
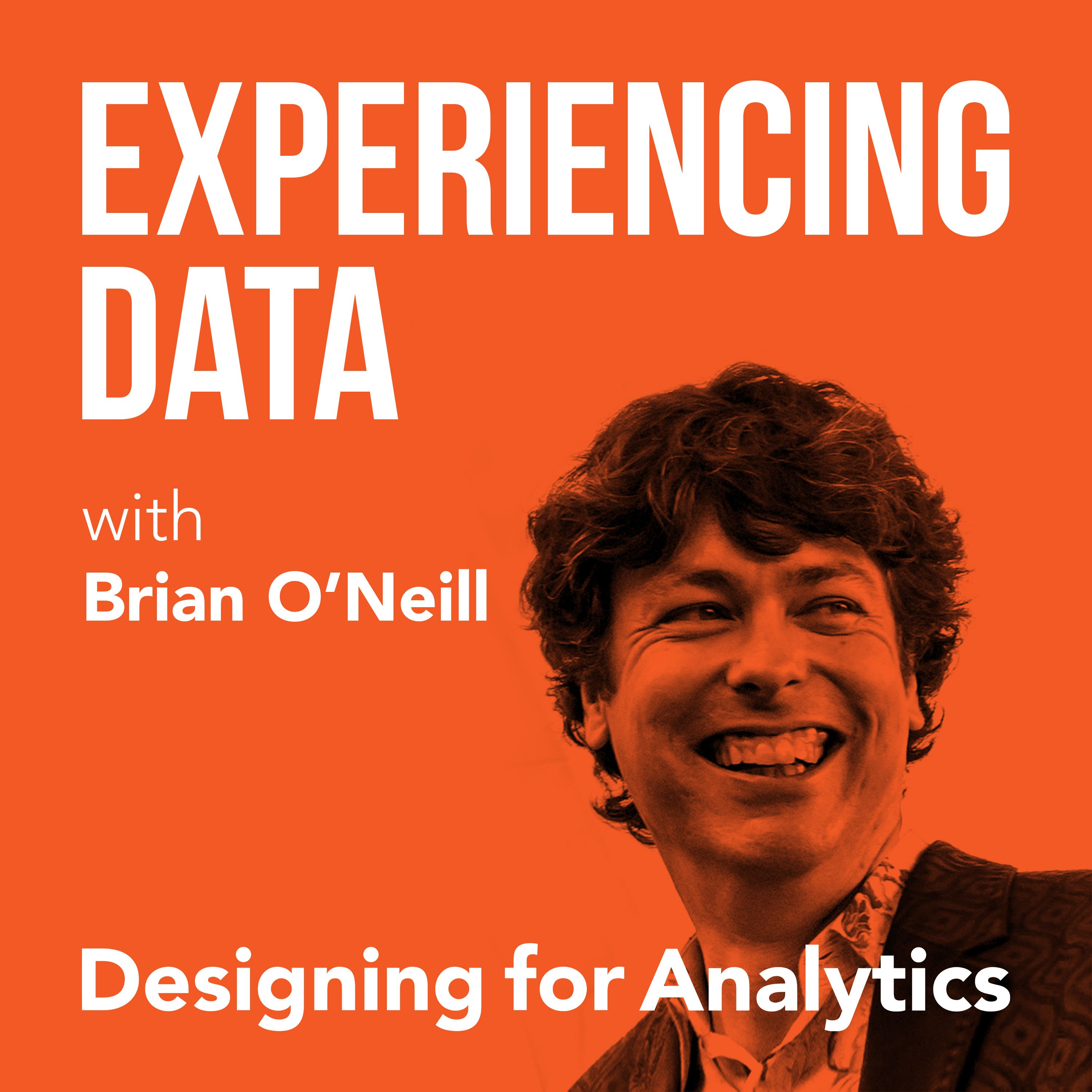
137.1K
Downloads
169
Episodes
Is the value of your enterprise analytics SAAS or AI product not obvious through it’s UI/UX? Got the data and ML models right...but user adoption of your dashboards and UI isn’t what you hoped it would be? While it is easier than ever to create AI and analytics solutions from a technology perspective, do you find as a founder or product leader that getting users to use and buyers to buy seems harder than it should be? If you lead an internal enterprise data team, have you heard that a ”data product” approach can help—but you’re concerned it’s all hype? My name is Brian T. O’Neill, and on Experiencing Data—one of the top 2% of podcasts in the world—I share the stories of leaders who are leveraging product and UX design to make SAAS analytics, AI applications, and internal data products indispensable to their customers. After all, you can’t create business value with data if the humans in the loop can’t or won’t use your solutions. Every 2 weeks, I release interviews with experts and impressive people I’ve met who are doing interesting work at the intersection of enterprise software product management, UX design, AI and analytics—work that you need to hear about and from whom I hope you can borrow strategies. I also occasionally record solo episodes on applying UI/UX design strategies to data products—so you and your team can unlock financial value by making your users’ and customers’ lives better. Hashtag: #ExperiencingData. JOIN MY INSIGHTS LIST FOR 1-PAGE EPISODE SUMMARIES, TRANSCRIPTS, AND FREE UX STRATEGY TIPS https://designingforanalytics.com/ed ABOUT THE HOST, BRIAN T. O’NEILL: https://designingforanalytics.com/bio/
Episodes
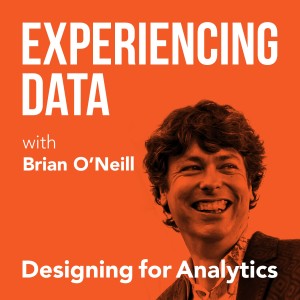
Tuesday Aug 23, 2022
098 - Why Emilie Schario Wants You to Run Your Data Team Like a Product Team
Tuesday Aug 23, 2022
Tuesday Aug 23, 2022
Today I’m chatting with Emilie Shario, a Data Strategist in Residence at Amplify Partners. Emilie thinks data teams should operate like product teams. But what led her to that conclusion, and how has she put the idea into practice? Emilie answers those questions and more, delving into what kind of pushback and hiccups someone can expect when switching from being data-driven to product-driven and sharing advice for data scientists and analytics leaders.
