
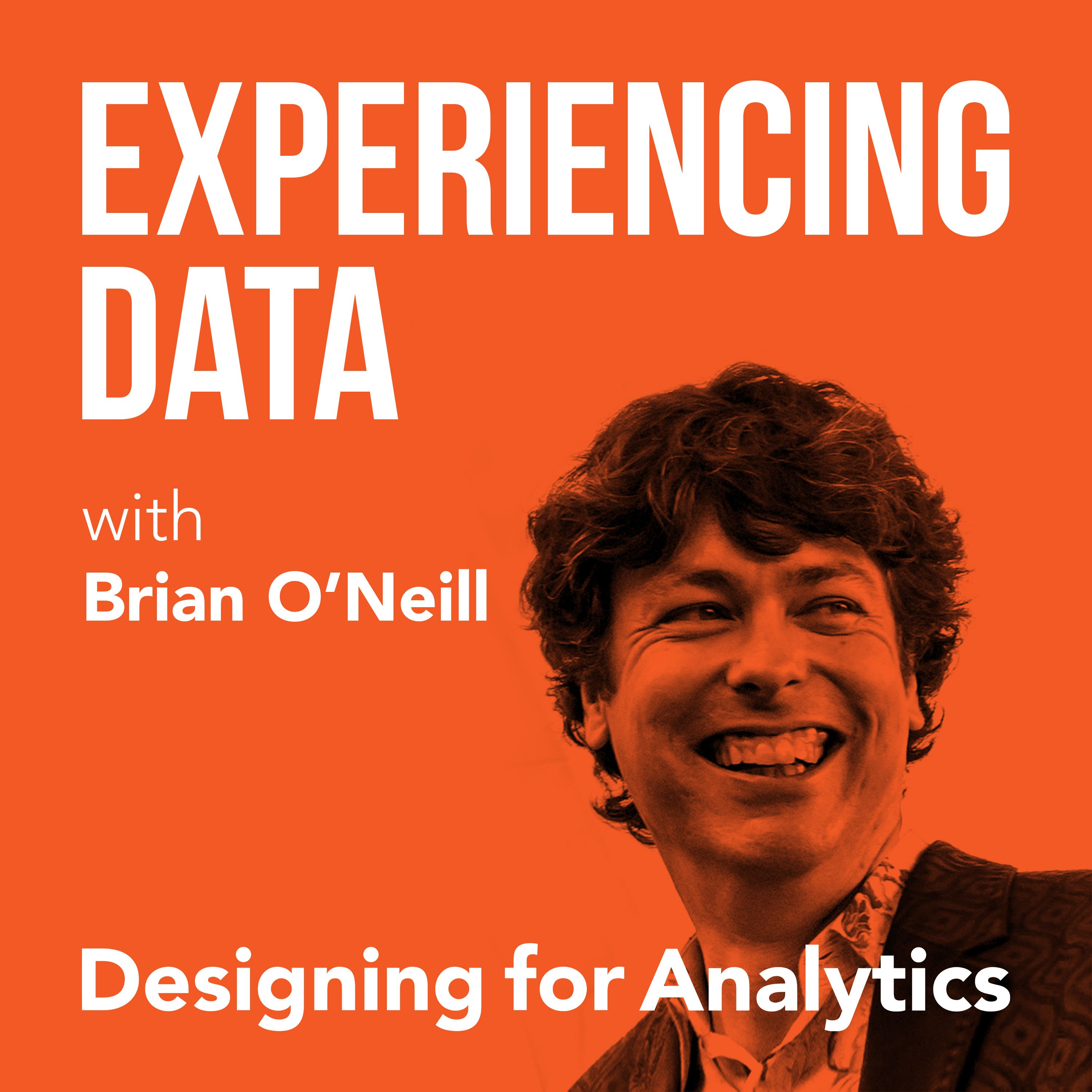
132.3K
Downloads
165
Episodes
Is the value of your enterprise analytics SAAS or AI product not obvious through it’s UI/UX? Got the data and ML models right...but user adoption of your dashboards and UI isn’t what you hoped it would be? While it is easier than ever to create AI and analytics solutions from a technology perspective, do you find as a founder or product leader that getting users to use and buyers to buy seems harder than it should be? If you lead an internal enterprise data team, have you heard that a ”data product” approach can help—but you’re concerned it’s all hype? My name is Brian T. O’Neill, and on Experiencing Data—one of the top 2% of podcasts in the world—I share the stories of leaders who are leveraging product and UX design to make SAAS analytics, AI applications, and internal data products indispensable to their customers. After all, you can’t create business value with data if the humans in the loop can’t or won’t use your solutions. Every 2 weeks, I release interviews with experts and impressive people I’ve met who are doing interesting work at the intersection of enterprise software product management, UX design, AI and analytics—work that you need to hear about and from whom I hope you can borrow strategies. I also occasionally record solo episodes on applying UI/UX design strategies to data products—so you and your team can unlock financial value by making your users’ and customers’ lives better. Hashtag: #ExperiencingData. JOIN MY INSIGHTS LIST FOR 1-PAGE EPISODE SUMMARIES, TRANSCRIPTS, AND FREE UX STRATEGY TIPS https://designingforanalytics.com/ed ABOUT THE HOST, BRIAN T. O’NEILL: https://designingforanalytics.com/bio/
Episodes
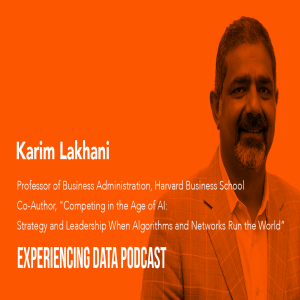
Tuesday Jul 28, 2020
Tuesday Jul 28, 2020
If there’s one thing that strikes fear into the heart of every business executive, it’s having your company become the next Blockbuster or Neiman Marcus — that is, ignoring change, and getting wiped out by digital competitors. In this episode, I dived into the changing business landscape with Karim Lakhani who is a Professor at Harvard Business School and co-author of the new book Competing in the Age of AI: When Algorithms and Networks Run the World, which he wrote with his friend and colleague at HBS, Marco Iansiti.
