindner, Service Designer at diconium data, we explore how behavioral science and UX can be used to increase adoption of data products. Klara describes how she went from having a highly technical career as an electrical engineer and being the founder of a solar startup to her current role in service design for data products. Klara shares powerful insights into the value of user research and human-centered design, including one which stopped me in my tracks during this episode: how the people making data products and evangelizing data-driven decision making aren’t actually following their own advice when it comes to designing their data products. Klara and I also explore some easy user research techniques that data professionals can use, and discuss who should ultimately be responsible for user adoption of data products. Lastly, Klara gives us a peek at her upcoming December 19th, 2023 webinar with the The Data Product Leadership Community (DPLC) where she will be going deeper on two frameworks from psychology and behavioral science that teams can use to increase adoption of data products. Klara is also a founding member of the DPLC and was one of—if not the very first—design/UX professionals to join.
Highlights/ Skip to:
- I introduce Klara, and she explains the role of Service Design to our audience (00:49)
- Klara explains how she realized she’s been doing design work longer than she thought by reflecting on the company she founded, Mobisol (02:09)
- How Klara balances the desire to design great dashboards with the mission of helping end users (06:15)
- Klara describes the psychology behind user research and her upcoming talk on December 19th at The Data Product Leadership Community (08:32)
- What data product teams can do as a starting point to begin implementing user research principles (10:52)
- Klara gives a powerful example of the type of insight and value even basic user research can provide (12:49)
- Klara and I discuss a key revelation when it comes to designing data products for users, which is the irony that even developers use intuition as well as quantitative data when building (16:43)
- What adjustments Klara had to make in her thinking when moving from a highly technical background to doing human-centered design (21:08)
- Klara describes the two frameworks for driving adoption that she’ll be sharing in her talk at the DPLC on December 19th (24:23)
- An example of how understanding and addressing adoption blockers is important for product and design teams (30:44)
- How Klara has seen her teams adopt a new way of thinking about product & service design (32:55)
- Klara gives her take on the Jobs to be Done framework, which she will also be sharing in her talk at the DPLC on December 19th (35:26)
- Klara’s advice to teams that are looking to build products around generative AI (39:28)
- Where listeners can connect with Klara to learn more (41:37)
Links
- diconium data: http://www.diconium.com/
- LinkedIn: https://www.linkedin.com/in/klaralindner/
- Personal Website: https://magic-investigations.com/
- Hear Klara speak on Dec 19, 2023 at 10am ET here: https://designingforanalytics.com/community/
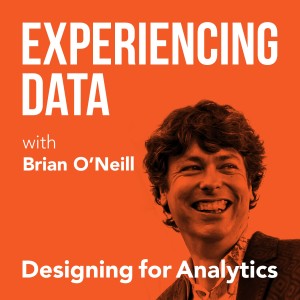
Tuesday Nov 28, 2023
Tuesday Nov 28, 2023
This week I’m covering Part 1 of the 15 Ways to Increase User Adoption of Data Products, which is based on an article I wrote for subscribers of my mailing list. Throughout this episode, I describe why focusing on empathy, outcomes, and user experience leads to not only better data products, but also better business outcomes. The focus of this episode is to show you that it’s completely possible to take a human-centered approach to data product development without mandating behavioral changes, and to show how this approach benefits not just end users, but also the businesses and employees creating these data products.
Highlights/ Skip to:
- Design behavior change into the data product. (05:34)
- Establish a weekly habit of exposing technical and non-technical members of the data team directly to end users of solutions - no gatekeepers allowed. (08:12)
- Change funding models to fund problems, not specific solutions, so that your data product teams are invested in solving real problems. (13:30)
- Hold teams accountable for writing down and agreeing to the intended benefits and outcomes for both users and business stakeholders. Reject projects that have vague outcomes defined. (16:49)
- Approach the creation of data products as “user experiences” instead of a “thing” that is being built that has different quality attributes. (20:16)
- If the team is tasked with being “innovative,” leaders need to understand the innoficiency problem, shortened iterations, and the importance of generating a volume of ideas (bad and good) before committing to a final direction. (23:08)
- Co-design solutions with [not for!] end users in low, throw-away fidelity, refining success criteria for usability and utility as the solution evolves. Embrace the idea that research/design/build/test is not a linear process. (28:13)
- Test (validate) solutions with users early, before committing to releasing them, but with a pre-commitment to react to the insights you get back from the test. (31:50)
Links:
- 15 Ways to Increase Adoption of Data Products: https://designingforanalytics.com/resources/15-ways-to-increase-adoption-of-data-products-using-techniques-from-ux-design-product-management-and-beyond/
- Company website: https://designingforanalytics.com
- Episode 54: https://designingforanalytics.com/resources/episodes/054-jared-spool-on-designing-innovative-ml-ai-and-analytics-user-experiences/
- Episode 106: https://designingforanalytics.com/resources/episodes/106-ideaflow-applying-the-practice-of-design-and-innovation-to-internal-data-products-w-jeremy-utley/
- Ideaflow: https://www.amazon.com/Ideaflow-Only-Business-Metric-Matters/dp/0593420586/
- Podcast website: https://designingforanalytics.com/podcast
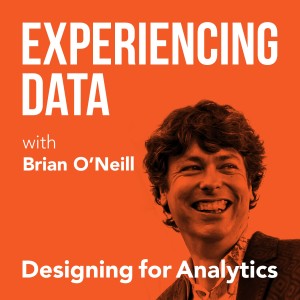
Tuesday Nov 14, 2023
Tuesday Nov 14, 2023
Today I’m joined by Nick Zervoudis, Data Product Manager at CKDelta. As we dive into his career and background, Nick shares insights into his approach when it comes to developing both internal and external data products. Nick explains why he feels that a software engineering approach is the best way to develop a product that could have multiple applications, as well as the unique way his team is structured to best handle the needs of both internal and external customers. He also talks about the UX design course he took, how that affected his data product work and research with users, and his thoughts on dashboard design. We discuss common themes he’s observed when data product teams get it wrong, and how he manages feelings of imposter syndrome in his career as a DPM.
