
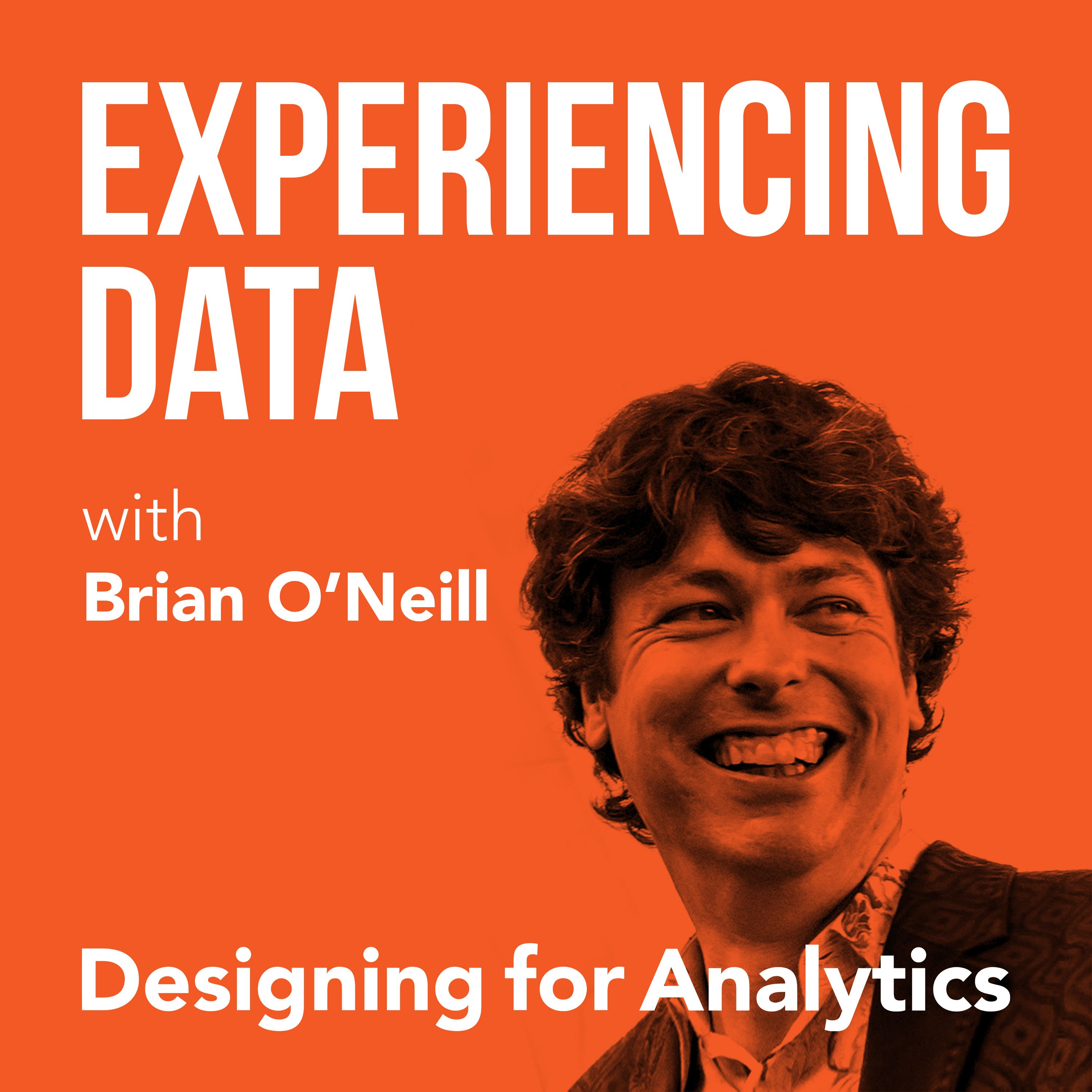
127.8K
Downloads
161
Episodes
If you’re a leader tasked with generating business and org. value through ML/AI and analytics, you’ve probably struggled with low user adoption. Making the tech gets easier, but getting users to use, and buyers to buy, remains difficult—but you’ve heard a ”data product” approach can help. Can it? My name is Brian T. O’Neill, and on Experiencing Data—one of the top 2% of podcasts in the world—I offer you a consulting designer’s perspective on why creating ML and analytics outputs isn’t enough to create business and UX outcomes. How can UX design and product management help you create innovative ML/AI and analytical data products? What exactly are data products—and how can data product management help you increase user adoption of ML/analytics—so that stakeholders can finally see the business value of your data? Every 2 weeks, I answer these questions via solo episodes and interviews with innovative chief data officers, data product management leaders, and top UX professionals. Hashtag: #ExperiencingData. PODCAST HOMEPAGE: Get 1-page summaries, text transcripts, and join my Insights mailing list: https://designingforanalytics.com/ed ABOUT THE HOST, BRIAN T. O’NEILL: https://designingforanalytics.com/bio/
Episodes
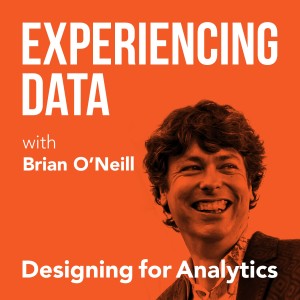
Tuesday Feb 07, 2023
Tuesday Feb 07, 2023
Today I’m chatting with Dr. Sebastian Klapdor, Chief Data Officer for Vista. Sebastian has developed and grown a successful Data Product Management team at Vista, and it all began with selling his vision to the rest of the executive leadership. In this episode, Sebastian explains what that process was like and what he learned. Sebastian shares valuable insights on how he implemented a data product orientation at Vista, what makes a good data product manager, and why technology usage isn’t the only metric that matters when measuring success. He also shares what he would do differently if he had to do it all over again.