We discuss how AI, machine learning, and digital operating models are changing business architecture, and disrupting traditional business models. I also pressed Karim to go a bit deeper on how, and whether he thinks product mindset and design factor in to the success of AI in today’s businesses. We also go off on a fun tangent about the music industry, which just might have to be a future episode!. In any case, I highly recommend the book. It’s particularly practical for those of you working in organizations that are not digital natives and want to hear how the featured companies in the book are setting themselves apart by leveraging data and AI in customer-facing products and in internal applications/operations. Our conversation covers:
- Karim’s new book, Competing in the Age of AI: When Algorithms and Networks Run the World, co-authored with Marco Iansiti.
- How digital operating models are colliding with traditional product-oriented businesses, and the impact this is having on today’s organizations.
- The critical role of data product management that is frequently missing when companies try to leverage AI
- Karim’s thoughts on ethics in AI and machine learning systems, and how they need to be baked into business and engineering.
- The similarity Karim sees between COVID-19 and AI
- The role of design, particularly in human-in-the-loop systems and how companies need to consider the human experience in applications of AI that augment decision making vs. automate it.
- How Karim sees the ability to adapt in business as being critical to survival in the age of AI
Resources and Links
- Book Link: https://www.amazon.com/Competing-Age-AI-Leadership-Algorithms/dp/1633697622/
- Twitter: https://twitter.com/klakhani
- LinkedIn: https://www.linkedin.com/in/professorkl/
- Harvard Business Analytics Program: https://analytics.hbs.edu/
Quotes from Today’s Episode
“Our thesis in the book is that a new type of an organization is emerging, which has eliminated bottlenecks in old processes.” - Karim
“Digital operating models have exponential scaling properties, in terms of the value they generate, versus traditional companies that have value curves that basically flatten out, and have fixed capacity. Over time, these digital operating models collide with these traditional product models, win over customers, and gather huge amounts of market share….” - Karim
“This whole question about human-in-the-loop is important, and it's not going to go away, but we need to start thinking about, well, how good are the humans, anyway? - Karim
“Somebody once said, “Ethics defines the boundaries of what you care about.” And I think that's a really important question…” - Brian
“Non-digital natives worry about these tech companies coming around and eating them up, and I can’t help but wonder ‘why aren't you also copying the way they design and build software?’” - Brian
“...These established companies have a tough time with the change process.” - Karim
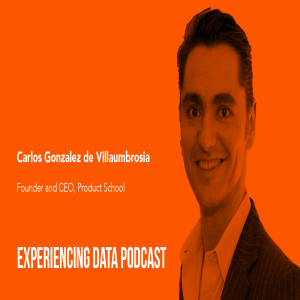
Tuesday Jul 14, 2020
Tuesday Jul 14, 2020
I am a firm believer that one of the reasons that data science and analytics has a high failure rate is a lack of product management and design. To me, product is about a mindset just as much as a job title, and I am repeatedly hearing how more and more voices in the data community are agreeing with me on this (Gartner CDO v4, International Inst. for Analytics, several O’Reilly authors, Karim Lakhani’s new book on AI, and others). This is even more true as more companies begin to leverage AI. So many of these companies fear what startups and software companies are doing, yet they do not copy the way tech companies build software applications and enable specific user experiences that unlock the desired business value.
Integral to building software is the product management function—and when these applications and tools have humans in the loop, the product/UX design function is equally as important to ensure adoption, usability, engagement, and alignment with the business objectives.
In modern tech companies, the overlap between product design and product management can be significant, and frequently, product leaders in tech companies come up through both design and engineering ranks and indeed my own work heavily overlaps with product. What this tells me is that product is a mindset, and it’s a role many can learn if they believe it’s critical.
So why aren’t more data science and analytics leaders forming strong product design and analytics functions? I don’t know, so I decided to bring Carlos onto the show to talk about his company, Product School, which offers product management training and features instructors from many of the big tech companies on how to do it. In this episode, Carlos provides a comprehensive overview of why he launched Product School, what makes an effective product manager, and the importance of having structured vision and alignment when developing products.
This conversation explores:
- Why Carlos launched the Product School for professionals who want to learn on the side without quitting their job and putting their life on hold.
- The type of mentality product managers need to have and whether specialization matters within product management.
- Whether being a product manager in machine learning and AI is different than working with a traditional software product.
- How product management is not project management
- Advice for approaching executive decision makers about product management education
- How to avoid the trap of focusing too heavily on process
- How product management often leads to executive leadership roles
- The “power trio” of engineering, product management, and design, and the value of aligning all three groups.
- Understanding the difference between applied and academic experience
- How the relationship between design and PM has changed over the last five years
- What the gap looks like between a skilled PM and an exceptional one.