Highlights/ Skip to:
- I introduce Nick, who is a Data Product Manager at CKDelta (00:35)
- Nick’s mindset around data products and how his early career in consulting shaped his approach (01:30)
- How Nick defines a data product and why he focuses more on the process rather than the end product (03:59)
- The types of data products that Nick has helped design and his work on both internal and external projects at CKDelta (07:57)
- The similarities and differences of working with internal versus external stakeholders (12:37)
- Nick dives into the details of the data products he has built and how they feed into complex use cases (14:21)
- The role that Nick plays in the Delta Power SaaS application and how the CKDelta team is structured around that product (17:14)
- Where Nick sees data products going wrong and how he’s found value in filling those gaps (23:30)
- Nick’s view on how a digital-first mindset affects the scalability of data products (26:15)
- Why Nick is often heavily involved in the design element of data product development and the course he took that helped shape his design work (28:55)
- The imposter syndrome that Nick has experienced when implementing this new strategy to data product design (36:51)
- Why Nick feels that figuring things out yourself is an inherent part of the DPM role (44:53)
- Nick shares the origins and information on the London Data Product Management meetup (46:08)
Quotes from Today’s Episode
- “What I’m always trying to do is see, how can we best balance the customer’s need to get exactly the data point or insight that they’re after to the business need. ... There’s that constant tug of war between customization and standardization that I have the joy of adjudicating. I think it’s quite fun.” — Nick Zervoudis (16:40)
- “I’ve had times where I was hired, told, 'You’re going to be the product manager for this data product that we have,' as if it’s already, to some extent built and maybe the challenge is scaling it or bringing it to more customers or improving it, and then within a couple of weeks of starting to peek under the hood, realizing that this thing that is being branded a product is actually a bunch of projects hiding under a trench coat.” — Nick Zervoudis (24:04)
- “If I just speak to five users because they’re the users, they’ll give me the insight I need. […] Even when you have a massive product with a huge user base, people face the same issues.” — Nick Zervoudis (33:49)
- “For me, it’s more about making sure that you’re bringing that more software engineering way of building things, but also, before you do that, knowing that your users' needs are going to [be varied]. So, it’s a combination of both, are we building the right thing—in other words, a product that’s flexible enough to meet the different needs of different users—but also, are we building it in the right way?” – Nick Zervoudis (27:51)
- “It’s not to say I’m the only person thinking about [UX design], but very often, I’m the one driving it.” – Nick Zervoudis (30:55)
- “You’re never going to be as good at the thing your colleague does because their job almost certainly is to be a specialist: they’re an architect, they’re a designer, they’re a developer, they’re a salesperson, whereas your job [as a DPM] is to just understand it enough that you can then pass information across other people.” – Nick Zervoudis (41:12)
- “Every time I feel like an imposter, good. I need to embrace that, because I need to be working with people that understand something better than me. If I’m not, then maybe something’s gone wrong there. That’s how I’ve actually embraced impostor syndrome.” – Nick Zervoudis (41:35)
Links
- CKDelta: https://www.ckdelta.ie
- LinkedIn: https://www.linkedin.com/in/nzervoudis/
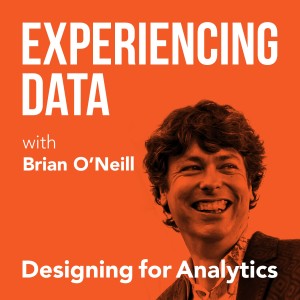
Tuesday Oct 31, 2023
Tuesday Oct 31, 2023
Today I’m joined by Marnix van de Stolpe, Product Owner at Coolblue in the area of data science. Throughout our conversation, Marnix shares the story of how he joined a data science team that was developing a solution that was too focused on the delivery of a data-science metric that was not on track to solve a clear customer problem. We discuss how Marnix came to the difficult decision to throw out 18 months of data science work, what it was like to switch to a human-centered, product approach, and the challenges that came with it. Marnix shares the impact this decision had on his team and the stakeholders involved, as well as the impact on his personal career and the advice he would give to others who find themselves in the same position. Marnix is also a Founding Member of the Data Product Leadership Community and will be going much more into the details and his experience live on Zoom on November 16 @ 2pm ET for members.