Highlights/ Skip to:
- How Sebastian defines a data product (01:48)
- Brian asks Sebastian about the change management process in leadership when implementing a data product approach (07:40)
- The three dimensions that Sebastian and his team measure to determine adoption success (10:22)
- Sebastian shares the financial results of Vista adopting a data product approach (12:56)
- The size and scale of the data team at Vista, and how their different roles ensure success (14:30)
- Sebastian explains how Vista created and grew a team of 35 data product managers (16:47)
- The skills Sebastian feels data product managers need to be successful at Vista (22:02)
- Sebastian describes what he would do differently if he had to implement a data product approach at a company again (29:46)
Quotes from Today’s Episode
- “You need to establish a culture, and that’s often the hardest part that takes the longest - to treat data as an asset, and not to treat it as a byproduct, but to treat it as a product and treat it as a valuable thing.” – Sebastian Klapdor (07:56)
- “One source of data product managers is taking data professionals. So, you take data engineers, data scientists, or former analysts, and develop them into the role by coaching them [through] the product management skills from the software industry.” – Sebastian Klapdor (17:39)
- “We went out there and we were hiring people in the market who were experienced [Product Managers]. But we also see internal people, actually grooming and growing into all of these roles, both from these 80 folks who have been around before, but also from other areas of Vista.” – Sebastian Klapdor (20:28)
- “[Being a good Product Manager] comes back to the good old classics of collaborating, of being empathetic to where other people are at, their priorities, and understanding where [our] priorities fit into their bigger piece, and jointly aligning on what is valuable for Vista.” – Sebastian Klapdor (22:27)
- “I think there’s nothing more detrimental than saying, ‘Yeah, sure, we can deliver things, and with data, it can do everything.’ And then you disappoint people and you don’t stick to your promises. … If you don’t stick to your promise, it will hurt you.” – Sebastian Klapdor (23:04)
- “You don’t do the typical waterfall approach of solving business problems with data. You don’t do the approach that a data scientist tries to get some data, builds a model, and hands it over to data engineer who should productionize that. And then the data engineer gets back and says certain features can’t be productionized because it’s very complex to get the data on a daily basis, or in real time. By doing [this work] in a data product team, you can work actually in Agile and you’re super fast building what we call a minimum lovable product.” – Sebastian Klapdor (26:15)
- “That was the biggest learning … whom do we staff as data product managers? And what do we expect of a good data product manager? How does a career path look like? That took us a really long time to figure out.” – Sebastian Klapdor (30:18)
- “We have a big, big, big commitment that we want to start stuffing UX designers onto our [data] product teams.” - Sebastian Klapdor (21:12)
Links
- Vista: https://vista.io
- LinkedIn: https://www.linkedin.com/in/sebastianklapdor/
- Vista Blog: https://vista.io/blog
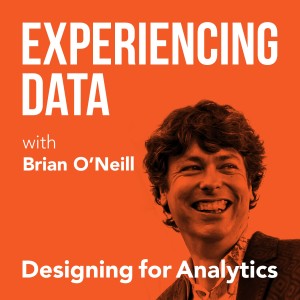
Tuesday Jan 24, 2023
Tuesday Jan 24, 2023
Today I’m chatting with Bob Mason, Managing Partner at Argon Ventures. Bob is a VC who seeks out early-stage founders in the ML/AI space and helps them inform their go-to-market, product, and design strategies. In this episode, Bob reveals what he looks for in early-stage data and intelligence startups who are trying to leverage ML/AI. He goes on to explain why it’s important to identify what your strengths are and what you enjoy doing so you can surround yourself with the right team. Bob also shares valuable insight into how to earn trust with potential customers as an early-stage startup, how design impacts a product’s success, and his strategy for differentiating yourself and creating a valuable product outside of the ubiquitous “platform play.”