Highlights / Skip to:
- Answering the question “whose job is it” (5:18)
- Understanding and solving problems instead of just building features people ask for (9:05)
- Emilie explains what Amplify Partners is and talks about her work experience and how it fuels her perspectives on data teams (11:04)
- Emilie and I talk about the definition of data product (13:00)
- Emilie talks about her approach to building and training a data team (14:40)
- We talk about UX designers and how they fit into Emilie’s data teams (18:40)
- Emilie talks about the book and blog “Storytelling with Data” (21:00)
- We discuss the push back you can expect when trying to switch a team from being data driven to being product driven (23:18)
- What hiccups can people expect when switching to a product driven model (30:36)
- Emilie’s advice for data scientists and and analyst leaders (35:50)
- Emilie explains what Locally Optimistic is (37:34)
Quotes from Today’s Episode
- “Our thesis is…we need to understand the problems we’re solving before we start building solutions, instead of just building the things people are asking for.” — Emilie (2:23)
- “I’ve seen this approach of flipping the ask on its head—understanding the problem you’re trying to solve—work and be more successful at helping drive impact instead of just letting your data team fall into this widget builder service trap.” — Emilie (4:43)
- “If your answer to any problem to me is, ‘That’s not my job,’ then I don’t want you working for me because that’s not what we’re here for. Your job is whatever the problem in front of you that needs to be solved.” — Emilie (7:14)
- “I don’t care if you have all of the data in the world and the most talented machine learning engineers and you’ve got the ability to do the coolest new algorithm fancy thing. If it doesn’t drive business impact, it doesn’t matter.” — Emilie (7:52)
- “Data is not just a thing that anyone can do. It’s not just about throwing numbers in a spreadsheet anymore. It’s about driving business impact. But part of how we drive business impact with data is making it accessible. And accessible isn’t just giving people the numbers, it’s also communicating with it effectively, and UX is a huge piece of how we do that.” — Emilie (19:57)
- “There are no null choices in design. Someone is deciding what some other human—a customer, a client, an internal stakeholder—is going to use, whether it’s a React app, or a Power BI dashboard, or a spreadsheet dump, or whatever it is, right? There will be an experience that is created, whether it is intentionally created or not.” — Brian (20:28)
- “People will think design is just putting in colors that match together, like, or spinning the color wheel and seeing what lands. You know, there’s so much more to it. And it is an expertise; it is a domain that you have to develop.” — Emilie (34:58)
Links Referenced:
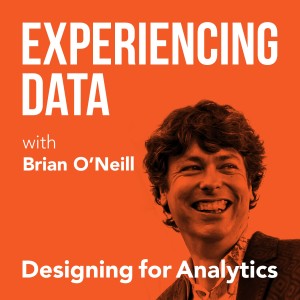
Tuesday Aug 09, 2022
Tuesday Aug 09, 2022
Today, I chat with Manav Misra, Chief Data and Analytics Officer at Regions Bank. I begin by asking Manav what it was like to come in and implement a user-focused mentality at Regions, driven by his experience in the software industry. Manav details his approach, which included developing a new data product partner role and using effective communication to gradually gain trust and cooperation from all the players on his team.
Manav then talks about how, over time, he solidified a formal framework for his team to be trained to use this approach and how his hiring is influenced by a product orientation. We also discuss his definition of data product at Regions, which I find to be one of the best I’ve heard to date. Today, Region Bank’s data products are delivering tens of millions of dollars in additional revenue to the bank. Given those results, I also dig into the role of design and designers to better understand who is actually doing the designing of Regions’ data products to make them so successful. Later, I ask Manav what it’s like when designers and data professionals work on the same team and how UX and data visualization design are handled at the bank.
Towards the end, Manav shares what he has learned from his time at Regions and what he would implement in a new organization if starting over. He also expounds on the importance of empowering his team to ask customers the right questions and how a true client/stakeholder partnership has led to Manav’s most successful data products.