Resources and Links
The State of Product Analytics (Also referred to as The Future of Product Analytics in the audio)
Mixpanel, company that they partnered with to create the above report
Episode 17 of Experiencing Data
Quotes from Today’s Episode
“You can become a product manager by building products. You don't need to be a software engineer. You don’t need to have an MBA. You don't need to be an incredible, inspiring visionary. This is stuff that you can learn, and the best way to learn it is by doing it.” - Carlos
“A product manager is a generalist. And in order to become a generalist, usually you have to have some sort of [specialty] before. So, we define product management as the intersection in between business, engineering, and design. And you can become a good product manager from either of those options.” - Carlos
“If you have [a power trio of technology, product, and design] and the energy is right, and the relationships are really strong, boy, you can get a lot of stuff done, and you can iterate quickly, and really produce some great stuff.” - Brian
“I think part of the product management mindset... is to realize part of your job now is to be a problem finder, it’s to help set the strategy, it's to help ensure that a model is not the solution.” - Brian
“I think about a bicycle wheel with the hub in the center and the spokes coming out. Product management is that hub, and it reports up into the business, but you have all these different spokes, QA, and software engineering, maybe data science and analytics, product design, and user experience design. These are all kind of spokes.” - Brian
“These are people who are constantly learning, but not just about their products. They’re constantly learning in general. Reading books, practicing sports, doing whatever it is, but always looking at what's new and wanting to play around with it, just to be dangerous enough. So, I think those three areas: obsession with a customer based on data; obsession with empathy; and then obsession with learning, or just being curious are really critical.” - Carlos
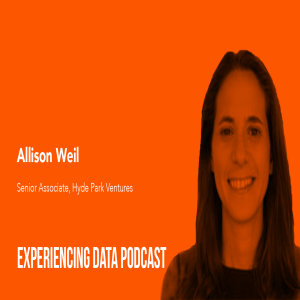
Tuesday Jun 30, 2020
Tuesday Jun 30, 2020
“What happened in Minneapolis and Louisville and Chicago and countlessother cities across the United States is unconscionable (and to be clear, racist). But what makes me the maddest is how easy this problem is to solve, just by the police deciding it’s a thing they want to solve.” - Allison Weil on Medium Before Allison Weil became an investor and Senior Associate at Hyde Park Ventures, she was a co-founder at Flag Analytics, an early intervention system for police departments designed to help identify officers at risk of committing harm. Unfortunately, Flag Analytics—as a business—was set up for failure from the start, regardless of its predictive capability. As Allison explains so candidly and openly in her recent Medium article (thanks Allison!), the company had “poor product-market fit, a poor problem-market fit, and a poor founder-market fit.” The technology was not the problem, and as a result, it did not help them succeed as a business or in producing the desired behavior change because the customers were not ready to act on the insights. Yet, the key takeaways from her team’s research during the design and validation of their product — and the uncomfortable truths they uncovered — are extremely valuable, especially now as we attempt to understand why racial injustice and police brutality continue to persist in law enforcement agencies. As it turns out, simply having the data to support a decision doesn’t mean the decision will be made using the data. This is what Allison found out while in her interactions with several police chiefs and departments, and it’s also what we discussed in this episode. I asked Allison to go deeper into her Medium article, and she agreed. Together, we covered:
- How Allison and a group of researchers tried to streamline the identification of urban police officers at risk of misconduct or harm using machine learning.
- Allison’s experience of trying to build a company and program to solve a critical societal issue, and dealing with police departments that weren’t ready to take action on the analytical insights her product revealed
- How she went about creating a “single pane of glass,” where officers could monitor known problem officers and also discover officers who may be in danger of committing harm.
- The barriers that prevented the project from being a success, from financial ones to a general unwillingness among certain departments to take remedial action against officers despite historical or predicted data
- The key factors and predictors Allison’s team found in the data set of thousands of officers that correlated highly with poor officer behavior in the future—and how it seemed to fall on deaf ears
- How Allison and her team approached the sensitive issue of race in the data, and a [perhaps unexpected] finding they discovered about how prevalent racism seemed to be in departments in general.
- Allison’s experience of conducting “ride-alongs” (qualitative 1x1 research) where she went on patrol with officers to observe their work and how the experience influenced how her team designed the product and influenced her perspective while analyzing the police officer data set.