Highlights/ Skip to:
- Bob explains why and how Argon Ventures focuses their investments in intelligent industry companies (00:53)
- Brian and Bob discuss the importance of prioritizing go-to-market strategy over technology (03:42)
- How Bob views the career progression from data science to product management, and the ways in which his own career has paralleled that journey (07:21)
- The role customer adoption and user experience play for Bob and the companies he invests in, both pre-investment and post-investment (11:10)
- Brian and Bob discuss the design capabilities of different teams and why Bob feels it’s something leaders need to keep top of mind (15:25)
- Bob explains his recommendation to seek out quick wins for AI companies who can’t expect customers to wait for an ROI (19:09)
- The importance Bob sees in identifying early adopters during a sales cycle for early-stage startups (21:34)
- Bob describes how being customer-centric allows start-ups to build trust, garner quick wins, and inform their product strategy (23:42)
- Bob and Brian dive into Bob’s belief that solving intrinsic business problems by vertical increases a start-up’s chance of success substantially over “the platform play” (27:29)
- Bob gives insight into product trends he believes are going to be extremely impactful in the near future (29:05)
Quotes from Today’s Episode
- “In a former life, I was a software engineer, founder, and CTO myself, so I have to watch myself to not just geek out on the technology itself because the most important element when you’re determining if you want to move forward with investment or not, is this: is there a real problem here to be solved or is this technology in search of a problem?” — Bob Mason (01:51)
- “User-centric research is really valuable, particularly at the earliest stages. If you’re just off by a degree or two, several years down the road, that can be a really material roadblock that you hit. And so, starting off on the right foot, I think is super, super valuable.” – Bob Mason (06:12)
- “I don’t think the technical folks in an early-stage startup absolve themselves of not being really intimately involved with their go-to-market and who they’re ultimately creating value for.” – Bob Mason (07:07)
- “When we’re making an investment decision, startups don’t generally have any customers, and so we don’t necessarily use the signal of long-term customer adoption as a driver for our initial investment decision. But it’s very much top of mind after investment and as we’re trying to build and bring the first version of the product to market. Being very thoughtful and mindful of sort of customer experience and long-term adoption is absolutely critical.” – Bob Mason (11:23)
- “If you’re a scientist, the way you’re presenting both raw data and sort of summaries of data could be quite different than if you’re working with a business analyst that’s a few years out of college with a liberal arts degree. How you interpret results and then present those results, I think, is actually a very interesting design problem.” – Bob Mason (18:40)
- “I think initially, a lot of early AI startups just kind of assumed that customers would be patient and let the system run, [waiting] 3, 6, 9, 12 months [to get this] magical ROI, and that’s just not how people (buyers) operate.” – Bob Mason (21:00)
- “Re: platform plays: Obviously, you could still create a tremendous platform that’s very broad, but we think if you focus on the business problem of that particular vertical or domain, that actually creates a really powerful wedge so you can increase your value proposition. You could always increase the breadth of a platform over time. But if you’re not solving that intrinsic problem at the very beginning, you may never get the chance to survive.” – Bob Mason (28:24)
Links
- Argon Ventures: https://argon.vc/
- LinkedIn: https://www.linkedin.com/in/robertmason/details/experience/
- Email: bob@argon.vc
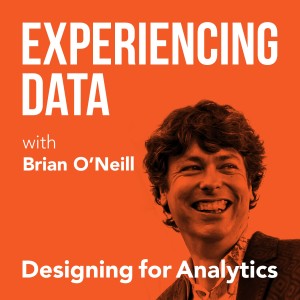
Tuesday Jan 10, 2023
Tuesday Jan 10, 2023
Today I’m chatting with Bruno Aziza, Head of Data & Analytics at Google Cloud. Bruno leads a team of outbound product managers in charge of BigQuery, Dataproc, Dataflow and Looker and we dive deep on what Bruno looks for in terms of skills for these leaders. Bruno describes the three patterns of operational alignment he’s observed in data product management, as well as why he feels ownership and customer obsession are two of the most important qualities a good product manager can have. Bruno and I also dive into how to effectively abstract the core problem you’re solving, as well as how to determine whether a problem might be solved in a better way.
Highlights / Skip to:
- Bruno introduces himself and explains how he created his “CarCast” podcast (00:45)
- Bruno describes his role at Google, the product managers he leads, and the specific Google Cloud products in his portfolio (02:36)
- What Bruno feels are the most important attributes to look for in a good data product manager (03:59)
- Bruno details how a good product manager focuses on not only the core problem, but how the problem is currently solved and whether or not that’s acceptable (07:20)
- What effective abstracting the problem looks like in Bruno’s view and why he positions product management as a way to help users move forward in their career (12:38)
- Why Bruno sees extracting value from data as the number one pain point for data teams and their respective companies (17:55)
- Bruno gives his definition of a data product (21:42)
- The three patterns Bruno has observed of operational alignment when it comes to data product management (27:57)
- Bruno explains the best practices he’s seen for cross-team goal setting and problem-framing (35:30)
Quotes from Today’s Episode
- “What’s happening in the industry is really interesting. For people that are running data teams today and listening to us, the makeup of their teams is starting to look more like what we do [in] product management.” — Bruno Aziza (04:29)
- “The problem is the problem, so focus on the problem, decompose the problem, look at the frictions that are acceptable, look at the frictions that are not acceptable, and look at how by assembling a solution, you can make it most seamless for the individual to go out and get the job done.” – Bruno Aziza (11:28)
- “As a product manager, yes, we’re in the business of software, but in fact, I think you’re in the career management business. Your job is to make sure that whatever your customer’s job is that you’re making it so much easier that they, in fact, get so much more done, and by doing so they will get promoted, get the next job.” – Bruno Aziza (15:41)
- “I think that is the task of any technology company, of any product manager that’s helping these technology companies: don’t be building a product that’s looking for a problem. Just start with the problem back and solution from that. Just make sure you understand the problem very well.” (19:52)
- “If you’re a data product manager today, you look at your data estate and you ask yourself, ‘What am I building to save money? When am I building to make money?’ If you can do both, that’s absolutely awesome. And so, the data product is an asset that has been built repeatedly by a team and generates value out of data.” – Bruno Aziza (23:12)
- “[Machine learning is] hard because multiple teams have to work together, right? You got your business analyst over here, you’ve got your data scientists over there, they’re not even the same team. And so, sometimes you’re struggling with just the human aspect of it.” (30:30)
- “As a data leader, an IT leader, you got to think about those soft ways to accomplish the stuff that’s binary, that’s the hard [stuff], right? I always joke, the hard stuff is the soft stuff for people like us because we think about data, we think about logic, we think, ‘Okay if it makes sense, it will be implemented.’ For most of us, getting stuff done is through people. And people are emotional, how can you express the feeling of achieving that goal in emotional value?” – Bruno Aziza (37:36)
Links
- As referenced by Bruno, “Good Product Manager/Bad Product Manager”: https://a16z.com/2012/06/15/good-product-managerbad-product-manager/
- LinkedIn: https://www.linkedin.com/in/brunoaziza/
- Bruno’s Medium Article on Competing Against Luck by Clayton M. Christensen: https://brunoaziza.medium.com/competing-against-luck-3daeee1c45d4
- The Data CarCast on YouTube: https://www.youtube.com/playlist?list=PLRXGFo1urN648lrm8NOKXfrCHzvIHeYyw
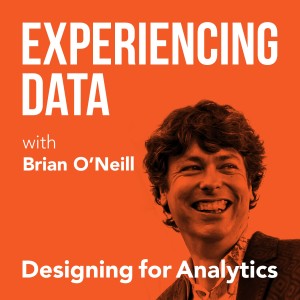
Tuesday Dec 27, 2022
Tuesday Dec 27, 2022
Today I’m chatting with returning guest Tom Davenport, who is a Distinguished Professor at Babson College, a Visiting Professor at Oxford, a Research Fellow at MIT, and a Senior Advisor to Deloitte’s AI practice. He is also the author of three new books (!) on AI and in this episode, we’re discussing the role of product orientation in enterprise data science teams, the skills required, what he’s seeing in the wild in terms of teams adopting this approach, and the value it can create. Back in episode 26, Tom was a guest on my show and he gave the data science/analytics industry an approximate “2 out of 10” rating in terms of its ability to generate value with data. So, naturally, I asked him for an update on that rating, and he kindly obliged. How are you all doing? Listen in to find out!