Highlights / Skip to:
- Brief history of decision science and how it influenced the way data science and analytics work has been done (and unfortunately still is in many orgs) (1:47)
- Manav’s philosophy and methods for changing the data science culture at Regions Bank to being product and user-driven (5:19)
- Manav talks about the size of his team and the data product role within the team as well as what he had to do to convince leadership to buy in to the necessity of the data product partner role (10:54)
- Quantifying and measuring the value of data products at Regions and some of his results (which include tens of millions of dollars in additional revenue) (13:05)
- What’s a “data product” at Regions? Manav shares his definition (13:44)
- Who does the designing of data products at Regions? (17:00)
- The challenges and benefits of having a team comprised of both designers and data scientists (20:10)
- Lessons Manav has learned from building his team and culture at Regions (23:09)
- How Manav coaches his team and gives them the confidence to ask the right questions (27:17)
- How true partnership has led to Manav’s most successful data products (31:46)
Quotes from Today’s Episode
- Re: how traditional, non-product oriented enterprises do data work: “As younger people come out of data science programs…that [old] culture is changing. The folks coming into this world now are looking to make an impact and then they want to see what this can do in the real world.” — Manav
- On the role of the Data Product Partner: “We brought in people that had both business knowledge as well as the technical knowledge, so with a combination of both they could talk to the ‘Internal customers,’ of our data products, but they could also talk to the data scientists and our developers and communicate in both directions in order to form that bridge between the two.” — Manav
- “There are products that are delivering tens of millions of dollars in terms of additional revenue, or stopping fraud, or any of those kinds of things that the products are designed to address, they’re delivering and over-delivering on the business cases that we created.” — Manav
- “The way we define a data product is this: an end-to-end software solution to a problem that the business has. It leverages data and advanced analytics heavily in order to deliver that solution.” — Manav
- “The deployment and operationalization is simply part of the solution. They are not something that we do after; they’re something that we design in from the start of the solution.” — Brian
- “Design is a team sport. And even if you don’t have a titled designer doing the work, if someone is going to use the solution that you made, whether it’s a dashboard, or report, or an email, or notification, or an application, or whatever, there is a design, whether you put intention behind it or not.” — Brian
- “As you look at interactive components in your data product, which are, you know, allowing people to ask questions and then get answers, you really have to think through what that interaction will look like, what’s the best way for them to get to the right answers and be able to use that in their decision-making.” — Manav
- “I have really instilled in my team that tools will come and go, technologies will come and go, [and so] you’ll have to have that mindset of constantly learning new things, being able to adapt and take on new ideas and incorporate them in how we do things.” — Manav
Links
- Regions Bank: https://www.regions.com/
- LinkedIn: https://www.linkedin.com/in/manavmisra/
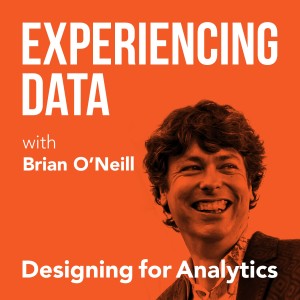
Tuesday Jul 26, 2022
Tuesday Jul 26, 2022
Today I chat with Chad Sanderson, Head of Product for Convoy’s data platform. I begin by having Chad explain why he calls himself a “data UX champion” and what inspired his interest in UX. Coming from a non-UX background, Chad explains how he came to develop a strategy for addressing the UX pain points at Convoy—a digital freight network. They “use technology to make freight more efficient, reducing costs for some of the nation’s largest brands, increasing earnings for carriers, and eliminating carbon emissions from our planet.” We also get into the metrics of success that Convoy uses to measure UX and why Chad is so heavily focused on user workflow when making the platform user-centered.
Later, Chad shares his definition of a data product, and how his experience with building software products has overlapped with data products. He also shares what he thinks is different about creating data products vs. traditional software products. Chad then explains Convoy’s approach to prototyping and the value of partnering with users in the design process. We wrap up by discussing how UX work gets accomplished on Chad’s team, given it doesn’t include any titled UX professionals.