Resources and Links:
Quotes from Today’s Episode
“The folks at the police departments that we were working with said they were well-intentioned, and said that they wanted to talk through, and fix the problem, but when it came to their actions, it didn't seem like [they were] really willing to make the choices that they needed to make based off of what the data said, and based off of what they knew already.” - Allison “I don't come from a policing background, and neither did any of my co-founders. And that made it really difficult to relate to different officers, and relate to departments. And so the combination of all of those things really didn't set me up for a whole lot of business success in that way.”- Allison “You can take a whole lot of data and do a bunch of analysis, but what I saw was the data didn't show anything that the police department didn't know already. It amplified some of what they knew, but [the problem here] wasn't about the data.” - Allison “It was really frustrating for me, as a founder, sure, because I was putting all this energy into trying to build a software and trying to build a company, but also just frustrating for me as a person and a citizen… you fundamentally want to solve a problem, or help a community solve a problem, and realize that the people at the center of it just aren't ready for it to be solved.” - Allison “...We did have race data, but race was not the primary predictor or reason for [brutality]. It may have been a factor, but it was not that there were racist cops wandering around, using force only against people of particular races. What we found was….” - Allison “The way complaints are filed department to department is really, really different. And so that results in complaints looking really, really different from department to department and counts looking different. But how many are actually reviewed and sustained? And that looks really, really different department to department.” - Allison “...Part of [diversity] is asking the questions you don't know to ask. And that's part of what you get out of having a diverse team— they're going to surface questions that no one else is asking about. And then you can have the discussion about what to do about them.” - Brian
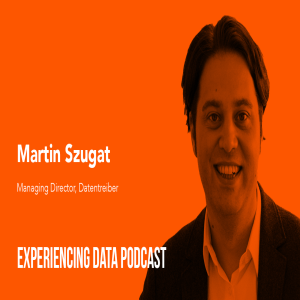
Tuesday Jun 16, 2020
Tuesday Jun 16, 2020
The job of many internally-facing data scientists in business settings is to discover,explore, interpret, and share data, turning it into actionable insight that can benefit the company and improve outcomes. Yet, data science teams often struggle with the very basic question of how the company’s data assets can best serve the organization. Problem statements are often vague, leading to data outputs that don’t turn into value or actionable decision support in the last mile.
This is where Martin Szugat and his team at Datentreiber step in, helping clients to develop and implement successful data strategy through hands-on workshops and training. Martin is based in Germany and specializes in helping teams learn to identify specific challenges data can solve, and think through the problem solving process with a human focus. This in turn helps teams to select the right technology and be objective about whether they need advanced tools such as ML/AI, or something more simple to produce value.
In our chat, we covered:
- How Datentreiber helps clients understand and derive value from their data — identifying assets, and determining relevant use cases.
- An example of how one client changed not only its core business model, but also its culture by working with Datentreiber, transitioning from a data-driven perspective to a user-driven perspective.
- Martin’s strategy of starting with small analytics projects, and slowly gaining buy-in from end users, with a special example around social media analytics that led to greater acceptance and understanding among team members.
- The canvas tools Martin likes to use to visualize abstract concepts related to data strategy, data products, and data analysis.
- Why it helps to mix team members from different departments like marketing, sales, and IT and how Martin goes about doing that
- How cultural differences can impact design thinking, collaboration, and visualization processes.
Resources and Links:
- Company site (German) (English machine translation)
- Datentreiber Open-Source Design Tools
- Data Strategy Design (German) (English machine translation)
- Martin’s LinkedIn
Quotes from Today’s Episode
“Often, [clients] already have this feeling that they're on the wrong path, but they can't articulate it. They can't name the reason why they think they are on the wrong path. They learn that they built this shiny dashboard or whatever, but the people—their users, their colleagues—don't use this dashboard, and then they learn something is wrong.” - Martin
“I usually like to call this technically right and effectively wrong solutions. So, you did all the pipelining and engineering and all that stuff is just fine, but it didn't produce a meaningful outcome for the person that it was supposed to satisfy with some kind of decision support.” - Brian
“A simple solution is becoming a trainee in other departments. So, ask, for example, the marketing department to spend a day, or a week and help them do their work. And just look over the shoulder, what they are doing, and really try to understand what they are doing, and why they are doing it, and how they are doing it. And then, come up with solution proposals.” - Martin
...I tend to think of design as a team sport, and it's a lot about facilitating groups of these different cross-departmental groups of arriving at a solution for a particular audience; a specific audience that needs a specific problem solved.” - Brian
“[One client said] we are very good at implementing the right solutions for the wrong problems. And I think this is what often happens in data science, or business intelligence, or whatever, also in IT departments: that they are too quick in starting thinking about the solution before they understand the problem.” - Martin
“If people don't understand what you're doing or what your analytic solution is doing, they won't use it and there will be no acceptance.” - Martin
“One thing we practice a lot, [...] is in visualizing those abstract things like data strategy, data product, and analytics. So, we work a lot with canvas tools because we learned that if you show people—and it doesn't matter if it's just on a sticky note on a canvas—then people start realizing it, they start thinking about it, and they start asking the right questions and discussing the right things. ” - Martin
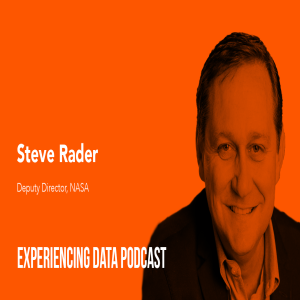
Tuesday Jun 02, 2020
Tuesday Jun 02, 2020
Innovation doesn’t just happen out of thin air. It requires a conscious effort, and team-wide collaboration. At the same time, innovation will be critical for NASA if the organization hopes to remain competitive and successful in the coming years. Enter Steve Rader. Steve has spent the last 31 years at NASA, working in a variety of roles including flight control under the legendary Gene Kranz, software development, and communications architecture. A few years ago, Steve was named Deputy Director for the Center of Excellence for Collaborative Innovation. As Deputy Director, Steve is spearheading the use of open innovation, as well as diversity thinking. In doing so, Steve is helping the organization find more effective ways of approaching and solving problems. In this fascinating discussion, Steve and Brian discuss design, divergent thinking, and open innovation plus:
- Why Steve decided to shift away from hands-on engineering and management to the emerging field of open innovation, and why NASA needs this as well as diversity in order to remain competitive.