Highlights / Skip to:
- Tom provides an updated rating (between 1-10) as to how well he thinks data science and analytics teams are doing these days at creating economic value (00:44)
- Why Tom believes that “motivation is not enough for data science work” (03:06)
- Tom provides his definition of what data products are and some opinions on other industry definitions (04:22)
- How Tom views the rise of taking a product approach to data roles and why data products must be tied to value (07:55)
- Tom explains why he feels top down executive support is needed to drive a product orientation (11:51)
- Brian and Tom discuss how they feel companies should prioritize true data products versus more informal AI efforts (16:26)
- The trends Tom sees in the companies and teams that are implementing a data product orientation (19:18)
- Brian and Tom discuss the models they typically see for data teams and their key components (23:18)
- Tom explains the value and necessity of data product management (34:49)
- Tom describes his three new books (39:00)
Quotes from Today’s Episode
- “Data science in general, I think has been focused heavily on motivation to fit lines and curves to data points, and that particular motivation certainly isn’t enough in that even if you create a good model that fits the data, it doesn’t mean at all that is going to produce any economic value.” – Tom Davenport (03:05)
- “If data scientists don’t worry about deployment, then they’re not going to be in their jobs for terribly long because they’re not providing any value to their organizations.” – Tom Davenport (13:25)
- “Product also means you got to market this thing if it’s going to be successful. You just can’t assume because it’s a brilliant algorithm with capturing a lot of area under the curve that it’s somehow going to be great for your company.” – Tom Davenport (19:04)
- “[PM is] a hard thing, even for people in non-technical roles, because product management has always been a sort of ‘minister without portfolio’ sort of job, and you know, influence without formal authority, where you are responsible for a lot of things happening, but the people don’t report to you, generally.” – Tom Davenport (22:03)
- “This collaboration between a human being making a decision and an AI system that might in some cases come up with a different decision but can’t explain itself, that’s a really tough thing to do [well].” – Tom Davenport (28:04)
- “This idea that we’re going to use externally-sourced systems for ML is not likely to succeed in many cases because, you know, those vendors didn’t work closely with everybody in your organization” – Tom Davenport (30:21)
- “I think it’s unlikely that [organizational gaps] are going to be successfully addressed by merging everybody together in one organization. I think that’s what product managers do is they try to address those gaps in the organization and develop a process that makes coordination at least possible, if not true, all the time.” – Tom Davenport (36:49)
Links
- Tom’s LinkedIn: https://www.linkedin.com/in/davenporttom/
- Tom’s Twitter: https://twitter.com/tdav
- All-in On AI by Thomas Davenport & Nitin Mittal, 2023
- Working With AI by Thomas Davenport & Stephen Miller, 2022
- Advanced Introduction to AI in Healthcare by Thomas Davenport, John Glaser, & Elizabeth Gardner, 2022
- Competing On Analytics by Thomas Davenport & Jeanne G. Harris, 2007
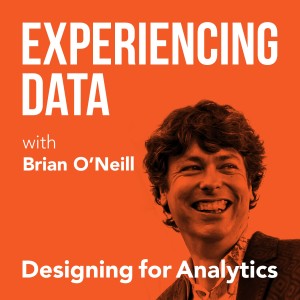
Tuesday Dec 13, 2022
Tuesday Dec 13, 2022
Today I’m chatting with former-analyst-turned-design-educator Jeremy Utley of the Stanford d.school and co-author of Ideaflow. Jeremy reveals the psychology behind great innovation, and the importance of creating psychological safety for a team to generate what they may view as bad ideas. Jeremy speaks to the critical collision of unrelated frames of reference when problem-solving, as well as why creativity is actually more of a numbers game than awaiting that singular stroke of genius. Listen as Jeremy gives real-world examples of how to practice and measure (!) your innovation efforts and apply them to data products.