Highlights:
- Chad explains how he became a data UX champion and what prompted him to care about UX (1:23)
- Chad talks about his strategy for beginning to address the UX issues at Convoy (4:42)
- How Convoy measures UX improvement (9:19)
- Chad talks about troubleshooting user workflows and it’s relevance to design (15:28)
- Chad explains what Convoy is and the makeup of his data platform team (21:00)
- What is a data product? Chad gives his definition and the similarities and differences between building software versus data products (23:21)
- Chad talks about using low fidelity work and prototypes to optimize solutions and resources in the long run (27:49)
- We talk about the value of partnering with users in the design process (30:37)
- Chad talks about the distribution of UX labor on his team (32:15)
Quotes from Today’s Episode
Re: user research: "The best content that you get from people is when they are really thinking about what to say next; you sort of get into a free-flowing exchange of ideas. So it’s important to find the topic where someone can just talk at length without really filtering themselves. And I find a good place to start with that is to just talk about their problems. What are the painful things that you’ve experienced in data in the last month or in the last week?" - Chad
Re: UX research: "I often recommend asking users to show you something they were working on recently, particularly when they were having a problem accomplishing their goal. It’s a really good way to surface UX issues because the frustration is probably fresh." - Brian
Re: user feedback, “One of the really great pieces of advice that I got is, if you’re getting a lot of negative feedback, this is actually a sign that people care. And if people care about what you’ve built, then it’s better than overbuilding from the beginning.” - Chad
“What we found [in our research around workflow], though, sometimes counterintuitively, is that the steps that are the easiest and simplest for a customer to do that I think most people would look at and say, ‘Okay, it’s pretty low ROI to invest in some automated solution or a product in this space,’ are sometimes the most important things that you can [address in your data product] because of the impacts that it has downstream.” - Chad
Re: user feedback, “The amazing thing about building data products, and I guess any internal products is that 100% of your customers sit ten feet away from you. [...] When you can talk to 100% of [your users], you are truly going to understand [...] every single persona. And that is tremendously effective for creating compelling narratives about why we need to build a particular thing.” - Chad
“If we can get people to really believe that this data product is going to solve the problem, then usually, we like to turn those people into advocates and evangelists within the company, and part of their job is to go out and convince other people about why this thing can solve the problem.” - Chad
Links:
- Convoy: https://convoy.com/
- Chad on LinkedIn: https://www.linkedin.com/in/chad-sanderson/
- Chad’s Data Products newsletter: https://dataproducts.substack.com
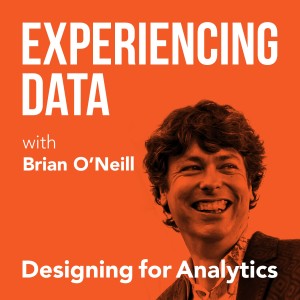
Tuesday Jul 12, 2022
Tuesday Jul 12, 2022
Today I am bringing you a recording of a live interview I did at the TDWI Munich conference for data leaders, and this episode is a bit unique as I’m in the “guest” seat being interviewed by the VP of TDWI Europe, Christoph Kreutz.
Christoph wanted me to explain the new workshop I was giving later that day, which focuses on helping leaders increase user adoption of data products through design. In our chat, I explained the three main areas I pulled out of my full 4-week seminar to create this new ½-day workshop as well as the hands-on practice that participants would be engaging in. The three focal points for the workshop were: measuring usability via usability studies, identifying the unarticulated needs of stakeholders and users, and sketching in low fidelity to avoid over committing to solutions that users won’t value.
Christoph also asks about the format of the workshop, and I explain how I believe data leaders will best learn design by doing it. As such, the new workshop was designed to use small group activities, role-playing scenarios, peer review…and minimal lecture! After discussing the differences between the abbreviated workshop and my full 4-week seminar, we talk about my consulting and training business “Designing for Analytics,” and conclude with a fun conversation about music and my other career as a professional musician.
In a hurry? Skip to:
- I summarize the new workshop version of “Designing Human-Centered Data Products” I was premiering at TDWI (4:18)
- We talk about the format of my workshop (7:32)
- Christoph and I discuss future opportunities for people to participate in this workshop (9:37)
- I explain the format of the main 8-week seminar versus the new half-day workshop (10:14)
- We talk about one on one coaching (12:22)
- I discuss my background, including my formal music training and my other career as a professional musician (14:03)
Quotes from Today’s Episode
- “We spend a lot of time building outputs and infrastructure and pipelines and data engineering and generating stuff, but not always generating outcomes. Users only care about how does this make my life better, my job better, my job easier? How do I look better? How do I get a promotion? How do I make the company more money? Whatever those goals are. And there’s a gap there sometimes, between the things that we ship and delivering these outcomes.” (4:36)
- “In order to run a usability study on a data product, you have to come up with some type of learning goals and some kind of scenarios that you’re going to give to a user and ask them to go show me how you would do x using the data thing that we built for you.” (5:54)
- “The reality is most data users and stakeholders aren’t designers and they’re not thinking about the user’s workflow and how a solution fits into their job. They don’t have that context. So, how do we get the really important requirements out of a user or stakeholder’s head? I teach techniques from qualitative UX interviewing, sales, and even hostage negotiation to get unarticulated needs out of people’s head.” (6:41)
- “How do we work in low fidelity to get data leaders on the same page with a stakeholder or a user? How do we design with users instead of for them? Because most of the time, when we communicate visually, it starts to click (or you’ll know it’s not clicking!)” (7:05)
- “There’s no right or wrong [in the workshop]. [The workshop] is really about the practice of using these design methods and not the final output that comes out of the end of it.” (8:14)
- “You learn design by doing design so I really like to get data people going by trying it instead of talking about trying it. More design doing and less design thinking!” (8:40)
- “The tricky thing [for most of my training clients], [and perhaps this is true with any type of adult education] is, ‘Yeah, I get the concept of what Brian’s talking about, but, how do I apply these design techniques to my situation? I work in this really weird domain, or on this particularly hard data space.’ Working on an exercise or real project, together, in small groups, is how I like start to make the conceptual idea of design into a tangible tool for data leaders..” (12:26)
Links
- Brian’s training seminar
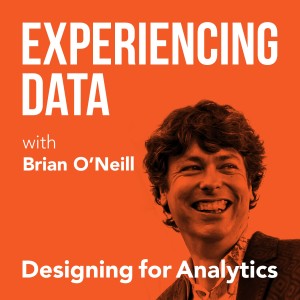
Tuesday Jun 28, 2022
Tuesday Jun 28, 2022
Today I sit down with Vijay Yadav, head of the data science team at Merck Manufacturing Division. Vijay begins by relating his own path to adopting a data product and UX-driven approach to applied data science, andour chat quickly turns to the ever-present challenge of user adoption. Vijay discusses his process of designing data products with customers, as well as the impact that building user trust has on delivering business value. We go on to talk about what metrics can be used to quantify adoption and downstream value, and then Vijay discusses the financial impact he has seen at Merck using this user-oriented perspective. While we didn’t see eye to eye on everything, Vijay was able to show how focusing on the last mile UX has had a multi-million dollar impact on Merck. The conversation concludes with Vijay’s words of advice for other data science directors looking to get started with a design and user-centered approach to building data products that achieve adoption and have measurable impact.