- The challenge of convincing leadership that diversity of thought matters, and why the idea of innovation often receives pushback.
- How NASA is starting to make room for diversity of thought, and leveraging open innovation to solve challenges and bring new ideas forward.
- Examples of how experts from unrelated fields help discover breakthroughs to complex and greasy problems, such as potato chips!
- How the rate of technological change is different today, why innovation is more important than ever, and how crowdsourcing can help streamline problem solving.
- Steve’s thoughts on the type of leader that’s needed to drive diversity at scale, and why that person should be a generalistPrioritizing outcomes over outputs, defining problems, and determining what success looks like early on in a project.
- The metrics a team can use to measure whether one is “doing innovation.”
Resources and Links
Designingforanalytics.com/theseminar Steve Rader’s LinkedIn: https://www.linkedin.com/in/steve-rader-92b7754/ NASA Solve: nasa.gov/solve Steve Rader’s Twitter: https://twitter.com/SteveRader NASA Solve Twitter: https://twitter.com/NASAsolve
Quotes from Today’s Episode
“The big benefit you get from open innovation is that it brings diversity into the equation […]and forms this collaborative effort that is actually really, really effective.” – Steve “When you start talking about innovation, the first thing that almost everyone does is what I call the innovation eye-roll. Because management always likes to bring up that we’re innovative or we need innovation. And it just sounds so hand-wavy, like you say. And in a lot of organizations, it gets lots of lip service, but almost no funding, almost no support. In most organizations, including NASA, you’re trying to get something out the door that pays the bills. Ours isn’t to pay the bills, but it’s to make Congress happy. And, when you’re doing that, that is a really hard, rough space for innovation.” – Steve “We’ve run challenges where we’re trying to improve a solar flare algorithm, and we’ve got, like, a two-hour prediction that we’re trying to get to four hours, and the winner of that in the challenge ends up to be a cell phone engineer who had an undergraduate degree from, like, 30 years prior that he never used in heliophysics, but he was able to take that extracting signal from noise math that they use in cell phones, and apply it to heliophysics to get an eight-hour prediction capability.” – Steve “If you look at how long companies stay around, the average in 1958 was 60 years, it is now less than 18. The rate of technology change and the old model isn’t working anymore. You can’t actually get all the skills you need, all the diversity. That’s why innovation is so important now, is because it’s happening at such a rate, that companies—that didn’t used to have to innovate at this pace—are now having to innovate in ways they never thought.” – Steve “…Innovation is being driven by this big technology machine that’s happening out there, where people are putting automation to work. And there’s amazing new jobs being created by that, but it does take someone who can see what’s coming, and can see the value of augmenting their experts with diversity, with open innovation, with open techniques, with innovation techniques, period.” – Steve “…You have to be able to fail and not be afraid to fail in order to find the real stuff. But I tell people, if you’re not willing to listen to ideas that won’t work, and you reject them out of hand and shut people down, you’re probably missing out on the path to innovation because oftentimes, the most innovative ideas only come after everyone’s thrown in 5 to 10 ideas that actually won’t work.” – Steve
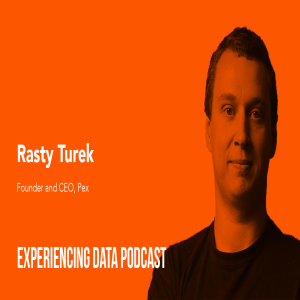
Tuesday May 19, 2020
Tuesday May 19, 2020
Every now and then, I like to insert a music-and-data episode into the show since hey, I’m a musician, and I’m the host 😉 Today is one of those days!
Rasty Turek is founder and CEO of Pex, a leading analytics and rights management platform used for discovering and tracking video and audio content using data science.
Pex’s AI crawls the internet for user-generated content (UGC), identifies copyrighted audio/ visual content, indexes the media, and then enables rights holders to understand where their art is being used so it can be monetized. Pex’s goal is to help its customers understand who is using their licensed content, and what they are using it for — along with key insights to support monetization initiatives and negotiations with UGC platform providers.
In this episode of Experiencing Data, we discuss:
- How the data science behind Pex works in terms of being able to fingerprint actual songs (the underlying IP of a composition) vs. masters (actual audio recordings of songs)
- The challenges PEX has in identifying complex, audio-rich user-generated content and cover recordings, and ensuring it is indexing as many usages as possible.
- The transitioning UGC market, and how Pex is trying to facilitate change. One item that Rasty discusses is Europe’s new Copyright Directive law, and how it’s impacting UGC from a licensing standpoint.
- How analytics are empowering publishers, giving them key insights and firepower to negotiate with UGC platforms over licensed content.
- Key product design and UX considerations that Pex has taken to make their analytics useful to customers
- What Rasty learned through his software iteration journey at Pex, including a memorable example about bias that influenced future iterations of the design/UI/UX
- How Pex predicts and priorities monetization opportunities for customers, and how they surface infringements.
- Why copyright education is the “last bastion of the internet” — and the role that Pex is playing in streamlining copyrighted material.
Brian also challenges Rasty directly, asking him how the Pex platform balances flexibility with complexity when dealing with extremely large data sets.