Highlights/ Skip to:
- Jeremy explains the methodology of thinking he’s adopted after moving from highly analytical roles to the role he’s in now (01:38)
- The approach Jeremy takes to the existential challenge of balancing innovation with efficiency (03:54)
- Brian shares a story of a creative breakthrough he had recently and Jeremy uses that to highlight how innovation often comes in a way contrary to normalcy and professionalism (09:37)
- Why Jeremy feels innovation and creativity demand multiple attempts at finding solutions (16:13)
- How to take a innovation-forward approach like the ones Jeremy has described when working on internal tool development (19:33)
- Jeremy’s advice for accelerating working through bad ideas to get to the good ideas (25:18)
- The approach Jeremy takes to generate a large volume of ideas, rather than focusing only on “good” ideas, including a real-life example (31:54)
- Jeremy’s beliefs on the importance of creating psychological safety to promote innovation and creative problem-solving (35:11)
Quotes from Today’s Episode
- “I’m in spreadsheets every day to this day, but I recognize that there’s a time and place when that’s the tool that’s needed, and then specifically, there’s a time and a place where that’s not going to help me and the answer is not going to be found in the spreadsheet.” – Jeremy Utley (03:13)
- “There’s the question of, ‘Are we doing it right?’ And then there’s a different question, which is, ‘Are we doing the right “it”?’ And I think a lot of us tend to fixate on, ‘Are we doing it right?’ And we have an ability to perfectly optimize that what should not be done.” – Jeremy Utley (05:05)
- “I think a vendetta that I have is against this wrong placement of—this exaltation of efficiency is the end-all, be-all. Innovation is not efficient. And the question is not how can I be efficient. It’s what is effective. And effectiveness, oftentimes when it comes to innovation and breaking through, doesn’t feel efficient.” – Jeremy Utley (09:17)
- “The way the brain works, we actually understand it. The way breakthroughs work we actually understand them. The difficulty is it challenges our definitions of efficiency and professionalism and all of these things.” – Jeremy Utley (15:13)
- “What’s the a priori probability that any solution is the right solution? Or any idea is a good idea? It’s exceptionally low. You have to be exceptionally arrogant to think that most of your ideas are good. They’re not. That’s fine, we don’t mind because then what’s efficient is actually to generate a lot.” – Jeremy Utley (26:20)
- “If you don’t learn that nothing happens when the ball hits the floor, you can never learn how to juggle. And to me, it’s a really good metaphor. The teams that don’t learn nothing happens when they have a bad idea. Literally, the world does not end. They don’t get fired. They don’t get ridiculed. Now, if they do get fired or ridiculed, that’s a leadership problem.” – Jeremy Utley (35:59)
- [The following] is an essential question for a team leader to ask. Do people on my team have the freedom, at least with me, to share what they truly fear could be an incredibly stupid idea?” – Jeremy Utley (41:52)
Links
- Ideaflow: https://www.amazon.com/Ideaflow-Only-Business-Metric-Matters-ebook/dp/B09R6M3292
- Ideaflow website: https://ideaflow.design
- Personal webpage: https://jeremyutley.design
- LinkedIn: https://www.linkedin.com/in/jeremyutley/
- Twitter: https://twitter.com/jeremyutley/
- Brian’s musical arrangement of Gershwin’s “Prelude for Piano IIfeaturing the Siamese Cat Song” performed by Mr. Ho’s Orchestrotica - listen on Spotify
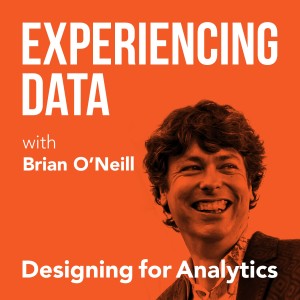
Tuesday Nov 29, 2022
Tuesday Nov 29, 2022
Today I’m discussing something we’ve been talking about a lot on the podcast recently - the definition of a “data product.” While my definition is still a work in progress, I think it’s worth putting out into the world at this point to get more feedback. In addition to sharing my definition of data products (as defined the “producty way”), on today’s episode definition, I also discuss some of the non-technical skills that data product managers (DPMs) in the ML and AI space need if they want to achieve good user adoption of their solutions. I’ll also share my thoughts on whether data scientists can make good data product managers, what a DPM can do to better understand your users and stakeholders, and how product and UX design factors into this role.