In our chat, we covered Vijay’s design process, metrics, business value, and more:
- Vijay shares how he came to approach data science with a data product management approach and how UX fits in (1:52)
- We discuss overcoming the challenge of user adoption by understanding user thinking and behavior (6:00)
- We talk about the potential problems and solutions when users self-diagnose their technology needs (10:23)
- Vijay delves into what his process of designing with a customer looks like (17:36)
- We discuss the impact “solving on the human level” has on delivering real world benefits and building user trust (21:57)
- Vijay talks about measuring user adoption and quantifying downstream value—and Brian discusses his concerns about tool usage metrics as means of doing this (25:35)
- Brian and Vijay discuss the multi-million dollar financial and business impact Vijay has seen at Merck using a more UX driven approach to data product development (31:45)
- Vijay shares insight on what steps a head of data science might wish to take to get started implementing a data product and UX approach to creating ML and analytics applications that actually get used (36:46)
Quotes from Today’s Episode
- “They will adopt your solution if you are giving them everything they need so they don’t have to go look for a workaround.” - Vijay (4:22)
- “It’s really important that you not only capture the requirements, you capture the thinking of the user, how the user will behave if they see a certain way, how they will navigate, things of that nature.” - Vijay (7:48)
- “When you’re developing a data product, you want to be making sure that you’re taking the holistic view of the problem that can be solved, and the different group of people that we need to address. And, you engage them, right?” - Vijay (8:52)
- “When you’re designing in low fidelity, it allows you to design with users because you don’t spend all this time building the wrong thing upfront, at which point it’s really expensive in time and money to go and change it.” - Brian (17:11)
- "People are the ones who make things happen, right? You have all the technology, everything else looks good, you have the data, but the people are the ones who are going to make things happen.” - Vijay (38:47)
- “You want to make sure that you [have] a strong team and motivated team to deliver. And the human spirit is something, you cannot believe how stretchable it is. If the people are motivated, [and even if] you have less resources and less technology, they will still achieve [your goals].” - Vijay (42:41)
- “You’re trying to minimize any type of imposition on [the user], and make it obvious why your data product is better—without disruption. That’s really the key to the adoption piece: showing how it is going to be better for them in a way they can feel and perceive. Because if they don’t feel it, then it’s just another hoop to jump through, right?” - Brian (43:56)
Resources and Links:
LinkedIn: https://www.linkedin.com/in/vijyadav/
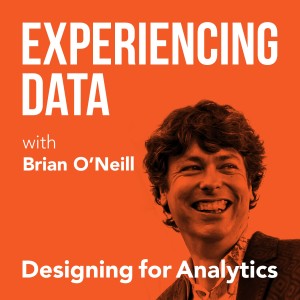
Tuesday Jun 14, 2022
093 - Why Agile Alone Won’t Increase Adoption of Your Enterprise Data Products
Tuesday Jun 14, 2022
Tuesday Jun 14, 2022
Episode Description
In one of my past memos to my list subscribers, I addressed some questions about agile and data products. Today, I expound on each of these and share some observations from my consulting work. In some enterprise orgs, mostly outside of the software industry, agile is still new and perceived as a panacea. In reality, it can just become a factory for shipping features and outputs faster–with positive outcomes and business value being mostly absent. To increase the adoption of enterprise data products that have humans in the loop, it’s great to have agility in mind, but poor technology shipped faster isn’t going to serve your customers any better than what you’re doing now.
Here are the 10 reflections I’ll dive into on this episode:
-
You can't project manage your way out of a [data] product problem.
-
The more you try to deploy agile at scale, take the trainings, and hire special "agilists", the more you're going to tend to measure success by how well you followed the Agile process.
-
Agile is great for software engineering, but nobody really wants "software engineering" given to them. They do care about the perceived reality of your data product.