Resources and Links
Designingforanalytics.com/theseminar
Twitter: https://twitter.com/synopsi
Quotes from Today’s Episode
“I will say, 80 to 90 percent of the population eventually will be rights owners of some sort, since this is how copyright works. Everybody that produces something is immediately a rights owner, but I think most of us will eventually generate our livelihood through some form of IP, especially if you believe that the machines are going to take the manual labor from us.” - Rasty
“When people ask me how it is to run a big data company, I always tell them I wish we were not [a big data company], because I would much rather have “small data,” and have a very good business, rather than big data.” - Rasty
“There's a lot of these companies that [have operated] in this field for 20 to 30 years, we just took it a little bit further. We adjusted it towards the UGC world, and we focused on simplicity” - Rasty
“We don't follow users, we follow content. And so, at some point [during our design process] we were exploring if we could follow users [of our customers’ copyrighted content].... As we explored this more, we started noticing that [our customers] started making incorrect decisions because they were biased towards users [of their copyrighted content].” - Rasty
“If you think that your general customer is a coastal elite, but the reality is that they are Midwest farmers, you don't want to see that as the reality and you start being biased towards that. So, we immediately started removing that data and really focused on the content itself—because that content is not biased.” - Rasty
“[Re: PEX’s design process] We always started with the guiding principles. What is the task that you're trying to solve? So, for instance, if your task is to monetize your content, then obviously you want to monetize the most obvious content that will get the most views, right?.” - Rasty
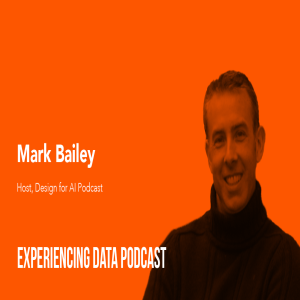
Tuesday May 05, 2020
Tuesday May 05, 2020
Mark Bailey is a leading UX researcher and designer, and host of the Design for AI podcast — a
program which, similar to Experiencing Data, explores the strategies and considerations around designing data-driven human-centered applications built with machine learning and AI.
In this episode of Experiencing Data — co-released with the podcast Design for AI — Brian and Mark share the host and guest role, and discuss 10 different UX concepts teams may need to consider when approaching ML-driven data products and AI applications. A great discussion on design and #MLUX ensued, covering:
- Recognizing the barrier of trust and adoption that exists with ML, particularly at non-digital native companies, and how to address it when designing solutions.
- Why designers need to dig beyond surface level knowledge of ML, and develop a comprehensive understanding of the space
- How companies attempt to “separate reality from the movies,” with AI and ML, deploying creative strategies to build trust with end users (with specific examples from Apple and Tesla)
- Designing for “undesirable results” (how to gracefully handle the UX when a model produces unexpected predictions)
- The ongoing dance of balancing UX with organizational goals and engineering milestones
- What designers and solution creators need to be planning for and anticipating with AI products and applications
- Accessibility considerations with AI products and applications – and how itcan be improved
- Mark’s approach to ethics and community as part of the design process.
- The importance of systems design thinking when collecting data and designing models
- The different model types and deployment considerations that affect a solution’s UX — and what solution designers need to know to stay ahead
- Collaborating, and visualizing — or storyboarding — with developers, to help understand data transformation and improve model design
- The role that designers can play in developing model transparency (i.e. interpretability and explainable AI)
- Thinking about pain points or problems that can be outfitted with decision support or intelligence to make an experience better
Resources and Links:
Experiencing Data – Episode 35
Designing for Analytics Seminar
Quotes from Today’s Episode
“There’s not always going to be a software application that is the output of a machine learning model or something like that. So, to me, designers need to be thinking about decision support as being the desired outcome, whatever that may be.” – Brian
“… There are [about] 30 to 40 different types of machine learning models that are the most popular ones right now. Knowing what each one of them is good for, as the designer, really helps to conform the machine learning to the problem instead of vice versa.” – Mark
“You can be technically right and effectively wrong. All the math part [may be] right, but it can be ineffective if the human adoption piece wasn’t really factored into the solution from the start.” – Brian
“I think it’s very interesting to see what some of the big companies have done, such as Apple. They won’t use the term AI, or machine learning in any of their products. You’ll see their chips, they call them neural engines instead have anything to do with AI. I mean, so building the trust, part of it is trying to separate out reality from movies.” – Mark
“Trust and adoption is really important because of the probabilistic nature of these solutions. They’re not always going to spit out the same thing all the time. We don’t manually design every single experience anymore. We don’t always know what’s going to happen, and so it’s a system that we need to design for.” – Brian
“[Thinking about] a small piece of intelligence that adds some type of value for the customer, that can also be part of the role of the designer.” – Brian
“For a lot of us that have worked in the software industry, our power trio has been product management, software engineering lead, and some type of design lead. And then, I always talk about these rings, like, that’s the close circle. And then, the next ring out, you might have some domain experts, and some front end developer, or prototyper, a researcher, but at its core, there were these three functions there. So, with AI, is it necessary, now, that we add a fourth function to that, especially if our product was very centered around this? That’s the role of the data scientist. And so, it’s no longer a trio anymore.” – Brian
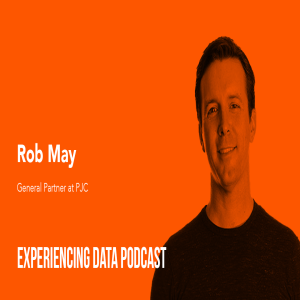
Tuesday Apr 21, 2020
Tuesday Apr 21, 2020
Rob May is a general partner at PJC, a leading venture capital firm. He was previously CEO of Talla, a platform for AI and automation, as well as co-founder and CEO of Backupify. Rob is an angel investor who has invested in numerous companies, and author of InsideAI which is said to be one of the most widely-read AI newsletters on the planet.