Highlights/ Skip to:
- I introduce my reasons for sharing my definition of a data product (0:46)
- My definition of data product (7:26)
- Thinking the “producty” way (8:14)
- My thoughts on necessary skills for data PMs (in particular, AI & machine learning product management) (12:21)
- How data scientists can become good data product managers (DPMs) by taking off the data science hat (13:42)
- Understanding the role of UX design within the context of DPM (16:37)
- Crafting your sales and marketing strategies to emphasize the value of your product to the people who can use or purchase it (23:07)
- How to build a team that will help you increase adoption of your data product (30:01)
- How to build relationships with stakeholders/customers that allow you to find the right solutions for them (33:47)
- Letting go of a technical identity to develop a new identity as a DPM who can lead a team to build a product that actually gets used (36:32)
Quotes from Today’s Episode
- “This is what’s missing in some of the other definitions that I see around data products [...] they’re not talking about it from the customer of the data product lens. And that orientation sums up all of the work that I’m doing and trying to get you to do as well, which is to put the people at the center of the work that you’re doing and not the data science, engineering, tech, or design. I want you to put the people at the center.” (6:12)
- “A data product is a data-driven, end-to-end, human-in-the-loop decision support solution that’s so valuable, users would potentially pay to use it.” (7:26)
- “I want to plunge all the way in and say, ‘if you want to do this kind of work, then you need to be thinking the product-y way.’ And this means inherently letting go of some of the data science-y way of thinking and the data-first kinds of ways of thinking.” (11:46)
- “I’ve read in a few places that data scientists don’t make for good data product managers. [While it may be true that they’re more introverted,] I don’t think that necessarily means that there’s an inherent problem with data scientists becoming good data product managers. I think the main challenge will be—and this is the same thing for almost any career transitioning into product management—is knowing when to let go of your former identity and wear the right hat at the right time.” (14:24)
- “Make better things for people that will improve their life and their outcomes and the business value will follow if you’ve properly aligned those two things together.” (17:21)
- “The big message here is this: there is always a design and experience, whether it is an API, or a platform, a dashboard, a full application, etc. Since there are no null design choices, how much are you going to intentionally shape that UX, or just pray that it comes out good on the other end? Prayer is not really a reliable strategy. If you want to routinely do this work right, you need to put intention behind it.” (22:33)
- “Relationship building is a must, and this is where applying user experience research can be very useful—not just for users, but also with stakeholders. It’s learning how to ask really good questions and learning the feelings, emotions, and reasons why people ask your team to build the thing that they’ve asked for. Learning how to dig into that is really important.” (26:26)
Links
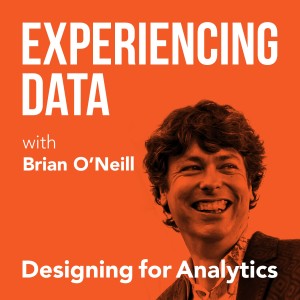
Tuesday Nov 15, 2022
Tuesday Nov 15, 2022
Today I’m chatting with Indi Young, independent qualitative data scientist and author of Time to Listen. Indi explains how it is possible to gather and analyze qualitative data in a way that is meaningful to the desired future state of your users, and that learning how to listen and not just interview users is much like learning to ride a bicycle. Listen (!) to find out why pushing back is a necessary part of the design research process, how to build an internal sensor that allows you to truly uncover the nuggets of information that are critical to your projects, and the importance of understanding thought processes to prevent harmful outcomes.
Highlights/ Skip to:
- Indi introduces her perspective on analyzing qualitative data sets (00:51)
- Indi’s motivation for working in design research and the importance of being able to capture and understand patterns to prevent harmful outcomes (05:09)
- The process Indi goes through for problem framing and understanding a user’s desired future state (11:11)
- Indi explains how to listen effectively in order to understand the thinking style of potential end users (15:42)
- Why Indi feels pushing back on problems within projects is a vital part of taking responsibility and her recommendations for doing so effectively (21:45)
- The importance Indi sees in building up a sensor in order to be able to detect nuggets clients give you for their upcoming projects (28:25)
- The difference in techniques Indi observes between an interview, a listening session, and a survey (33:13)
- Indi describes her published books and reveals which one she’d recommend listeners start with (37:34)
Quotes from Today’s Episode
- “A lot of qualitative data is not trusted, mainly because the people who are doing the not trusting have encountered bad qualitative data.” — Indi Young (03:23)
- “When you’re learning to ride a bike, when you’re learning to decide what knowledge is needed, you’re probably going to burn through a bunch of money-making knowledge that never gets used. So, that’s when you start to learn, ‘I need to frame this better, and to frame it, I can’t do it by myself.’” – Indi Young (11:57)
- “What you want to do is get beyond the exterior and get to the interior, which is where somebody tells you what actually went through their mind when they did that thing in the past, not what’s going through their mind right now. And it’s that’s a very important distinction.” – Indi Young (20:28)
- “Re: dealing with stakeholders: You’re not doing your job if you don’t push back. You built up a lot of experience, you got hired, they hired you and your thinking and your experience, and if what went through your mind is, like, ‘This is wrong,’ but you don’t act on it, then they should not pay you a salary.” – Indi Young (22:45)
- “I’ve seen a lot of people leave their perfectly promising career because it was too hard to get to the point of accepting that you have to network, that I’m not going to be that one-in-a-million person who’s the brilliant person with a brilliant idea and get my just rewards that way.” – Indi Young (25:13)
- “What’s really interesting about a listening session is that it doesn’t—aside from building this sensor and learning what the techniques are for helping a person get to their interior cognition rather than that exterior … to get past that into the inner thinking, the emotional reactions, and the guiding principles, aside from the sensor and those techniques, there’s not much to it.” – Indi Young (32:45)
- “And once you start building that [sensor], and this idea of just having one generative question about the purpose—because the whole thing is framed by the purpose—there you go. Get started. You have to practice. So, it’s like riding a bike. Go for it. You won’t have those sensors at first, but you’ll start to learn how to build them.” – Indi Young (36:41)
Links Referenced:
- Time to Listen: https://www.amazon.com/Time-Listen-Invention-Inclusion-Assumptions/dp/1944627111
- Mental Models: https://www.amazon.com/Mental-Models-Aligning-Strategy-Behavior/dp/1933820063
- Practical Empathy: https://www.amazon.com/Practical-Empathy-Collaboration-Creativity-Your/dp/1933820489
- indiyoung.com: https://indiyoung.com
- LinkedIn: https://www.linkedin.com/in/indiyoung/
- Instagram: https://www.instagram.com/indiyoung_/
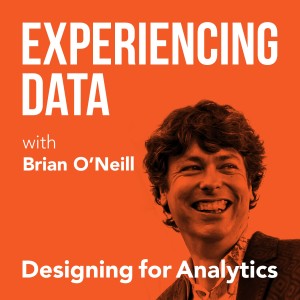
Tuesday Nov 01, 2022
Tuesday Nov 01, 2022
Today I’m chatting with Eugenio Zuccarelli, Research Scientist at MIT Media Lab and Manager of Data Science at CVS. Eugenio explains how he has created multiple algorithms designed to help shape decisions made in life or death situations, such as pediatric cardiac surgery and during the COVID-19 pandemic. Eugenio shared the lessons he’s learned on how to build trust in data when the stakes are life and death. Listen and learn how culture can affect adoption of decision support and ML tools, the impact delivery of information has on the user's ability to understand and use data, and why Eugenio feels that design is more important than the inner workings of ML algorithms.