-
Run from anyone that tells you that you shouldn't ever do any design, user research, or UX work "up front" because "that is waterfall."
-
Everybody else is also doing modified scrum (or modified _______).
-
Marty Cagan talks about this a lot, but in short: while the PM (product managers) may own the backlog and priorities, what’s more important is that these PMs “own the problem” space as opposed to owning features or being solution-centered.
-
Before Agile can thrive, you will need strong senior leadership buy-in if you're going to do outcome-driven data product work.
-
There's a huge promise in the word "agile." You've been warned.
-
If you don't have a plan for how you'll do discovery work, defining clear problem sets and success metrics, and understanding customers feelings, pains, needs, and wants, and the like, Agile won't deliver much improvement for data products (probably).
-
Getting comfortable with shipping half-right, half-quality, half-done is hard.
Quotes from Today’s Episode
- “You can get lost in following the process and thinking that as long as we do that, we’re going to end up with a great data product at the end.” - Brian (3:16)
- “The other way to define clear success criteria for data products and hold yourself accountable to those on the user and business side is to really understand what does a positive outcome look like? How would we measure it?” - Brian (5:26)
- “The most important thing is to know that the user experience is the perceived reality of the technology that you built. Their experience is the only reality that matters.” - Brian (9:22)
- “Do the right amount of planning work upfront, have a strategy in place, make sure the team understands it collectively, and then you can do the engineering using agile.” - Brian (18:15)
- “If you don’t have a plan for how you’ll do discovery work, defining clear problem sets and success metrics, and understanding customers’ feelings, pains, needs, wants, and all of that, then agile will not deliver increased adoption of your data products. - Brian (36:07)
Links:
- designingforanalytics.com: https://designingforanalytics.com
- designingforanalytics.com/list: https://designingforanalytics.com/list
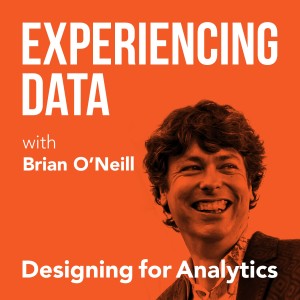
Tuesday May 31, 2022
Tuesday May 31, 2022
Today I’m talking about how to measure data product value from a user experience and business lens, and where leaders sometimes get it wrong. Today’s first question was asked at my recent talk at the Data Summit conference where an attendee asked how UX design fits into agile data product development. Additionally, I recently had a subscriber to my Insights mailing list ask about how to measure adoption, utilization, and satisfaction of data products. So, we’ll jump into that juicy topic as well.
Answering these inquiries also got me on a related tangent about the UX challenges associated with abstracting your platform to support multiple, but often theoretical, user needs—and the importance of collaboration to ensure your whole team is operating from the same set of assumptions or definitions about success. I conclude the episode with the concept of “game framing” as a way to conceptualize these ideas at a high level.
Key topics and cues in this episode include:
- An overview of the questions I received (:45)
- Measuring change once you’ve established a benchmark (7:45)
- The challenges of working in abstractions (abstracting your platform to facilitate theoretical future user needs) (10:48)
- The value of having shared definitions and understanding the needs of different stakeholders/users/customers (14:36)
- The importance of starting from the “last mile” (19:59)
- The difference between success metrics and progress metrics (24:31)
- How measuring feelings can be critical to measuring success (29:27)
- “Game framing” as a way to understand tracking progress and success (31:22)
Quotes from Today’s Episode
- “Once you’ve got your benchmark in place for a data product, it’s going to be much easier to measure what the change is because you’ll know where you’re starting from.” - Brian (7:45)
- “When you’re deploying technology that’s supposed to improve people’s lives so that you can get some promise of business value downstream, this is not a generic exercise. You have to go out and do the work to understand the status quo and what the pain is right now from the user's perspective.” - Brian (8:46)
- “That user perspective—perception even—is all that matters if you want to get to business value. The user experience is the perceived quality, usability, and utility of the data product.” - Brian (13:07)
- “A data product leader’s job should be to own the problem and not just the delivery of data product features, applications or technology outputs. ” - Brian (26:13)
- “What are we keeping score of? Different stakeholders are playing different games so it’s really important for the data product team not to impose their scoring system (definition of success) onto the customers, or the users, or the stakeholders.” - Brian (32:05)
- “We always want to abstract once we have a really good understanding of what people do, as it’s easier to create more user-centered abstractions that will actually answer real data questions later on. ” - Brian (33:34)
Links
- https://designingforanalytics.com/community
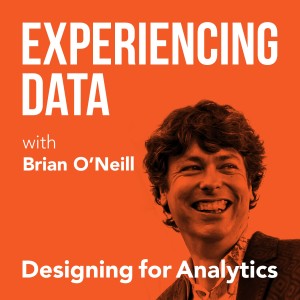
Tuesday May 17, 2022
Tuesday May 17, 2022
Today I talked with João Critis from Oi. Oi is a Brazilian telecommunications company that is a pioneer in convergent broadband services, pay TV, and local and long-distance voice transmission. They operate the largest fiber optics network in Brazil which reaches remote areas to promote digital inclusion of the population. João manages a design team at Oi that is responsible for the front end of data products including dashboards, reports, and all things data visualization.