In this episode, Rob and I discuss AI from a VC perspective. We look into the current state of AI, service as a software, and what Rob looks for in his startup investments and portfolio companies. We also investigate why so many companies are struggling to push their AI projects forward to completion, and how this can be improved. Finally, we outline some important things that founders can do to make products based on machine intelligence (machine learning) attractive to investors.
In our chat, we covered:
- The emergence of service as a software, which can be understood as a logical extension of “software eating the world” and the 2 hard things to get right (Yes, you read it correctly and Rob will explain what this new SAAS acronym means!) !
- How automation can enable workers to complete tasks more efficiently and focus on bigger problems machines aren’t as good at solving
- Why AI will become ubiquitous in business—but not for 10-15 years
- Rob’s Predict, Automate, and Classify (PAC) framework for deploying AI for business value, and how it can help achieve maximum economic impact
- Economic and societal considerations that people should be thinking about when developing AI – and what we aren’t ready for yet as a society
- Dealing with biases and stereotypes in data, and the ethical issues they can create when training models
- How using synthetic data in certain situations can improve AI models and facilitate usage of the technology
- Concepts product managers of AI and ML solutions should be thinking about
- Training, UX and classification issues when designing experiences around AI
- The importance of model-market fit. In other words, whether a model satisfies a market demand, and whether it will actually make a difference after being deployed.
Resources and Links:
The PAC Framework for Deploying AI
Quotes from Today’s Episode
“[Service as a software] is a logical extension of software eating the world. Software eats industry after industry, and now it’s eating industries using machine learning that are primarily human labor focused.” — Rob
“It doesn’t have to be all digital. You could also think about it in terms of restaurant automation, and some of those things where if you keep the interface the same to the customer—the service you’re providing—you strip it out, and everything behind that, if it’s digital it’s an algorithm and if it’s physical, then you use a robot.” — Rob, on service as a software.
“[When designing for] AI you really want to find some way to convey to the user that the tool is getting smarter and learning.”— Rob
“There’s a gap right now between the business use cases of AI and the places it’s getting adopted in organizations,” — Rob
“The reason that AI’s so interesting is because what you effectively have now is software models that don’t just execute a task, but they can learn from that execution process and change how they execute.” — Rob
“If you are changing things and your business is changing, which is most businesses these days, then it’s going to help to have models around that can learn and grow and adapt. I think as we get better with different data types—not just text and images, but more and more types of data types—I think every business is going to deploy AI at some stage.” — Rob
“The general sense I get is that overall, putting these models and AI solutions is pretty difficult still.” — Brian
“They’re not looking at what’s the actual best use of AI for their business, [and thinking] ‘Where could you really apply to have the most economic impact?’ There aren’t a lot of people that have thought about it that way.” — Rob, on how AI is being misapplied in the enterprise.
“You have to focus on the outcome, not just the output.” — Brian
“We need more heuristics for how, as a product manager, you think of AI and building it into products.” — Rob
“When the internet came about, it impacted almost every business in some way, shape, or form.[…]he reason that AI’s so interesting is because what you effectively have now is software models that don’t just execute a task, but they can learn from that execution process and change how they execute.” — Rob
“Some biases and stereotypes are true, and so what happens if the AI uncovers one that we’re really uncomfortable with?” — Rob
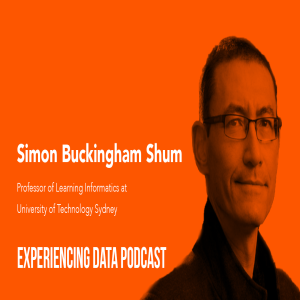
Tuesday Apr 07, 2020
Tuesday Apr 07, 2020
Simon Buckingham Shum is Professor of Learning Informatics at Australia’s University of Technology Sydney (UTS) and Director of the Connected Intelligence Centre (CIC)—an innovation center where students and staff can explore education data science applications. Simon holds a Ph.D from the University of York, and is known for bringing a human-centered approach to analytics and development. He also co-founded the Society for Learning Analytics Research (SoLAR), which is committed to advancing learning through ethical, educationally sound data science.
In this episode, Simon and I discuss the state of education technology (edtech), privacy, human-centered design in the context of using AI in higher ed, and the numerous technological advancements that are re-shaping the higher level education landscape.
Our conversation covered:
- How the hype cycle around big data and analytics is starting to pervade education
- The differences between using BI and analytics to streamline operations, improve retention rates, vs. the ways AI and data are used to increase learning and engagement
- Creating systems that teachers see as interesting and valuable, in order to drive user adoption and avoid friction.
- The more difficult-to-design-for, but more important skills and competencies researchers are working on to prepare students for a highly complex future workplace
- The data and privacy issues that must be factored into ethical solution designs
- Why “learning is not shopping,” meaning we the creators of the tech have to infer what goes on in the mind when studying humans, mostly by studying behavior.
- Why learning scientists and educational professionals play an important role in the edtech design process, in addition to technical workers
- How predictive modeling can be used to identify students who are struggling—and the ethical questions that such solutions raise.