Highlights/ Skip to:
- Eugenio explains why he decided to work on machine learning models for cardiologists and healthcare workers involved in the COVID-19 pandemic (01:53)
- The workflow surgeons would use when incorporating the predictive algorithm and application Eugenio helped develop (04:12)
- The question Eugenio’s predictive algorithm helps surgeons answer when evaluating whether to use various pediatric cardiac surgical procedures (06:37)
- The path Eugenio took to build trust with experienced surgeons and drive product adoption and the role of UX (09:42)
- Eugenio’s approach to identifying key problems and finding solutions using data (14:50)
- How Eugenio has tracked value delivery and adoption success for a tool that relies on more than just accurate data & predictions, but also surgical skill and patient case complexity (22:26)
- The design process Eugenio started early on to optimize user experience and adoption (28:40)
- Eugenio’s key takeaways from a different project that helped government agencies predict what resources would be needed in which areas during the COVID-19 pandemic (34:45)
Quotes from Today’s Episode
- “So many people today are developing machine-learning models, but I truly find the most difficult parts to be basically everything around machine learning … culture, people, stakeholders, products, and so on.” — Eugenio Zuccarelli (01:56)
- “Developing machine-learning components, clean data, developing the machine-learning pipeline, those were the easy steps. The difficult ones who are gaining trust, as you said, developing something that was useful. And talking about trust, it’s especially tricky in the healthcare industry.” — Eugenio Zuccarelli (10:42)
- “Because this tennis match, this ping-pong match between what can be done and what’s [the] problem [...] thankfully, we know, of course, it is not really the route to go. We don’t want to develop technology for the sake of it.” — Eugenio Zuccarelli (14:49)
- “We put so much effort on the machine-learning side and then the user experience is so key, it’s probably even more important than the inner workings.” — Eugenio Zuccarelli (29:22)
- “It was interesting to see exactly how the doctor is really focused on their job and doing it as well as they can, not really too interested in fancy [...] solutions, and so we were really able to not focus too much on appearance or fancy components, but more on usability and readability.” — Eugenio Zuccarelli (33:45)
- “People’s ability to trust data, and how this varies from a lot of different entities, organizations, countries, [etc.] This really makes everything tricky. And of course, when you have a pandemic, this acts as a catalyst and enhances all of these cultural components.” — Eugenio Zuccarelli (35:59)
- “I think [design success] boils down to delivery. You can package the same information in different ways [so that] it actually answers their questions in the ways that they’re familiar with.” — Eugenio Zuccarelli (37:42)
Links
- LinkedIn: https://www.linkedin.com/in/jayzuccarelli
- Twitter: twitter.com/jayzuccarelli
- Personal website: https://eugeniozuccarelli.com
- Medium: jayzuccarelli.medium.com
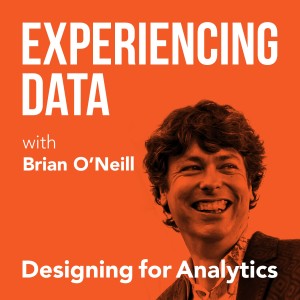
Tuesday Oct 18, 2022
Tuesday Oct 18, 2022
Today I’m chatting with Iván Herrero Bartolomé, Chief Data Officer at Grupo Intercorp. Iván describes how he was prompted to write his new article in CDO Magazine, “CDOs, Let’s Get Out of Our Comfort Zone” as he recognized the importance of driving cultural change within organizations in order to optimize the use of data. Listen in to find out how Iván is leveraging the role of the analytics translator to drive this cultural shift, as well as the challenges and benefits he sees data leaders encounter as they move from tactical to strategic objectives. Iván also reveals the number one piece of advice he’d give CDOs who are struggling with adoption.