We begin by discussing João’s role leading a team of data designers. João then explains what data products actually are, and who makes up his team’s users and customers. João goes on to discuss user adoption challenges at Oi and the methods they use to uncover what users need in the last mile. He then explains the specific challenges his team has faced, particularly with middle management, and how his team builds credibility with senior leadership. In conclusion, João reflects on the value of empathy in the design process.
In this episode, João shares:
- A data product (4:48)
- The research process used by his data teams to build journey maps for clients (7:31)
- User adoption challenges for Oi (15:27)
- His answer to the question “how do you decide which mouths to feed?” (16:56)
- The unique challenges of middle management in delivering useful data products (20:33)
- The importance of empathy in innovation (25:23)
- What data scientists need to learn about design and vice versa (27:55)
Quotes from Today’s Episode
- “We put the final user in the center of our process. We [conduct] workshops involving co-creation and prototyping, and we test how people work with data.” - João (8:22)
- "My first responsibility here is value generation. So, if you have to take two or three steps back, another brainstorm, rethink, and rebuild something that works…. [well], this is very common for us.” - João (19:28)
- “If you don’t make an impact on the individuals, you’re not going to make an impact on the business. Because as you said, if they don’t use any of the outputs we make, then they really aren’t solutions and no value is created. - Brian (25:07)
- “It’s really important to do what we call primary research where you’re directly interfacing as much as possible with the horse’s mouth, no third parties, no second parties. You’ve really got to develop that empathy.” - Brian (25:23)
- “When we are designing some system or screen or other digital artifact, [we have to understand] this is not only digital, but a product. We have to understand people, how people interact with systems, with computers, and how people interact with visual presentations.” - João (28:16)
Links
- Oi: https://www.oi.com.br/
- LinkedIn: https://www.linkedin.com/in/critis/
- Instagram: https://www.instagram.com/critis/
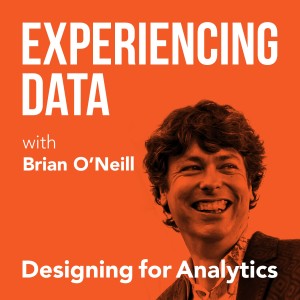
Tuesday May 03, 2022
Tuesday May 03, 2022
Michelle Carney began her career in the worlds of neuroscience and machine learning where she worked on the original Python Notebooks. As she fine-tuned ML models and started to notice discrepancies in the human experience of using these models, her interest turned towards UX. Michelle discusses how her work today as a UX researcher at Google impacts her work with teams leveraging ML in their applications. She explains how her interest in the crossover of ML and UX led her to start MLUX, a collection of meet-up events where professionals from both data science and design can connect and share methods and ideas. MLUX now hosts meet-ups in several locations as well as virtually.
Our conversation begins with Michelle’s explanation of how she teaches data scientists to integrate UX into the development of their products. As a teacher, Michelle utilizes the IDEO Design Kit with her students at the Stanford School of Design (d.school). In her teaching she shares some of the unlearning that data scientists need to do when trying to approach their work with a UX perspective in her course, Designing Machine Learning.
Finally, we also discussed what UX designers need to know about designing for ML/AI. Michelle also talks about how model interpretability is a facet of UX design and why model accuracy isn’t always the most important element of a ML application. Michelle ends the conversation with an emphasis on the need for more interdisciplinary voices in the fields of ML and AI.