Highlights/ Skip to:
- I introduce Marnix, Product Owner at Coolblue and one of the original members of the Data Product Leadership Community (00:35)
- Marnix describes what Coolblue does and his role there (01:20)
- Why and how Marnix decided to throw away 18 months of machine learning work (02:51)
- How Marnix determined that the KPI (metric) being created wasn’t enough to deliver a valuable product (07:56)
- Marnix describes the conversation with his data science team on mapping the solution back to the desired outcome (11:57)
- What the culture is like at Coolblue now when developing data products (17:17)
- Marnix’s advice for data product managers who are coming into an environment where existing work is not tied to a desired outcome (18:43)
- Marnix and I discuss why data literacy is not the solution to making more impactful data products (21:00)
- The impact that Marnix’s human-centered approach to data product development has had on the stakeholders at Coolblue (24:54)
- Marnix shares the ultimate outcome of the product his team was developing to measure product returns (31:05)
- How you can get in touch with Marnix (33:45)
Links
- Coolblue: https://www.coolblue.nl
- LinkedIn: https://www.linkedin.com/in/marnixvdstolpe/
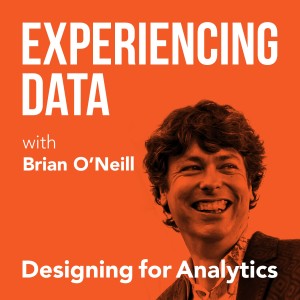
Tuesday Oct 17, 2023
Tuesday Oct 17, 2023
Today I’m joined by Vishal Singh, Head of Data Products at Starburst and co-author of the newly published e-book, Data Products for Dummies. Throughout our conversation, Vishal explains how the variations in definitions for a data product actually led to the creation of the e-book, and we discuss the differences between our two definitions. Vishal gives a detailed description of how he believes Data Product Managers should be conducting their discovery and gathering feedback from end users, and how his team evaluates whether their data products are truly successful and user-friendly.
Highlights/ Skip to:
- I introduce Vishal, the Head of Data Products at Starburst and contributor of the e-book Data Products for Dummies (00:37)
- Vishal describes how his customers at Starburst all had a common problem, but differing definitions of a data product, which led to the creation of his e-book (01:15)
- Vishal shares his one-sentence definition of a data product (02:50)
- How Vishal’s definition of a data product differs from mine, and we both expand on the possibilities between the two (05:33)
- The tactics Vishal uses to useful feedback to ensure the data products he develops are valuable for end users (07:48)
- Why Vishal finds it difficult to get one on one feedback from users during the iteration phase of data product development (11:07)
- The danger of sunk cost bias in the iteration phase of data product development (13:10)
- Vishal describes how he views the role of a DPM when it comes to doing effective initial discovery (15:27)
- How Vishal structures his teams and their interactions with each other and their end users (21:34)
- Vishal’s thoughts on how design affects both data scientists and end users (24:16)
- How DPMs at Starburst evaluate if the data product design is user-friendly (28:45)
- Vishal’s views on where Designers are valuable in the data product development process (35:00)
- Vishal and I discuss the importance of ensuring your products truly solve your user’s problems (44:44)
- Where you can learn more about Vishal’s upcoming events and the e-book, Data Products for Dummies (49:48)
Links
- Starburst: https://www.starburst.io/
- Data Products for Dummies: https://www.starburst.io/info/data-products-for-dummies/
- “How to Measure the Impact of Data Products with Doug Hubbard”: https://designingforanalytics.com/resources/episodes/080-how-to-measure-the-impact-of-data-productsand-anything-else-with-forecasting-and-measurement-expert-doug-hubbard/
- Trino Summit: https://www.starburst.io/info/trinosummit2023/
- Galaxy Platform: https://www.starburst.io/platform/starburst-galaxy/
- Datanova Summit: https://www.starburst.io/datanova/
- LinkedIn: https://www.linkedin.com/in/singhsvishal/
- Twitter: https://twitter.com/vishal_singh