Highlights / Skip to:
- Iván explains what prompted him to write his new article, “CDOs, Let’s Get Out of Our Comfort Zone” (01:08)
- What Iván feels is necessary for data leaders to close the gap between data and the rest of the business and why (03:44)
- Iván dives into who he feels really owns delivery of value when taking on new data science and analytics projects (09:50)
- How Iván’s team went from managing technical projects that often didn’t make it to production to working on strategic projects that almost always make it to production (13:06)
- The framework Iván has developed to upskill technical and business roles to be effective data / analytics translators (16:32)
- The challenge Iván sees data leaders face as they move from setting and measuring tactical goals to moving towards strategic goals and initiatives (24:12)
- Iván explains how the C-Suite’s attitude impacts the cross-functional role of data & analytics leadership (28:55)
- The number one piece of advice Iván would give new CDO’s struggling with low adoption of their data products and solutions (31:45)
Quotes from Today’s Episode
- “We’re going to do all our best to ensure that [...] everything that is expected from us is done in the best possible way. But that’s not going to be enough. We need a sponsorship and we need someone accountable for the project and someone who will be pushing and enabling the use of the solution once we are gone. Because we cannot stay forever in every company.” – Iván Herrero Bartolomé (10:52)
- “We are trying to upskill people from the business to become data translators, but that’s going to take time. Especially what we try to do is to take product owners and give them a high-level immersion on the state-of-the-art and the possibilities that data analytics bring to the table. But as we can’t rely on our companies having this kind of talent and these data translators, they are one of the profiles that we bring in for every project that we work on.” – Iván Herrero Bartolomé (13:51)
- “There’s a lot to do, not just between data and analytics and the other areas of the company, but aligning the incentives of all the organization towards the same goals in a way that there’s no friction between the goals of the different areas, the people, [...] and the final goals of the organization. – Iván Herrero Bartolomé (23:13)
- “Deciding which goals are you going to be co-responsible for, I think that is a sophisticated process that it’s not mastered by many companies nowadays. That probably is one of the main blockers keeping data analytics areas working far from their business counterparts” – Iván Herrero Bartolomé (26:05)
- “When the C-suite looks at data and analytics, if they think these are just technical skills, then the data analytics team are just going to behave as technical people. And many, many data analytics teams are set up as part of the IT organization. So, I think it all begins somehow with how the C-suite of our companies look at us.” – Iván Herrero Bartolomé (28:55)
- “For me, [digital] means much more than the technical development of solutions; it should also be part of the transformation of the company, both in how companies develop relationships with their customers, but also inside how every process in the companies becomes more nimble and can react faster to the changes in the market.” – Iván Herrero Bartolomé (30:49)
- “When you feel that everyone else not doing what you think they should be doing, think twice about whether it is they who are not doing what they should be doing or if it’s something that you are not doing properly.” – Iván Herrero Bartolomé (31:45)
Links
- “CDOs, Let’s Get Out of Our Comfort Zone”: https://www.cdomagazine.tech/cdo_magazine/topics/opinion/cdos-lets-get-out-of-our-comfort-zone/article_dce87fce-2479-11ed-a0f4-03b95765b4dc.html
- LinkedIn: https://www.linkedin.com/in/ivan-herrero-bartolome/
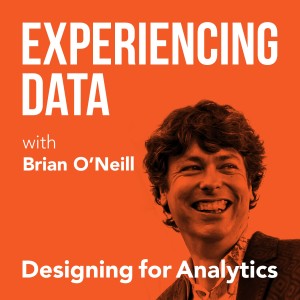
Tuesday Oct 04, 2022
Tuesday Oct 04, 2022
Today I’m chatting with Katy Pusch, Senior Director of Product and Integration for Cox2M. Katy describes the lessons she’s learned around making sure that the “juice is always worth the squeeze” for new users to adopt data solutions into their workflow. She also explains the methodologies she’d recommend to data & analytics professionals to ensure their IOT and data products are widely adopted. Listen in to find out why this former analyst turned data product leader feels it’s crucial to focus on more than just delivering data or AI solutions, and how spending more time upfront performing qualitative research on users can wind up being more efficient in the long run than jumping straight into development.
Highlights/ Skip to:
- What Katy does at Cox2M, and why the data product manager role is so hard to define (01:07)
- Defining the value of the data in workflows and how that’s approached at Cox2M (03:13)
- Who buys from Cox2M and the customer problems that Katy’s product solves (05:57)
- How Katy approaches the zero-to-one process of taking IOT sensor data and turning it into a customer experience that provides a valuable solution (08:00)
- What Katy feels best motivates the adoption of a new solution for users (13:21)
- Katy describes how she spends more time upfront before development to ensure she’s solving the right problems for users (16:13)
- Katy’s views on the importance of data science & analytics pros being able to communicate in the language of their audience (20:47)
- The differences Katy sees between designing data products for sophisticated data users vs a broader audience (24:13)
- The methods Katy uses to effectively perform qualitative research and her triangulation method to surface the real needs of end users (27:29)
- Katy’s views on the most valuable skills for future data product managers (35:24)
Quotes from Today’s Episode
- “I’ve had the opportunity to get a little bit closer to our customers than I was in the beginning parts of my tenure here at Cox2M. And it’s just like a SaaS product in the sense that the quality of your data is still dependent on your customers’ workflows and their ability to engage in workflows that supply accurate data. And it’s been a little bit enlightening to realize that the same is true for IoT.” – Katy Pusch (02:11)
- “Providing insights to executives that are [simply] interesting is not really very impactful. You want to provide things that are actionable and that drive the business forward.” – Katy Pusch (4:43)
- “So, there’s one side of it, which is [the] happy path: figure out a way to embed your product in the customer’s existing workflow. That’s where the most success happens. But in the situation we find ourselves in right now with [this IoT solution], we do have to ask them to change their workflow.”-- Katy Pusch (12:46)
- “And the way to communicate [the insight to other stakeholders] is not with being more precise with your numbers [or adding] statistics. It’s just to communicate the output of your analysis more clearly to the person who needs to be able to make a decision.” -- Katy Pusch (23:15)
- “You have to define ‘What decision is my user making on a repeated basis that is worth building something that it does automatically?’ And so, you say, ‘What are the questions that my user needs answers to on a repeated basis?’ … At its essence, you’re answering three or four questions for that user [that] have to be the most important [...] questions for your user to add value. And that can be a difficult thing to derive with confidence.” – Katy Pusch (25:55)
- “The piece of workflow [on the IOT side] that’s really impactful there is we’re asking for an even higher degree of change management in that case because we’re asking them to attach this device to their vehicle, and then detach it at a different point in time and there’s a procedure in the solution to allow for that, but someone at the dealership has to engage in that process. So, there’s a change management in the workflow that the juice has to be worth the squeeze to encourage a customer to embark in that journey with you.” – Katy Pusch (12:08)
- “Finding people in your organization who have the appetite to be cross-functionally educated, particularly in a data arena, is very important [to] help close some of those communication gaps.” – Katy Pusch (37:03)