Skip to a topic here:
- Michelle talks about what drove her career shift from machine learning and neuroscience to user experience (1:15)
- Michelle explains what MLUX is (4:40)
- How to get ML teams on board with the importance of user experience (6:54)
- Michelle discusses the “unlearning” data scientists might have to do as they reconsider ML from a UX perspective (9:15)
- Brian and Michelle talk about the importance of considering the UX from the beginning of model development (10:45)
- Michelle expounds on different ways to measure the effectiveness of user experience (15:10)
- Brian and Michelle talk about what is driving the increase in the need for designers on ML teams (19:59)
- Michelle explains the role of design around model interpretability and explainability (24:44)
Quotes from Today’s Episode
- “The first step to business value is the hurdle of adoption. A user has to be willing to try—and care—before you ever will get to business value.” - Brian O’Neill (13:01)
- “There’s so much talk about business value and there’s very little talk about adoption. I think providing value to the end-user is the gateway to getting any business value. If you’re building anything that has a human in the loop that’s not fully automated, you can’t get to business value if you don’t get through the first gate of adoption.” - Brian O’Neill (13:17)
- “I think that designers who are able to design for ambiguity are going to be the ones that tackle a lot of this AI and ML stuff.” - Michelle Carney (19:43)
- “That’s something that we have to think about with our ML models. We’re coming into this user’s life where there’s a lot of other things going on and our model is not their top priority, so we should design it so that it fits into their ecosystem.” - Michelle Carney (3:27)
- “If we aren’t thinking about privacy and ethics and explainability and usability from the beginning, then it’s not going to be embedded into our products. If we just treat usability of our ML models as a checkbox, then it just plays the role of a compliance function.” - Michelle Carney (11:52)
- “I don’t think you need to know ML or machine learning in order to design for ML and machine learning. You don’t need to understand how to build a model, you need to understand what the model does. You need to understand what the inputs and the outputs are.” - Michelle Carney (18:45)
Links
- Twitter @mluxmeetup: https://twitter.com/mluxmeetup
- MLUX LinkedIn: https://www.linkedin.com/company/mlux/
- MLUX YouTube channel: https://bit.ly/mluxyoutube
- Twitter @michelleRcarney: https://twitter.com/michelleRcarney
- IDEO Design Kit - https://tinyurl.com/2p984znh
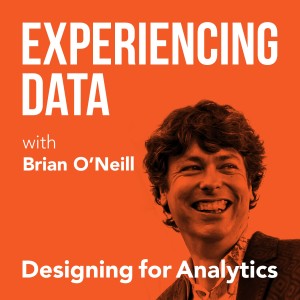
Tuesday Apr 19, 2022
089 - Reader Questions Answered about Dashboard UX Design
Tuesday Apr 19, 2022
Tuesday Apr 19, 2022
Dashboards are at the forefront of today’s episode, and so I will be responding to some reader questions who wrote in to one of my weekly mailing list missives about this topic. I’ve not talked much about dashboards despite their frequent appearance in data product UIs, and in this episode, I’ll explain why. Here are some of the key points and the original questions asked in this episode:
- My introduction to dashboards (00:00)
- Some overall thoughts on dashboards (02:50)
- What the risk is to the user if the insights are wrong or misinterpreted (4:56)
- Your data outputs create an experience, whether intentional or not (07:13)
- John asks:
How do we figure out exactly what the jobs are that the dashboard user is trying to do? Are they building next year's budget or looking for broken widgets? What does this user value today? Is a low resource utilization percentage something to be celebrated or avoided for this dashboard user today? (13:05) - Value is not intrinsically in the dashboard (18:47)
- Mareike asks:
How do we provide Information in a way that people are able to act upon the presented Information? How do we translate the presented Information into action? What can we learn about user expectation management when designing dashboard/analytics solutions? (22:00) - The change towards predictive and prescriptive analytics (24:30)
- The upfront work that needs to get done before the technology is in front of the user (30:20)
- James asks:
How can we get people to focus less on the assumption-laden and often restrictive term "dashboard", and instead worry about designing solutions focused on outcomes for particular personas and workflows that happen to have some or all of the typical ingredients associated with the catch-all term "dashboards?” (33:30) - Stop measuring the creation of outputs and focus on the user workflows and the jobs to be done (37:00)
- The data product manager shouldn’t just be focused on deliverables (42:28)
Quotes from Today’s Episode
- “The term dashboards is almost meaningless today, it seems to mean almost any home default screen in a data product. It also can just mean a report. For others, it means an entire monitoring tool, for some, it means the summary of a bunch of data that lives in some other reports. The terms are all over the place.”- Brian (@rhythmspice) (01:36)
- “The big idea here that I really want leaders to be thinking about here is you need to get your teams focused on workflows—sometimes called jobs to be done—and the downstream decisions that users want to make with machine-learning or analytical insights. ” - Brian (@rhythmspice) (06:12)
- “This idea of human-centered design and user experience is really about trying to fit the technology into their world, from their perspective as opposed to building something in isolation where we then try to get them to adopt our thing. This may be out of phase with the way people like to do their work and may lead to a much higher barrier to adoption.” - Brian (@rhythmspice) (14:30)
- “Leaders who want their data science and analytics efforts to show value really need to understand that value is not intrinsically in the dashboard or the model or the engineering or the analysis.” - Brian (@rhythmspice) (18:45)
- “There's a whole bunch of plumbing that needs to be done, and it’s really difficult. The tool that we end up generating in those situations tends to be a tool that’s modeled around the data and not modeled around [the customers] mental model of this space, the customer purchase space, the marketing spend space, the sales conversion, or propensity-to-buy space.” - Brian (@rhythmspice) (27:48)
- “Data product managers should be these problem owners, if there has to be a single entity for this. When we’re talking about different initiatives in the enterprise or for a commercial software company, it’s really sits at this product management function.” - Brian (@rhythmspice) (34:42)
- “It’s really important that [data product managers] are not just focused on deliverables; they need to really be the ones that summarize the problem space for the entire team, and help define a strategy with the entire team that clarifies the direction the team is going in. They are not a project manager; they are someone responsible for delivering value.” - Brian (@rhythmspice) (42:23)
Links Referenced:
- Mailing List: https://designingforanalytics.com/list
- CED UX Framework for Advanced Analytics:
- My LinkedIn Live about Measuring the Usability of Data Products: https://www.linkedin.com/video/event/urn:li:ugcPost:6911800738209800192/
- Work With Me / My Services: https://designingforanalytics.com/services