Resources and Links
Designing for Analytics Podcast
Quotes from Today’s Episode
“We are seeing AI products coming out. Some of them are great, and are making a huge difference for learning STEM type subjects— science, tech, engineering, and medicine. But some of them are not getting the balance right.” — Simon
“The trust break-down will come, and has already come in certain situations, when students feel they’re being tracked…” — Simon, on students perceiving BI solutions as surveillance tools instead of beneficial
“Increasingly, it’s great to see so many people asking critical questions about the biases that you can get in training data, and in algorithms as well. We want to ask questions about whether people are trusting this technology. It’s all very well to talk about big data and AI, etc., but ultimately, no one’s going to use this stuff if they don’t trust it.” — Simon
“I’m always asking what’s the user experience going to be? How are we actually going to put something in front of people that they’re going to understand…” — Simon
“There are lots of success stories, and there are lots of failure stories. And that’s just what you expect when you’ve got edtech companies moving at high speed.” — Simon
“We’re dealing, on the one hand, with poor products that give the whole field a bad name, but on the other hand, there are some really great products out there that are making a tangible difference, and teachers are extremely enthusiastic about.” — Simon
“There’s good evidence now, about the impact that some of these tools can have on learning. Teachers can give some homework out, and the next morning, they can see on their dashboard which questions were the students really struggling with.” — Simon
“The area that we’re getting more and more interested in, and which educators are getting more and more interested in, are the kinds of skills and competencies you need for a very complex future workplace.” — Simon
“We obviously want the students’ voice in the design process. But that has to be balanced with all the other voices are there as well, like the educators’ voice, as well as the technologists, and the interaction designers and so forth.” — Simon on the nuance of UX considerations for students
“…you have to balance satisfying the stakeholder with actually what is needed.” — Brian
“…we’re really at the mercy of behavior. We have to try and infer, from behavior or traces, what’s going on in the mind, of the humans we are studying.” — Simon
“We might say, “Well, if we see a student writing like this, using these kinds of textual features that we can pick up using natural language processing, and they revise their draft writing in response to feedback that we’ve provided automatically, well, that looks like progress. It looks like they’re thinking more critically, or it looks like they’re reflecting more deeply on an experience they’ve had, for example, like a work placement.” — Simon
“They’re in products already, and when they’re used well, they can be effective. But they can also be sort of weapon of mass destruction if you use them badly.” — Simon, on predictive models
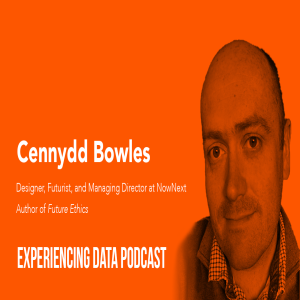
Tuesday Mar 24, 2020
Tuesday Mar 24, 2020
Cennydd Bowles is a London-based digital product designer and futurist, with almost two decades of consulting experience working with some of the largest and most influential brands in the world. Cennydd has earned a reputation as a trusted guide, helping companies navigate complex issues related to design, technology, and ethics. He’s also the author of Future Ethics, a book which outlines key ethical principles and methods for constructing a fairer future.
In this episode, Cennydd and I explore the role that ethics plays in design and innovation, and why so many companies today—in Silicon Valley and beyond—are failing to recognize the human element of their technological pursuits. Cennydd offers his unique perspective, along with some practical tips that technologists can use to design with greater mindfulness and consideration for others.
In our chat, we covered topics from Cennydd’s book and expertise including:
- Why there is growing resentment towards the tech industry and the reason all companies and innovators need to pay attention to ethics
- The importance of framing so that teams look beyond the creation of an “ethical product / solution” and out towards a better society and future
- The role that diversity plays in ethics and the reason why homogenous teams working in isolation can be dangerous for an organization and society
- Cennydd’s “front-page test,” “designated dissenter,” and other actionable ethics tips that innovators and data product teams can apply starting today
- Navigating the gray areas of ethics and how large companies handle them
- The unfortunate consequences that arise when data product teams are complacent
- The fallacy that data is neutral—and why there is no such thing as “raw” data
- Why stakeholders must take part in ethics conversations
Resources and Links:
Future Ethics (book)
Quotes from Today’s Episode
“There ought to be a clearer relationship between innovation and its social impacts.” — Cennydd
“I wouldn’t be doing this if I didn’t think there was a strong upside to technology, or if I didn’t think it couldn’t advance the species.” — Cennydd
“I think as our power has grown, we have failed to use that power responsibly, and so it’s absolutely fair that we be held to account for those mistakes.” — Cennydd
“I like to assume most creators and data people are trying to do good work. They’re not trying to do ethically wrong things. They just lack the experience or tools and methods to design with intent.” — Brian
“Ethics is about discussion and it’s about decisions; it’s not about abstract theory.” — Cennydd
“I have seen many times diversity act as an ethical early warning system [where] people who firmly believe the solution they’re about to put out into the world is, if not flawless, pretty damn close.” — Cennydd
“The ethical questions around the misapplication or the abuse of data are strong and prominent, and actually have achieved maybe even more recognition than other forms of harm that I talk about.” — Cennydd
“There aren’t a whole lot of ethical issues that are black and white.” — Cennydd
“When you never talk to a customer or user, it’s really easy to make choices that can screw them at the benefit of increasing some KPI or business metric.” — Brian
“I think there’s really talented people in the data space who actually understand bias really well, but when they think about bias, they think they’re thinking more about, ‘how is it going to skew the insight from the data?’ Not the human impact.” — Brian
“I think every business has almost a moral duty to take their consequences seriously.” — Cennydd